-CLVAE: a semantic disentangled generative model
MULTIMEDIA TOOLS AND APPLICATIONS(2024)
摘要
Learning disentangled representations from data that reveal inherent semantic information is crucial for the interpretability of generative models such as Variational Auto-Encoders (VAEs) and Generative Adversarial Networks (GANs). However, finding a balance between disentanglement and generation is challenging. The traditional disentangled model, beta-VAE, often fails to reconstruct clear images. To address this issue, we propose a novel contrastive learning method called beta-CLVAE that enhances the generation ability and improves the disentanglement of beta-VAE. In our method, we use a Recurrent Neural Network (RNN) to generate robust anchor samples that are fed, along with the reconstructions from beta-VAE, to a contrastive learning framework. We also introduce a pixel-level Gram matrix that imposes constraints on the input and output images to improve the quality of the decoder's reconstruction. By using contrastive learning, beta-CLVAE can learn valuable semantic information that benefits disentangled and generative representations. Our experiments demonstrate the advantages of our model in terms of qualitative comparison of disentangled features, quantitative comparison of disentanglement scores, and the accuracy of downstream classification tasks. Overall, beta-CLVAE is an excellent semantic disentangled representation model that is beneficial for downstream tasks.
更多查看译文
关键词
Variational auto-encoder,Contrastive learning,Gram matrix,Disentangled representation
AI 理解论文
溯源树
样例
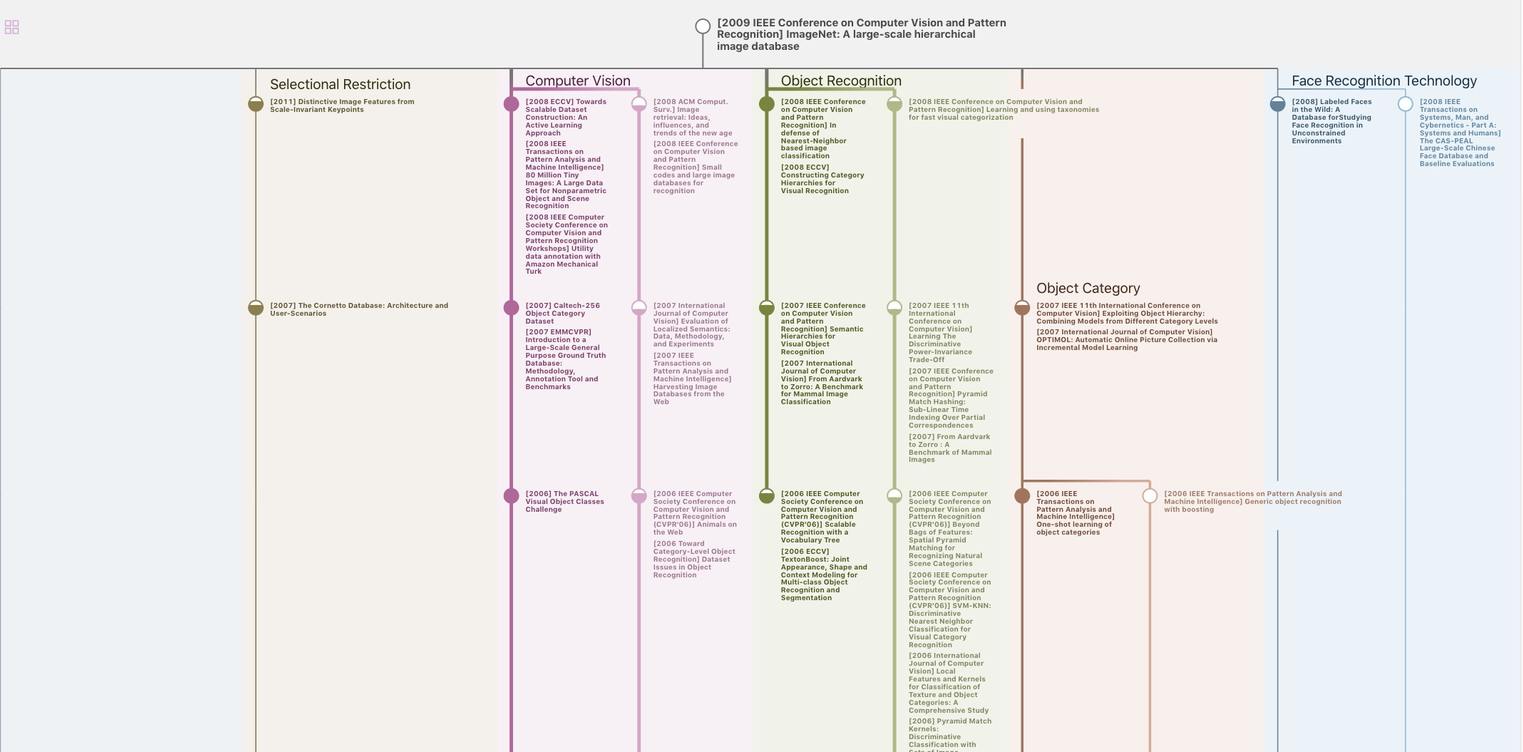
生成溯源树,研究论文发展脉络
Chat Paper
正在生成论文摘要