Autoencoders with Deformable Convolutions for latent representation of EEG Spectrograms in classification tasks
FIFTEENTH INTERNATIONAL CONFERENCE ON MACHINE VISION, ICMV 2022(2023)
摘要
Electroencephalogram (EEG) is a set of time series each of which can be represented as a 2D image (spectrogram), so that EEG recording can be mapped to the C-dimensional image (where C denotes the number of channels in the image and equals to the number of electrodes in EEG montage). In this paper, a novel approach for automated feature extraction from spectrogram representation is proposed. The method involves the usage of autoencoder models based on 3-dimensional convolution layers and 2-dimensional deformable convolution layers. Features, extracted by autoencoders, can be used to classify patients with Major Depressive Disorder (MDD) from healthy controls based on resting-state EEG. The proposed approach outperforms baseline ML models trained on spectral features extracted manually. The source code is available on: source code
更多查看译文
关键词
2D/3D autoencoders, deformable convolutions, deep learning for psychiatry, EEG spectral features, EEG for major depressive disorder
AI 理解论文
溯源树
样例
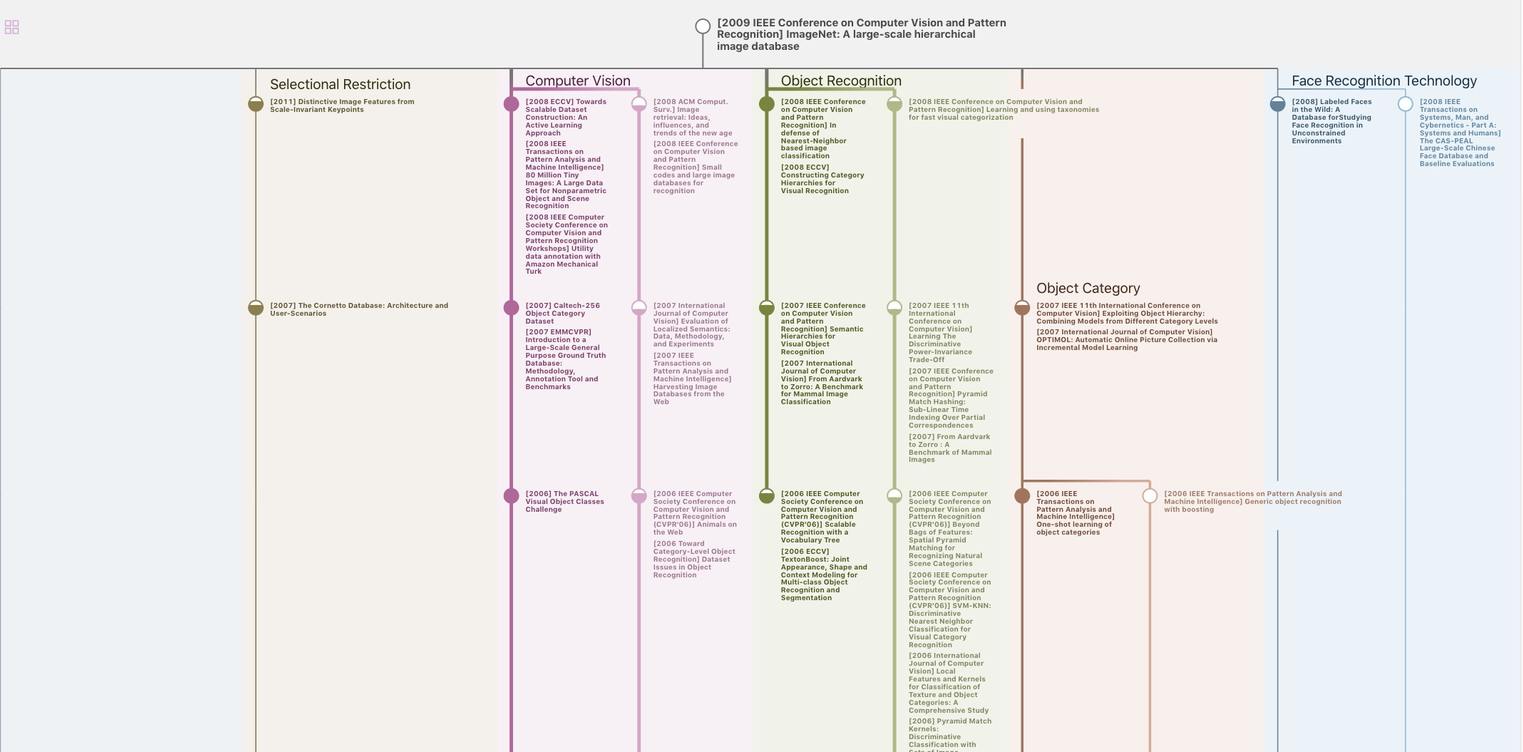
生成溯源树,研究论文发展脉络
Chat Paper
正在生成论文摘要