Supervised biadjacency networks for stereo matching
Multimedia Tools and Applications(2024)
摘要
Convolutional neural network (CNN) based stereo matching methods using cost volume techniques have gained prominence in stereo matching. State-of-the-art cost volume based methods use two weight-sharing feature extractors to respectively extract left and right unary features and then use them to construct cost volume(s). The quality of those unary features is crucial for the subsequent stereo matching. We propose a Supervised Biadjacency-based (SuperB) module to improve their quality by employing supervised biadjacency matrices to embed stereo information into both unary features. Specifically, disparity supervision is imposed on the biadjacency matrices by transforming them into disparity estimations. The SuperB Module can therefore adaptively enhance matched features and suppress unmatched features. Being aware of the stereo correspondence, the resultant stereo-aware features are more discriminative for subsequent cost aggregation and disparity estimation. Experiments show the SuperB Module can be plugged into cost volume based stereo matching models and lower the disparity estimation error. In addition, a scale-adaptive Voxel-wise Selective Fusion (VSF) module is proposed to adaptively aggregate the multi-scale matching costs. The competitive and efficient experimental results on both synthetic and real-world datasets demonstrate the effectiveness of the resultant Supervised Biadjacency Stereo matching networks (SuperBStereo).
更多查看译文
关键词
Stereo matching,Convolutional neural networks,Bipartite graph,Supervision,Disparity
AI 理解论文
溯源树
样例
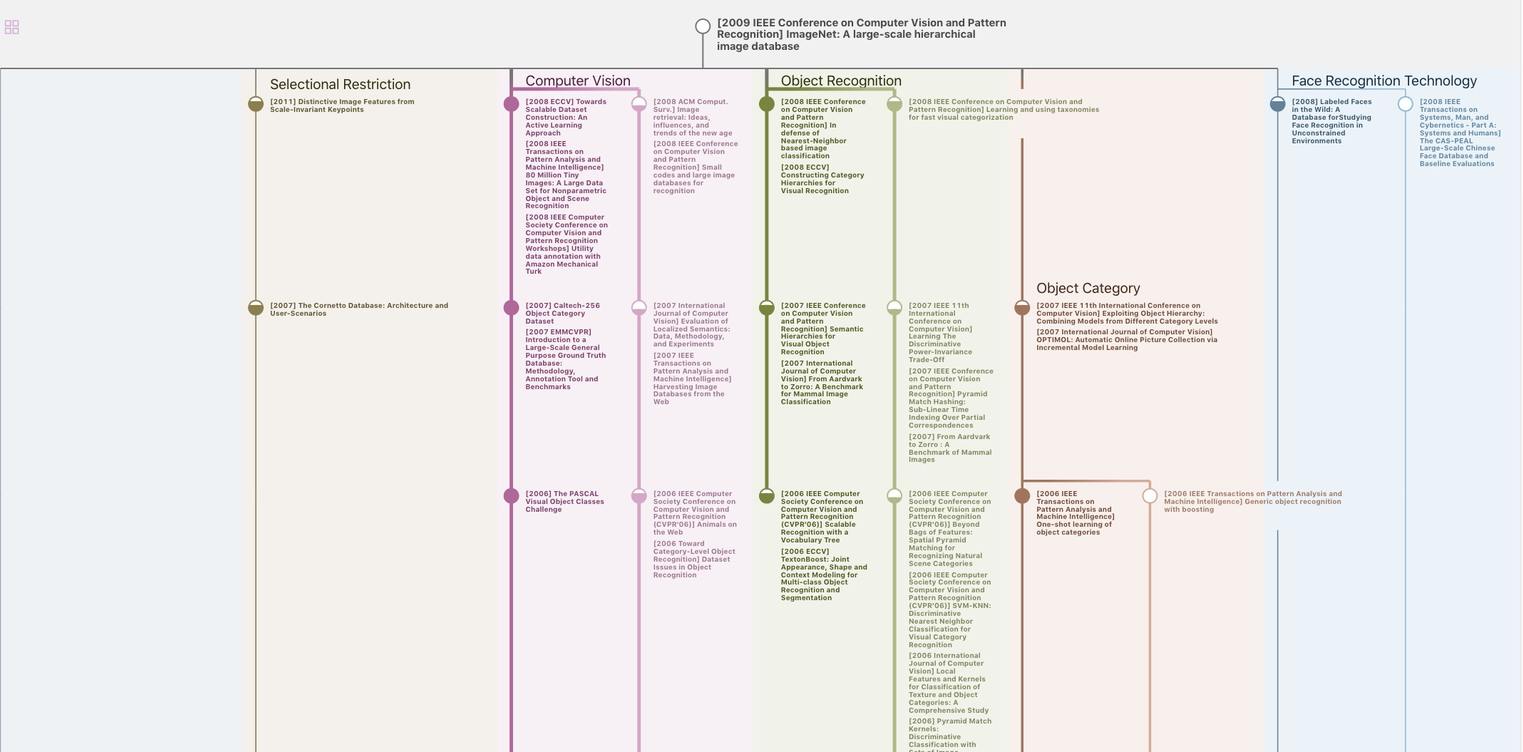
生成溯源树,研究论文发展脉络
Chat Paper
正在生成论文摘要