RSARE: A physically-based vegetation index for estimating wheat green LAI to mitigate the impact of leaf chlorophyll content and residue-soil background
ISPRS JOURNAL OF PHOTOGRAMMETRY AND REMOTE SENSING(2023)
摘要
The green leaf area index (LAI) is an important structural parameter that can be used for monitoring plant transpiration and carbon cycling over large spatial extents. LAI is also critical for understanding biophysical processes and predicting crop productivity. However, the retrieval of wheat green LAI based on canopy reflec-tance is always affected by the background material. For example, in the Yangtze River Basin of China, rice residues are typically returned to rice-wheat rotation fields. Thus the background for observing wheat canopy varies from a mixture of traditional wheat-soil components to wheat-residue or wheat-residue-soil, posing a significant challenge for accurate LAI estimation at the early stages of crop growth. Furthermore, when current vegetation indices (VIs) are used to estimate LAI, leaf chlorophyll content (LCC) and field moisture are two additional confounding factors. To resolve these issues, we propose a novel residue-soil adjusted red edge dif-ference index (RSARE), which draws on concepts from a simple physical algorithm, i.e., linear spectral mixture analysis (LSMA). The results of field, satellite and modeling experiments demonstrate that the RSARE-LAI model is generally not sensitive to LCC, or to variations in residue-soil and traditional soil backgrounds, and estimates LAI with high accuracy (RMSEval = 0.55, RRMSEval=20.71%). In comparison to traditional VI-LAI maps, RASRE-LAI maps are less sensitive to confounding factors associated with the background components, field moisture, LCC, and both inter-annual and geographical differences. This novel residue-soil background-resistant RSARE particularly improves the accuracy of wheat LAI estimation at low LAI levels, thus facilitating large-scale mapping of LAI in the early growing season.
更多查看译文
关键词
vegetation index,leaf chlorophyll content,green lai,physically-based,residue-soil
AI 理解论文
溯源树
样例
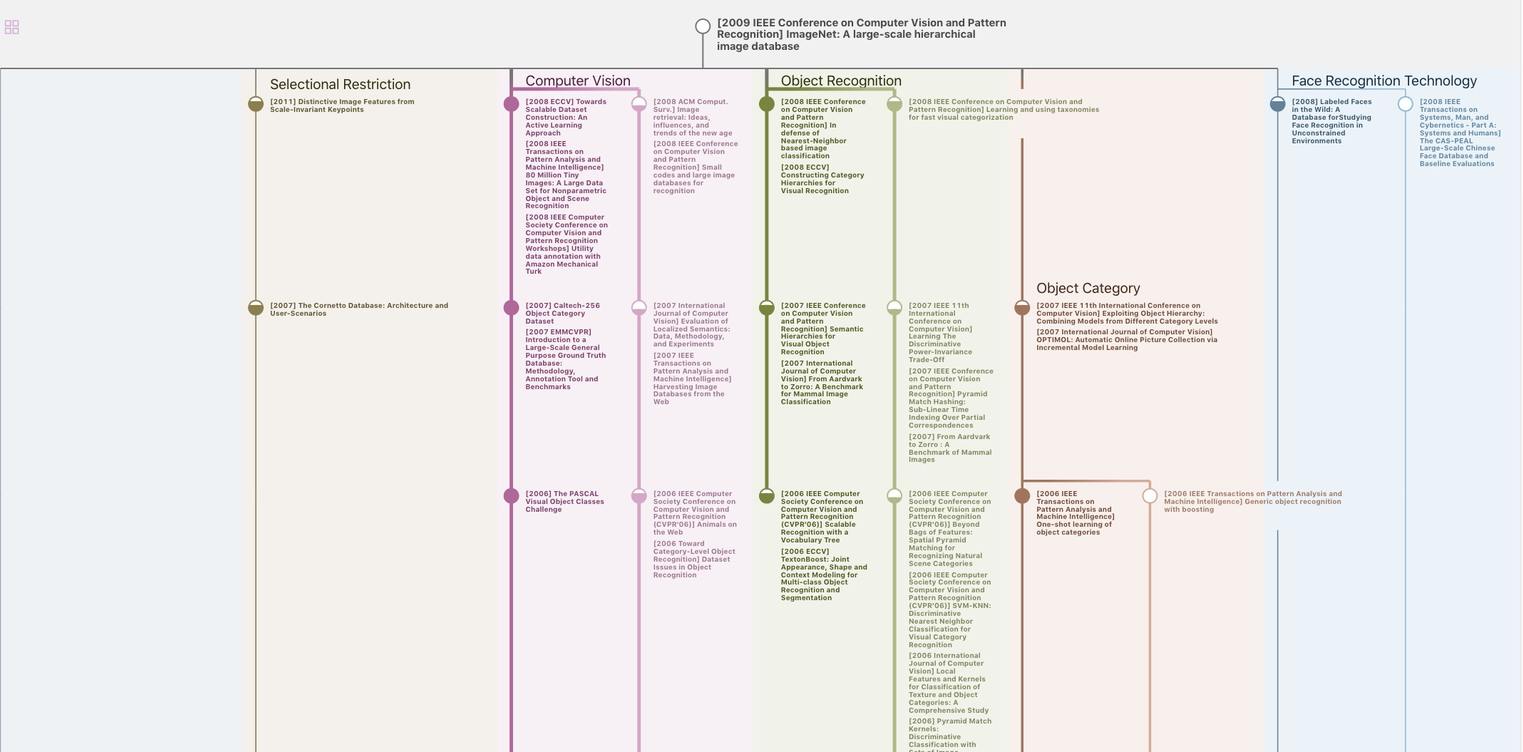
生成溯源树,研究论文发展脉络
Chat Paper
正在生成论文摘要