Self-Supervised Pretraining Improves Performance and Inference Efficiency in Multiple Lung Ultrasound Interpretation Tasks
IEEE ACCESS(2023)
摘要
In this study, we investigated whether self-supervised pretraining could produce a neural network feature extractor applicable to multiple classification tasks in B-mode lung ultrasound analysis. When fine-tuning on three lung ultrasound tasks, pretrained models resulted in an improvement of the average across-task area under the receiver operating characteristic curve (AUC) by 0.032 and 0.061 on local and external test sets respectively. Compact nonlinear classifiers trained on features outputted by a single pretrained model did not improve performance across all tasks; however, they reduced inference time by 49% compared to the serial execution of separate fine-tuned models. When training using 1% of the available labels, pretrained models consistently outperformed fully supervised models, with a maximum observed test AUC increase of 0.396 for the task of view classification. Overall, the results indicate that self-supervised pretraining is a useful strategy for producing initial weights for lung ultrasound classifiers.
更多查看译文
关键词
Task analysis,Feature extraction,Ultrasonic imaging,Lungs,Multitasking,Videos,Training,Self-supervised learning,Multi-task,self-supervised learning,ultrasound
AI 理解论文
溯源树
样例
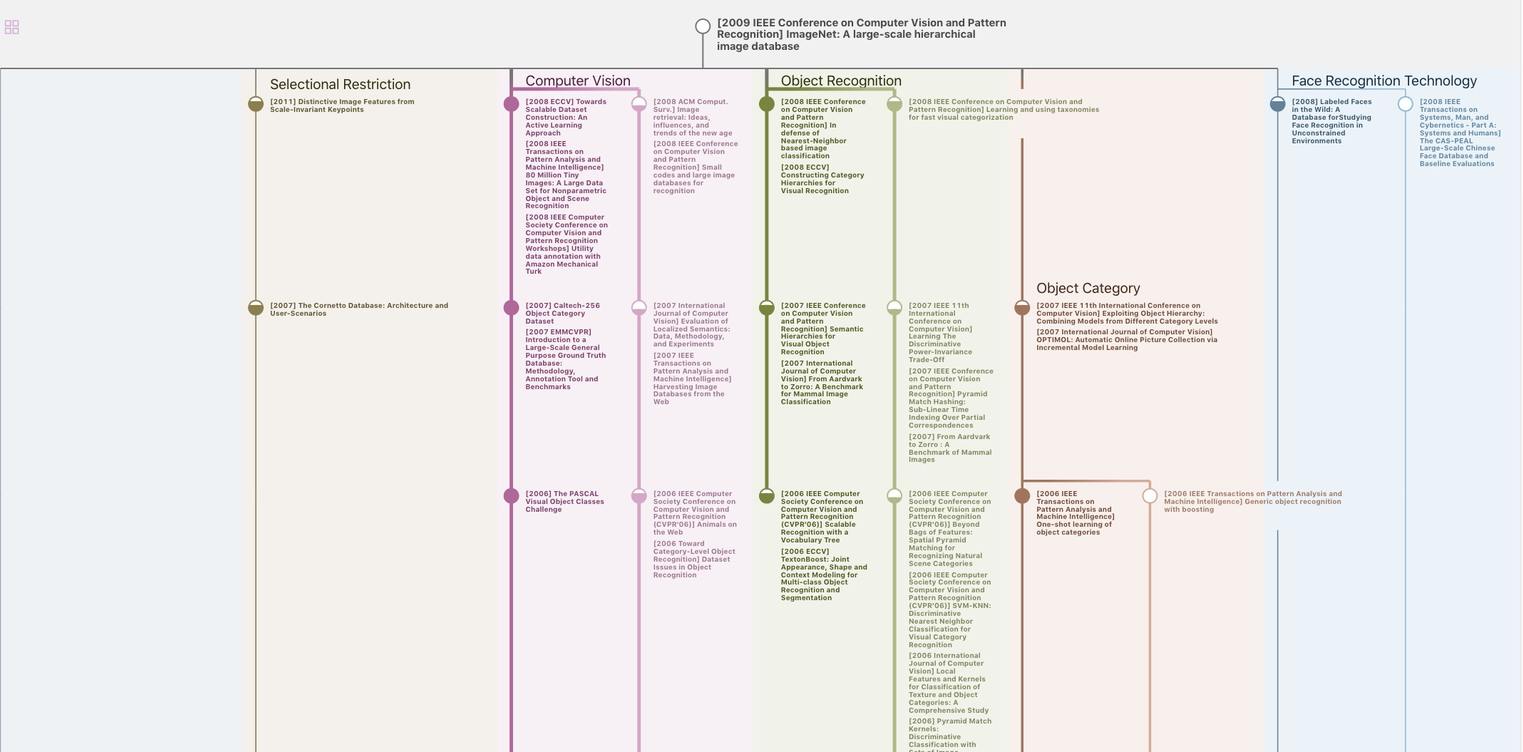
生成溯源树,研究论文发展脉络
Chat Paper
正在生成论文摘要