Data-Driven Real-Time Denied Boarding Prediction in Urban Railway Systems
TRANSPORTATION RESEARCH RECORD(2024)
摘要
Providing real-time crowding information in urban railways would enable informed travel decisions and encourage cooperative behavior of passengers, as well as improve operating efficiency and safety. However, the problem of real-time crowding prediction is not trivial because of the unavailability of ground-truth crowding data, particularly for the direct impact of crowding on passengers (e.g., denied boarding on platforms). This paper proposes a data-driven method for real-time denied boarding prediction in urban railway systems using automated fare collection (AFC) and automated vehicle location (AVL) data. It predicts the denied boarding probability distribution or its derived metrics as a function of explanatory variables, including demand, operations, and incident-related factors. The method is validated through a case study covering 18 months on Hong Kong Mass Transit Railways. The results highlight the model's accurate and robust performance in predicting denied boarding on platforms using purely AFC and AVL data (e.g., an average of 6%-7% error) under both recurrent and non-recurrent situations, in which transfer demand-related factors contribute most to the prediction. The prediction model outputs can support proactive operations control and management, as well as provision of customer information without relying on expensive and time-consuming data collection efforts. Customer information, for example, can include the average number of trains to wait before boarding or the average waiting time on the platform.
更多查看译文
关键词
rail transit systems,crowding prediction,denied boarding probability,big data,smart card data
AI 理解论文
溯源树
样例
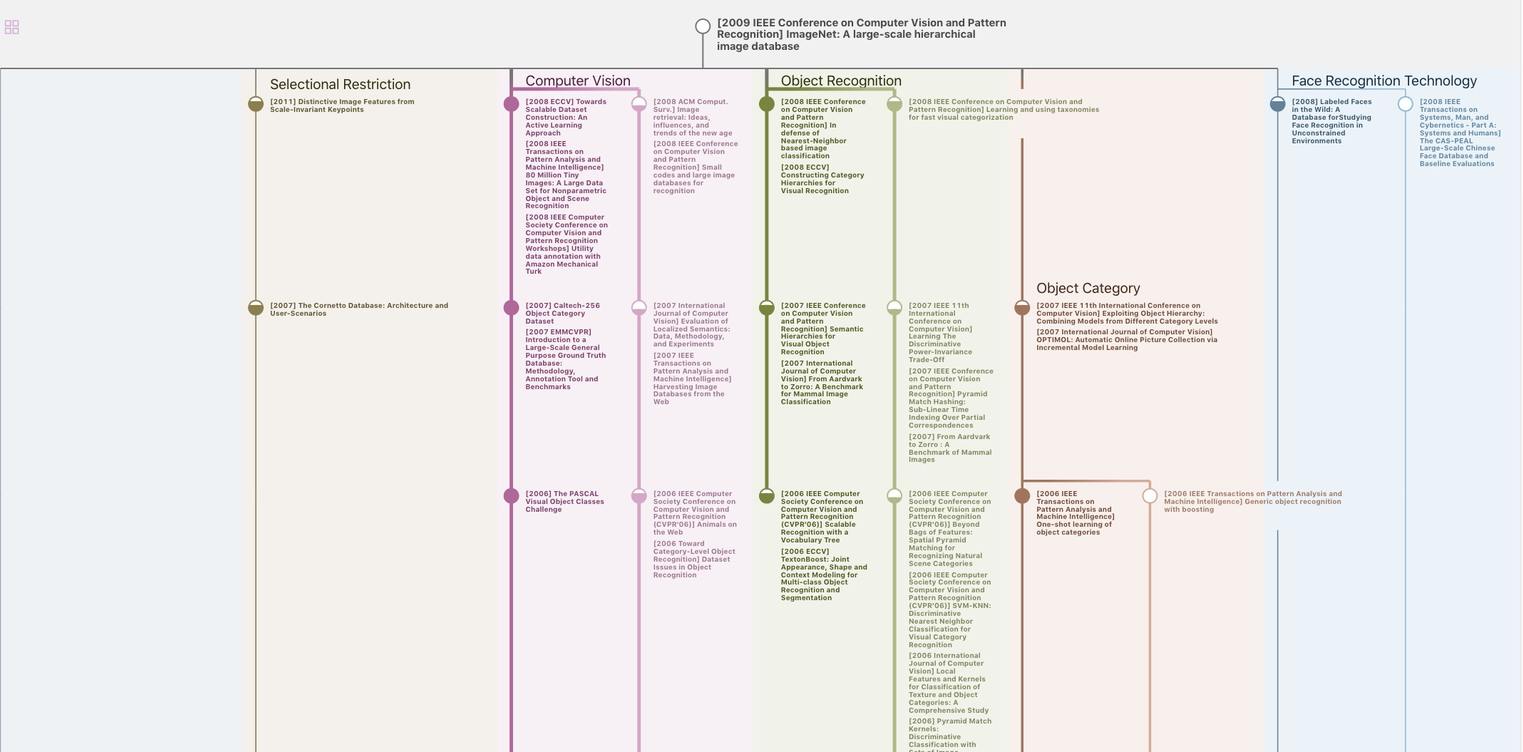
生成溯源树,研究论文发展脉络
Chat Paper
正在生成论文摘要