Coordinated Variable Speed Limit Control for Consecutive Bottlenecks on Freeways Using Multiagent Reinforcement Learning
JOURNAL OF ADVANCED TRANSPORTATION(2023)
摘要
Most of the current variable speed limit (VSL) strategies are designed to alleviate congestion in relatively short freeway segments with a single bottleneck. However, in reality, consecutive bottlenecks can occur simultaneously due to the merging flow from multiple ramps. In such situations, the existing strategies use multiple VSL controllers that operate independently, without considering the traffic flow interactions and speed limit differences. In this research, we introduced a multiagent reinforcement learning-based VSL (MARL-VSL) approach to enhance collaboration among VSL controllers. The MARL-VSL approach employed a centralized training with decentralized execution structure to achieve a joint optimal solution for a series of VSL controllers. The consecutive bottleneck scenarios were simulated in the modified cell transmission model to validate the effectiveness of the proposed strategy. An independent single-agent reinforcement learning-based VSL (ISARL-VSL) and a feedback-based VSL (feedback-VSL) were also applied for comparison. Time-varying heterogeneous traffic flow stemming from the mainline and ramps was loaded into the freeway network. The results demonstrated that the proposed MARL-VSL achieved superior performance compared to the baseline methods. The proposed approach reduced the total time spent by the vehicles by 18.01% and 17.07% in static and dynamic traffic scenarios, respectively. The control actions of the MARL-VSL were more appropriate in maintaining a smooth freeway traffic flow due to its superior collaboration performance. More specifically, the MARL-VSL significantly improved the average driving speed and speed homogeneity across the entire freeway.
更多查看译文
关键词
freeways,consecutive bottlenecks,speed
AI 理解论文
溯源树
样例
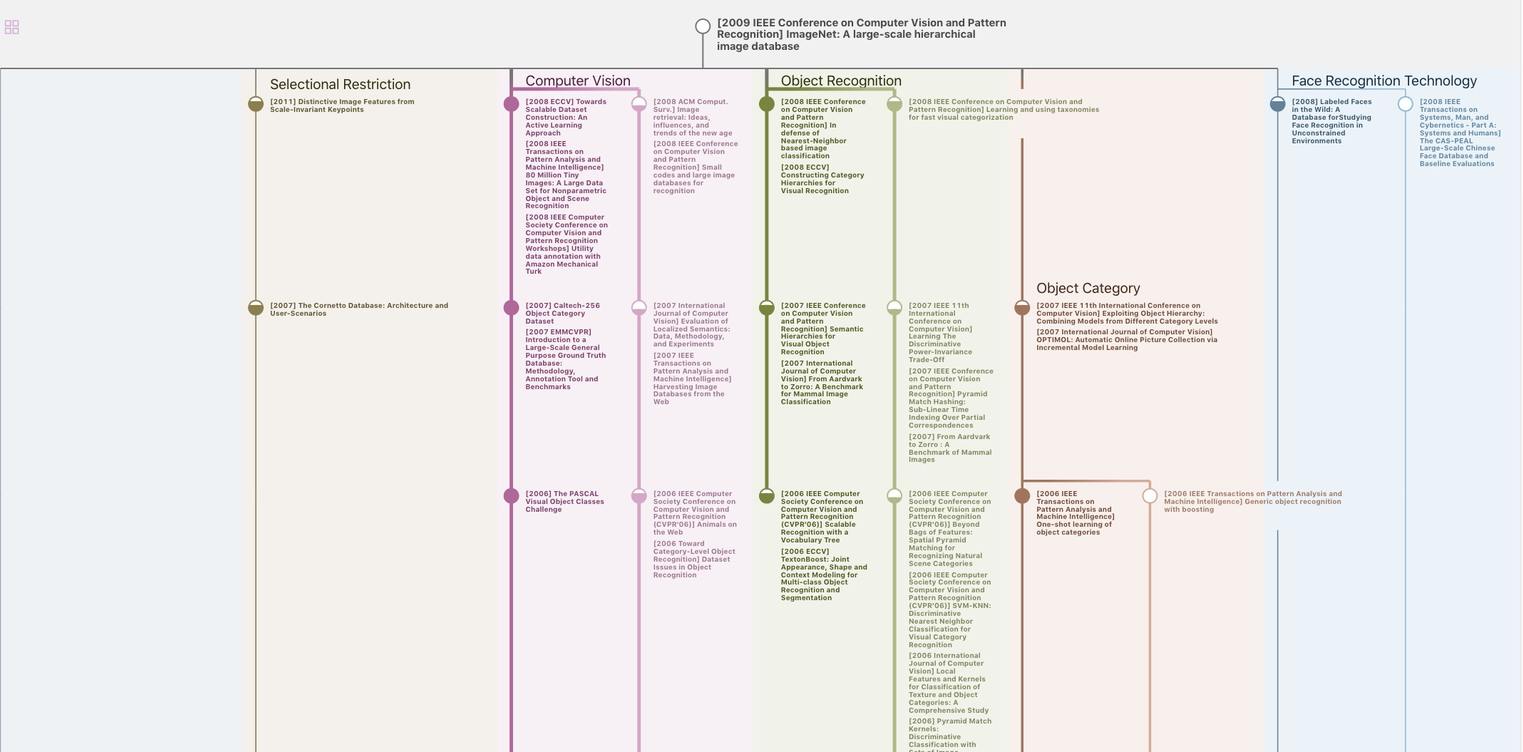
生成溯源树,研究论文发展脉络
Chat Paper
正在生成论文摘要