Cross-domain state-of-health estimation of Li-ion batteries based on transfer neural network with soft-dynamic time warping
ENERGY SCIENCE & ENGINEERING(2023)
摘要
The success of deep learning in the field of state-of-health (SOH) estimation relies on a large amount of battery data and the fact that all data possess the same probability distribution. While in real situations, a model based on one working condition data set may not be valid for another working condition data set due to distribution differences. Therefore, this article proposes a transfer learning method using soft-dynamic time warping (soft-DTW) as the statistical feature in the feature transfer method, called soft-DTW domain adaptation network (SDDAN). By combining the prediction error with the time-series gap in the model training process, the feature transformation can make the obtained prediction results more similar to the source domain results, which can help us to obtain better prediction results in the target domain. Experimental results show that SDDAN can effectively predict the SOH of Li-ion batteries and significantly improve the performance of feature learning and knowledge transfer.
更多查看译文
关键词
dynamic time warping (DTW),state‐of‐health (SOH),transfer learning
AI 理解论文
溯源树
样例
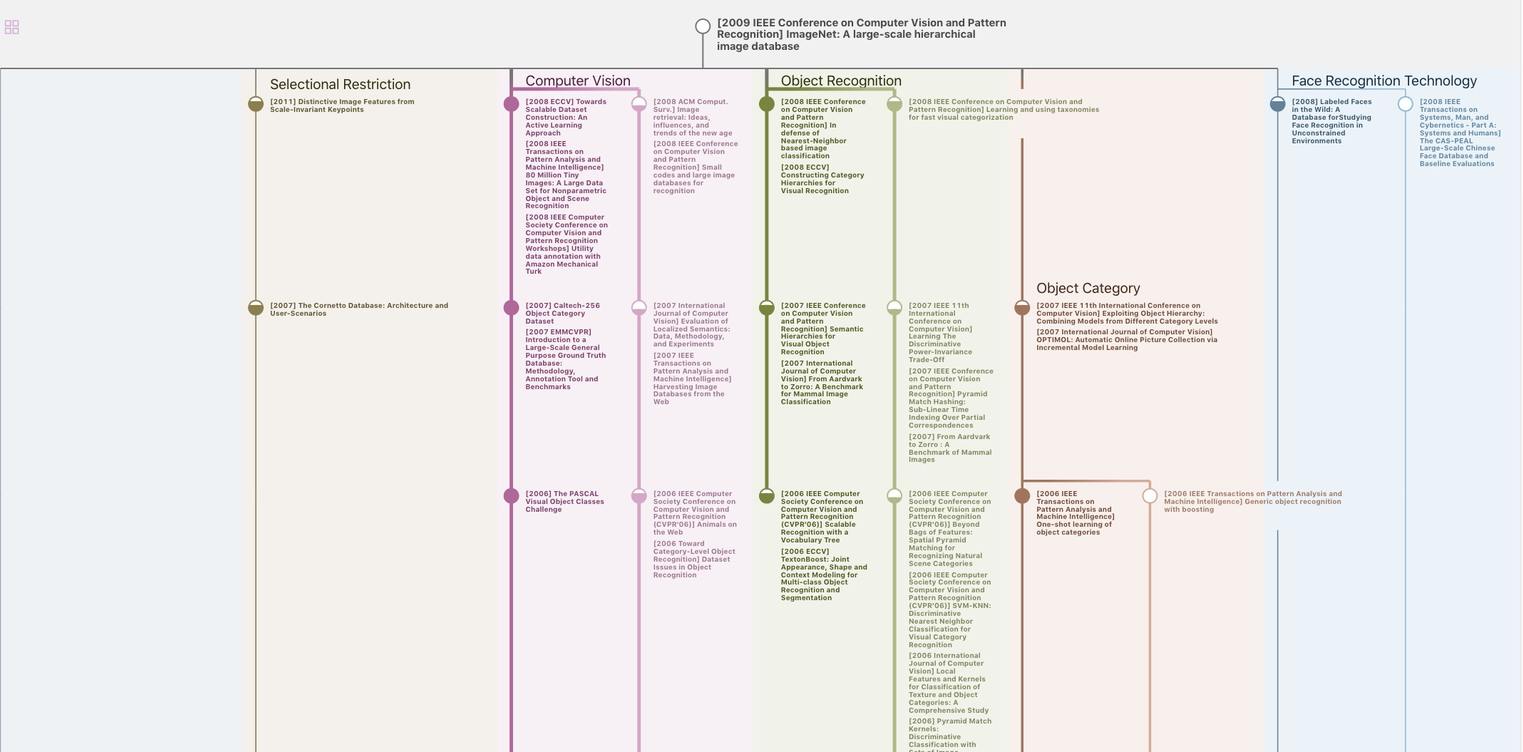
生成溯源树,研究论文发展脉络
Chat Paper
正在生成论文摘要