Collaborative Wideband Spectrum Sensing and Scheduling for Networked UAVs in UTM Systems
IEEE CONFERENCE ON GLOBAL COMMUNICATIONS, GLOBECOM(2023)
摘要
In this paper, we propose a data-driven framework for collaborative wideband spectrum sensing and scheduling for networked unmanned aerial vehicles (UAVs), which act as the secondary users to opportunistically utilize detected spectrum holes. To this end, we propose a multi-class classification problem for wideband spectrum sensing to detect vacant spectrum spots based on collected I/Q samples. To enhance the accuracy of the spectrum sensing module, the outputs from the multi-class classification by each individual UAV are fused at a server in the unmanned aircraft system traffic management (UTM) ecosystem. In the spectrum scheduling phase, we leverage reinforcement learning (RL) solutions to dynamically allocate the detected spectrum holes to the secondary users (i.e., UAVs). To evaluate the proposed methods, we establish a comprehensive simulation framework that generates a near-realistic synthetic dataset using MATLAB LTE toolbox by incorporating base-station (BS) locations in a chosen area of interest, performing ray-tracing, and emulating the primary users channel usage in terms of I/Q samples. This evaluation methodology provides a flexible framework to generate large spectrum datasets that could be used for developing ML/AI-based spectrum management solutions for aerial devices.
更多查看译文
关键词
Unmanned Aerial Vehicles,Spectrum Sensing,Multi-label,Base Station,Dynamic Allocation,Unlicensed Spectrum,Multi-class Classification Problem,Unmanned Aerial Systems,Secondary Users,Spectra Dataset,Convolutional Neural Network,Performance Metrics,Precision And Recall,Time Slot,Current Step,Markov Decision Process,Target Network,Reinforcement Learning Algorithm,Primary Network,Deep Q-network,Spectrum Access,Double Deep Q-network,Unmanned Aerial Vehicle Position,Fusion Rule,Spectrum Allocation,Multiple Unmanned Aerial Vehicles,Integer Programming Problem,Deep Q-learning,Locations Of Base Stations,Experience Replay
AI 理解论文
溯源树
样例
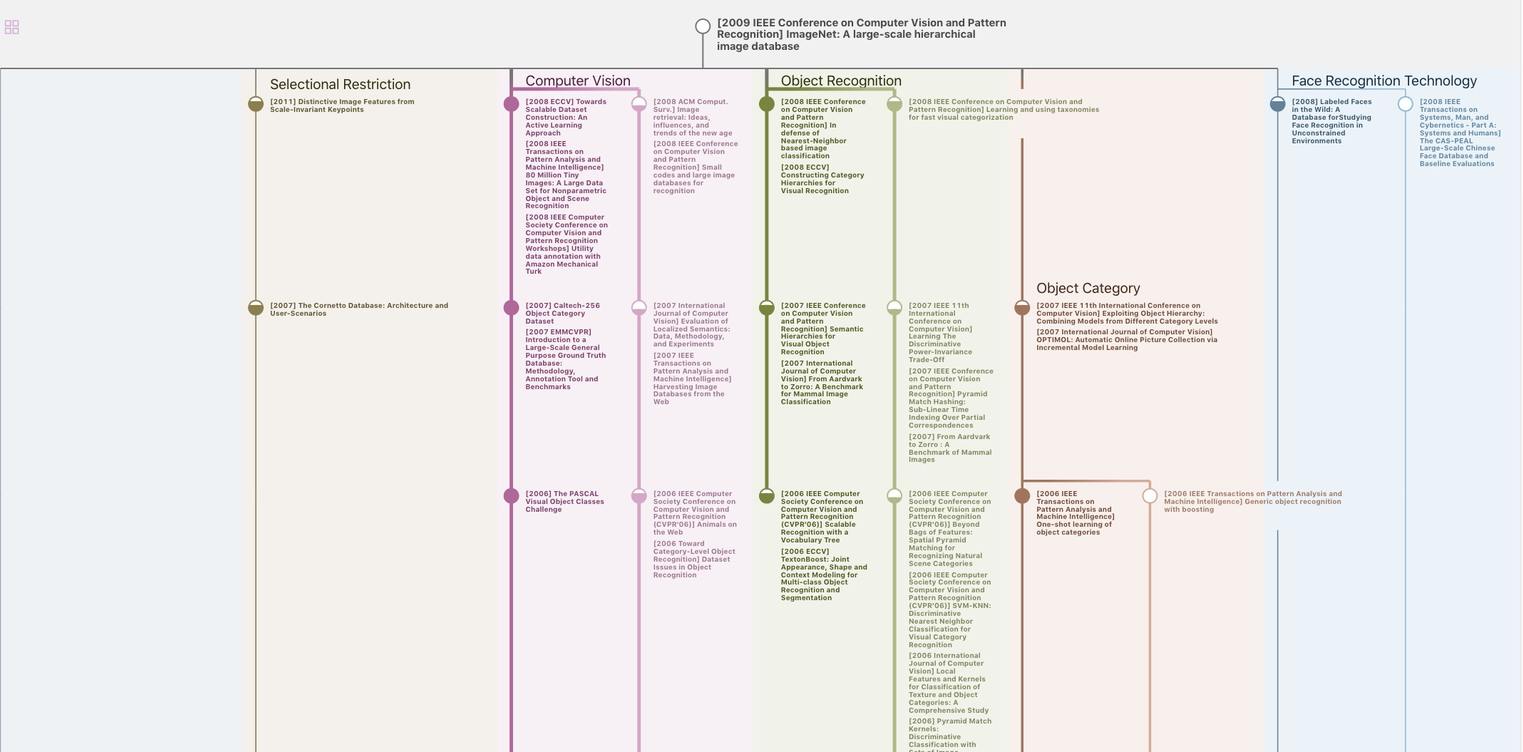
生成溯源树,研究论文发展脉络
Chat Paper
正在生成论文摘要