Tram-FL: Routing-based Model Training for Decentralized Federated Learning
2024 IEEE 21ST CONSUMER COMMUNICATIONS & NETWORKING CONFERENCE, CCNC(2024)
摘要
In decentralized federated learning (DFL), the dual challenges of extensive inter-node communication and nonindependent, identically distributed (non-IID) data impede the attainment of high-accuracy models while maintaining minimal communication traffic. We propose Tram-FL, which progressively refines a global model by transferring it sequentially amongst nodes. We also introduce a dynamic model routing algorithm for optimal route selection, aimed at enhancing model precision with minimal forwarding. Our experiments demonstrate that TramFL with the proposed routing delivers high model accuracy, outperforming baselines while reducing communication costs.
更多查看译文
关键词
Decentralized Federated Learning,Communication Efficiency,Distributed Machine Learning.
AI 理解论文
溯源树
样例
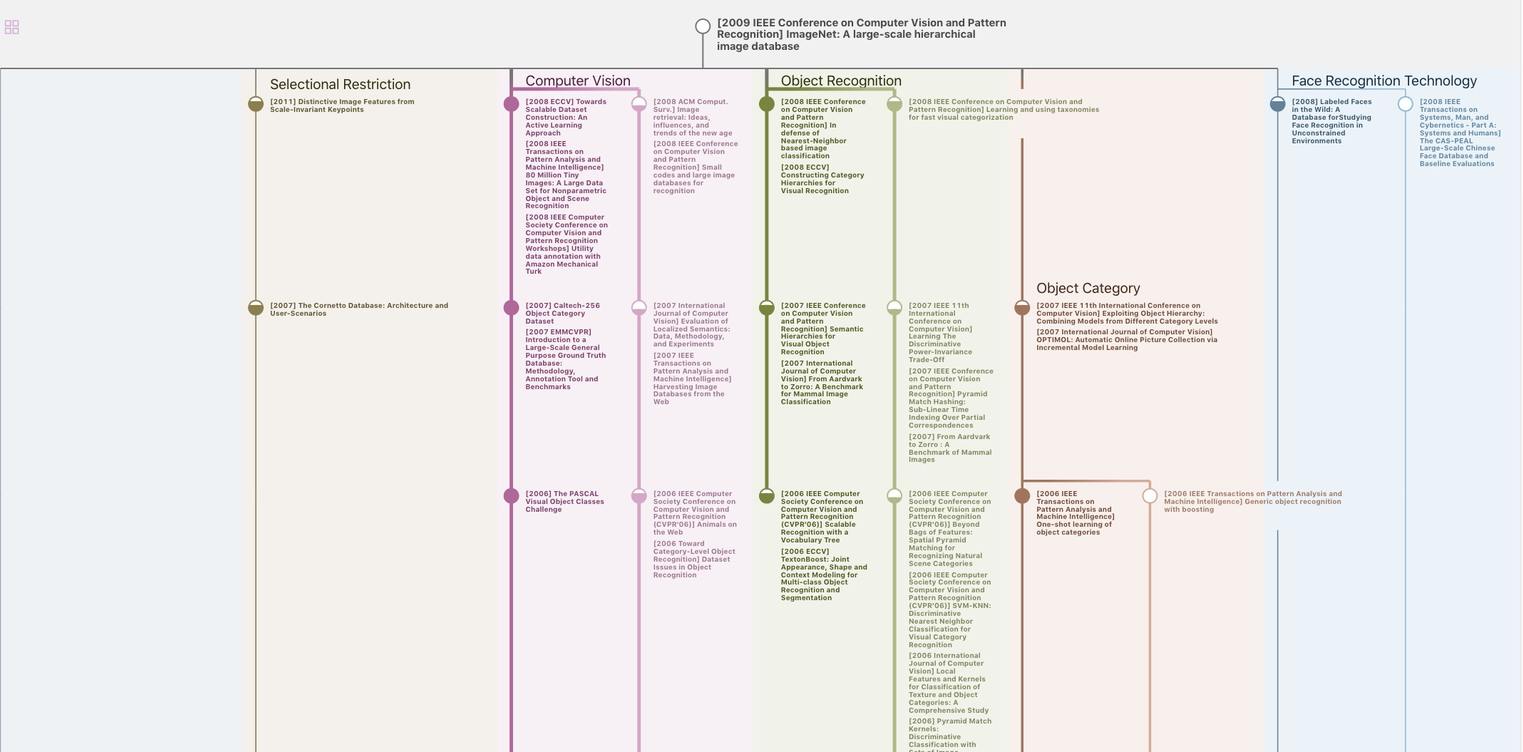
生成溯源树,研究论文发展脉络
Chat Paper
正在生成论文摘要