SSL-Auth: An Authentication Framework by Fragile Watermarking for Pre-trained Encoders in Self-supervised Learning
CoRR(2023)
摘要
Self-supervised learning (SSL) which leverages unlabeled datasets for pre-training powerful encoders has achieved significant success in recent years. These encoders are commonly used as feature extractors for various downstream tasks, requiring substantial data and computing resources for their training process. With the deployment of pre-trained encoders in commercial use, protecting the intellectual property of model owners and ensuring the trustworthiness of the models becomes crucial. Recent research has shown that encoders are threatened by backdoor attacks, adversarial attacks, etc. Therefore, a scheme to verify the integrity of pre-trained encoders is needed to protect users. In this paper, we propose SSL-Auth, the first fragile watermarking scheme for verifying the integrity of encoders without compromising model performance. Our method utilizes selected key samples as watermark information and trains a verification network to reconstruct the watermark information, thereby verifying the integrity of the encoder. By comparing the reconstruction results of the key samples, malicious modifications can be effectively detected, as altered models should not exhibit similar reconstruction performance as the original models. Extensive evaluations on various models and diverse datasets demonstrate the effectiveness and fragility of our proposed SSL-Auth.
更多查看译文
AI 理解论文
溯源树
样例
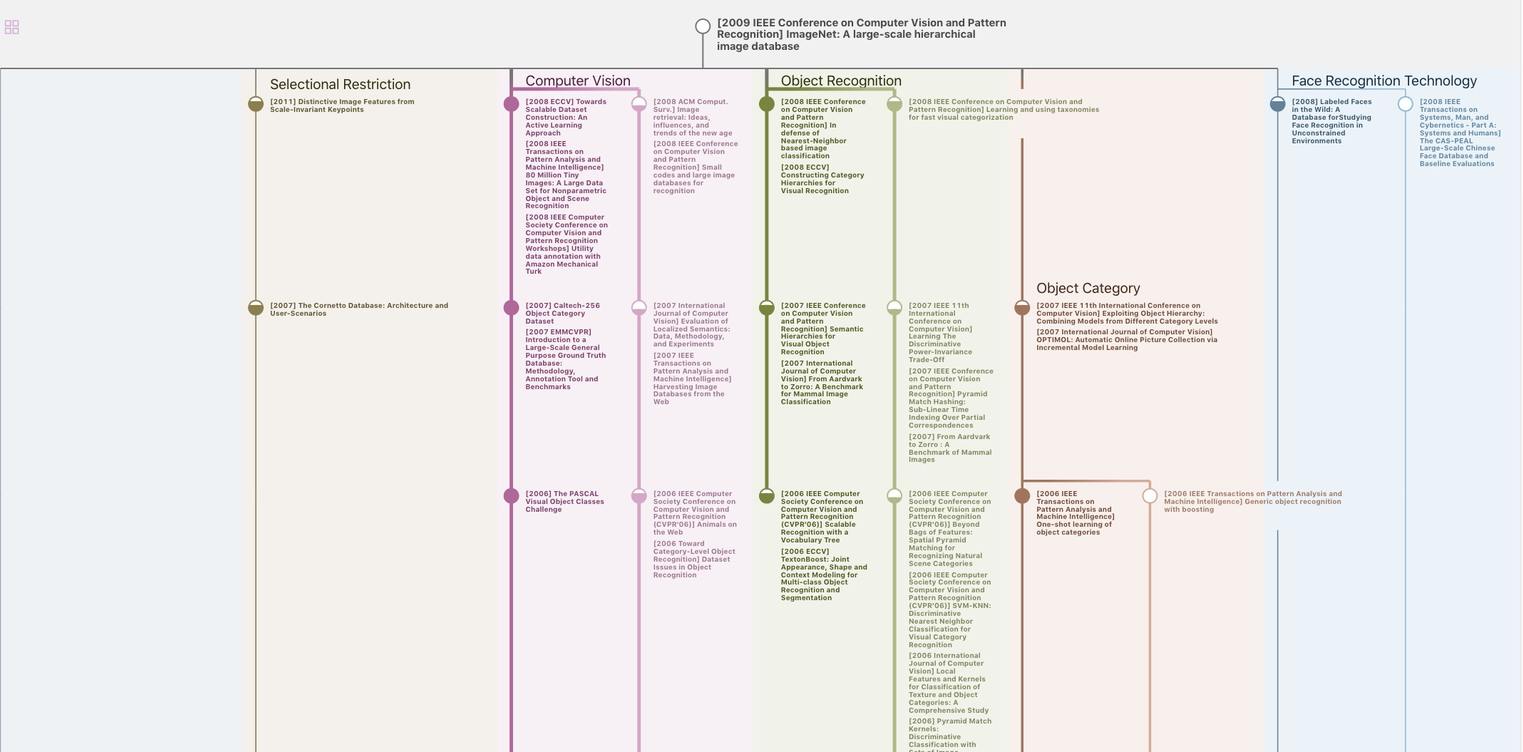
生成溯源树,研究论文发展脉络
Chat Paper
正在生成论文摘要