Inverse Modeling for Subsurface Flow Based on Deep Learning Surrogates and Active Learning Strategies
WATER RESOURCES RESEARCH(2023)
摘要
Inverse modeling is usually necessary for prediction of subsurface flows, which is beneficial to characterize underground geologic properties and reduce prediction uncertainty. Considering the intensive computational effort required for repeated simulation runs when solving inverse problems, surrogate models can be built to substitute for the simulator and improve inversion efficiency. Deep learning models have been widely used for surrogate modeling of subsurface flow. However, a large amount of training data is usually needed to train the models, especially for constructing globally accurate surrogate models, which would bring large computational burden. In fact, the local accuracy of surrogate models in regions around the true solution of the inverse problem and the potential searching path of the solution is more important for the inversion processes. The local accuracy of surrogate models can be enhanced with active learning. In this work, active learning strategies based on likelihood or posterior information are proposed for inverse modeling, including both offline and online learning strategies. In the offline strategy, a pre-trained model is utilized to select samples with higher likelihood, which can produce model responses closer to the observations, and then the selected samples can be used to retrain the surrogate. The retrained surrogate is further integrated with the iterative ensemble smoother (IES) algorithm for inversion. In the online strategy, the pre-trained model is adaptively updated and refined with the selected posterior samples in each iteration of IES to continuously adapt to the solution searching path. Several subsurface flow problems, including both single-phase groundwater flow and two-phase (oil-water) flow problems, are introduced to evaluate the performance of the proposed active learning strategies. The results show that the proposed strategies achieve better inversion performance than the original surrogate-based inversion method without active learning, and the number of required simulation runs can also be reduced.
更多查看译文
关键词
inverse modeling,deep learning,active learning,subsurface flow,surrogate modeling
AI 理解论文
溯源树
样例
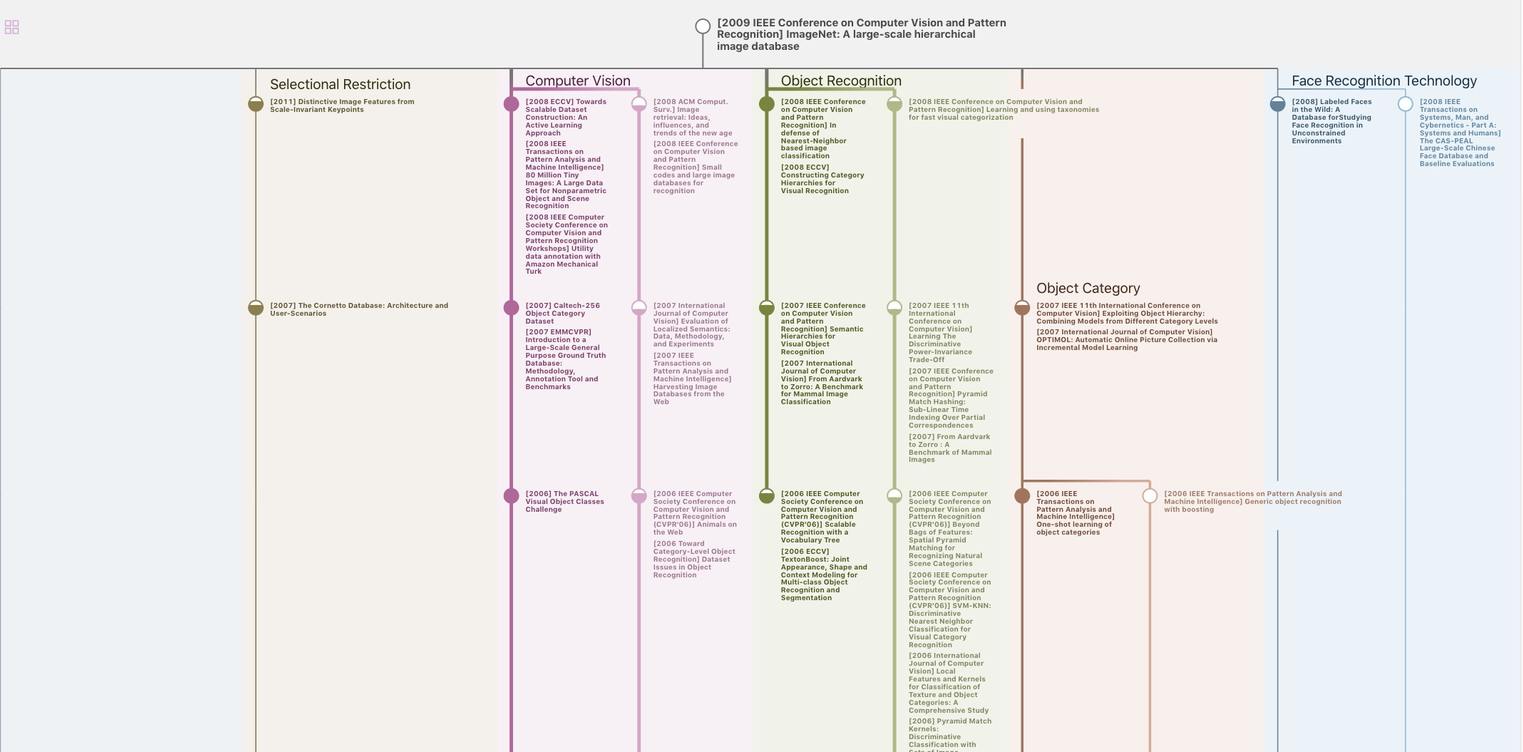
生成溯源树,研究论文发展脉络
Chat Paper
正在生成论文摘要