Depth Estimation from SEM Images using Deep Learning and Angular Data Diversity
METROLOGY, INSPECTION, AND PROCESS CONTROL XXXVII(2023)
摘要
There is a growing need for accurate depth measurements of on-chip structures. Since Scanning Electron Microscopes (SEMs) are already regularly being used for fast and local 2D imaging, it is attractive to explore the 3D capabilities of SEMs. This paper presents a comprehensive study of depth estimation performance when single- or multi-angle data is available. The research starts with an analytical line-scan model to show the major contributors of the signal change with increasing height and angle. We also analyze Monte-Carlo scattering simulations for height sensitivity on similar structures. Next, we validate the depth estimation performance with a supervised machine learning model and show correlation with the previous studies. As predicted by the sensitivity studies, we show that the height prediction greatly improves with increasing tilt angle. Even with a small angle of 3 degrees, a threefold average performance improvement is obtained (RMSE of 16.06 nm to 5.28 nm). Finally, we discuss a preliminary proof-of-concept of a self-supervised algorithm, where no ground-truth data is needed anymore for height retrieval. With this work we show that a data-driven tilted-beam approach is a leap forward in accurate height prediction for the semiconductor industry.
更多查看译文
关键词
CD-SEM images, average height estimation, tilted beam, neural network, simulations, height sensitivity
AI 理解论文
溯源树
样例
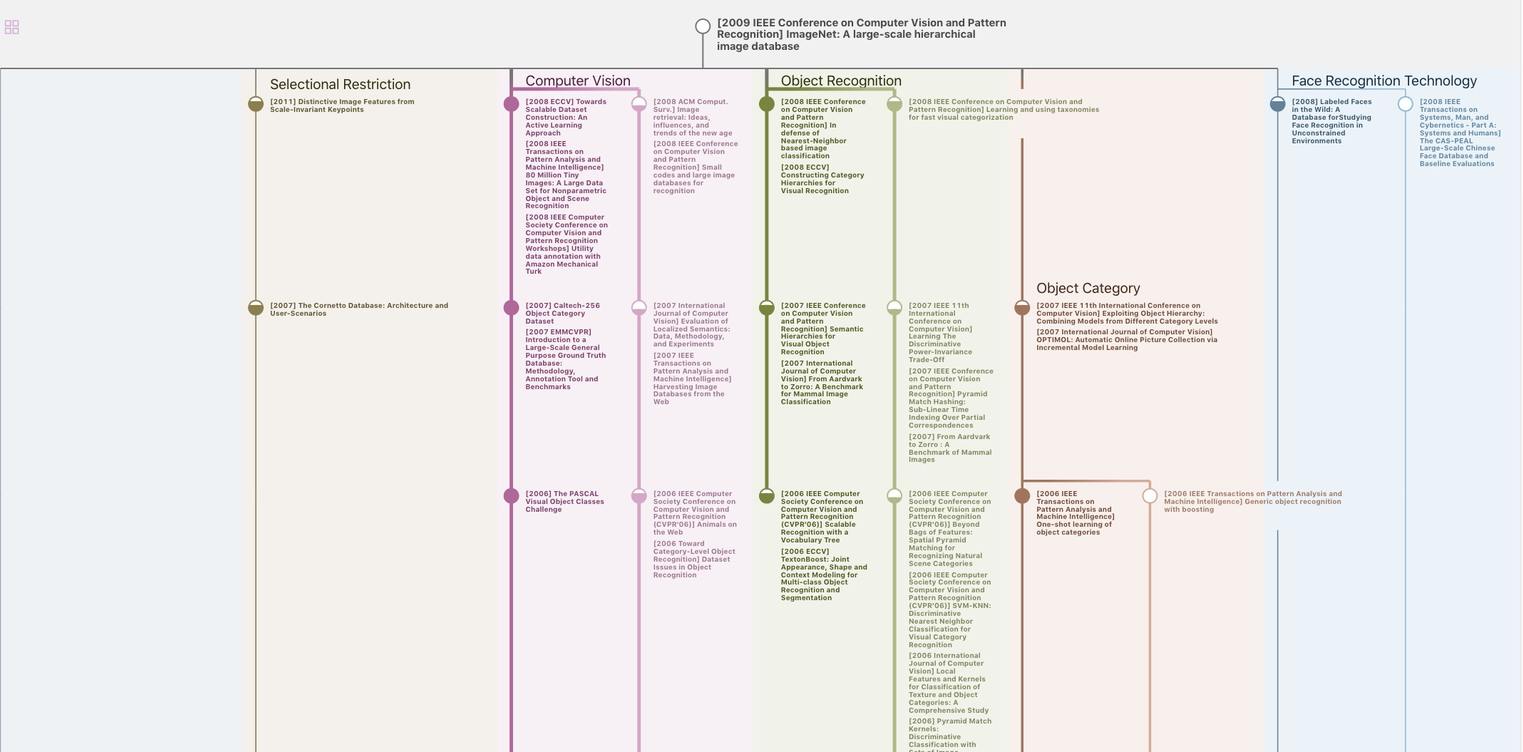
生成溯源树,研究论文发展脉络
Chat Paper
正在生成论文摘要