Catalysing assistive solutions by deploying light-weight deep learning model on edge devices
JOURNAL OF EXPERIMENTAL & THEORETICAL ARTIFICIAL INTELLIGENCE(2023)
摘要
Nowadays, real-time object detection, which is a crucial task, is being performed through image processing and deep learning techniques. As there are several high-performance computing edge devices available, selecting the best-fit device for a particular problem is a tough task and keeping in mind the cost, performance, and weight of the device in mind. One faces several challenges while performing this task in real-time such as a lack of resources in terms of power and mobility. We have provided an insight into the computation power of devices in terms of Frames per Second (FPS) by deploying object detection models on them. This paper will provide insight into selecting the appropriate combination of device and object detection models for real-time applications. Raspberry Pi 3 (RPi3), Raspberry Pi 4 (RPi4), Intel Neural Compute Stick 2 (NCS2), and Nvidia Jetson NANO are popular devices with high computation power used for real-time applications. The memory constraints of devices along with the deployment of different You Only Look Once (YOLO) and Single-Shot Detector (SSD) are the two object detection models that have been explained in this paper. A deep learning inference optimiser, TensorRT, has been used in NANO to achieve high throughput in the performance of object detection. The precision, recall, and F1 score achieved on deploying each tested model have been presented. After observing the devices during experimentation, RPi4+NCS2 showed the best execution with the blend of factors i.e. speed, portability, and user-friendliness.
更多查看译文
关键词
Assistive technology,edge devices,raspberry,neural compute stick,jetson NANO
AI 理解论文
溯源树
样例
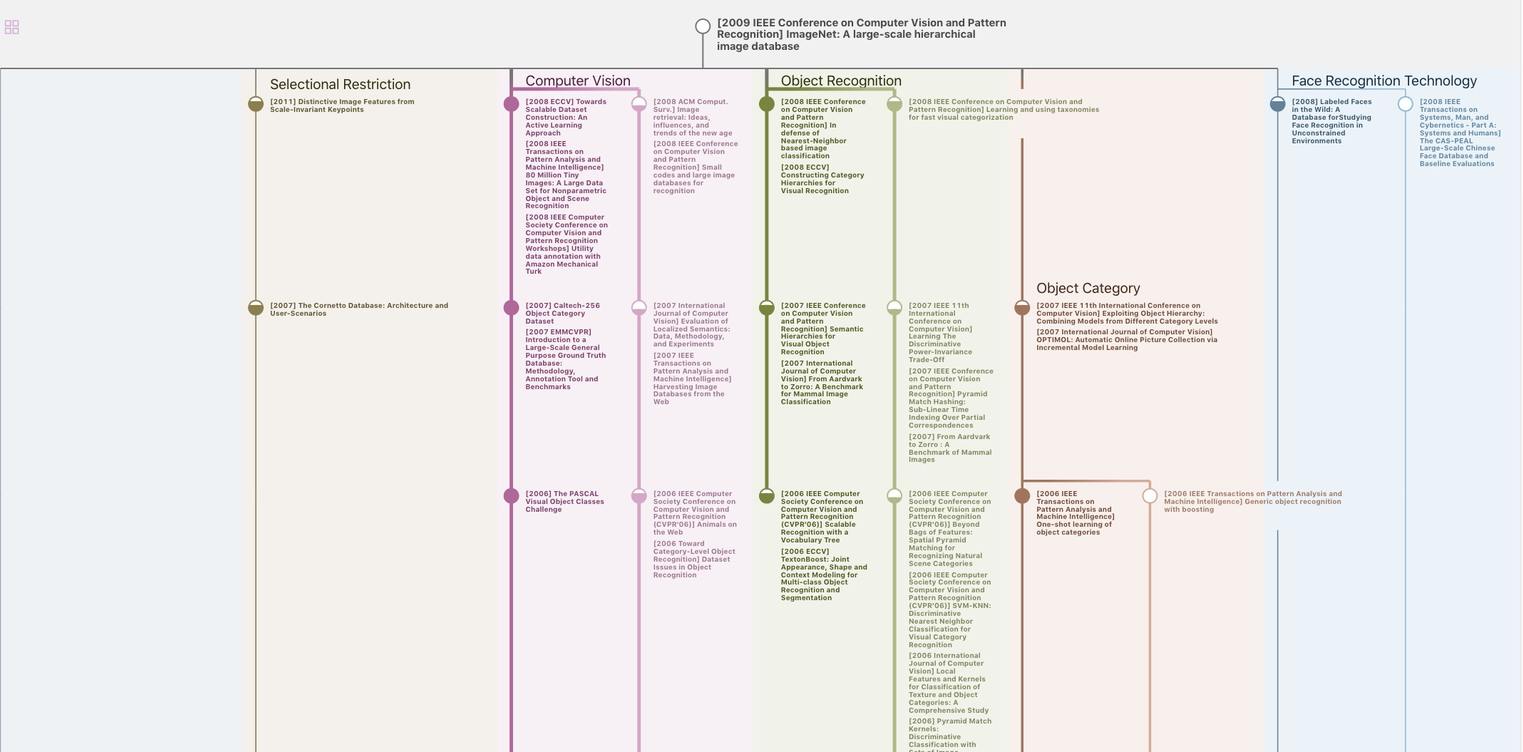
生成溯源树,研究论文发展脉络
Chat Paper
正在生成论文摘要