An Explainable Artificial Intelligence-Based Robustness Optimization Approach for Age-Related Macular Degeneration Detection Based on Medical IOT Systems
ELECTRONICS(2023)
摘要
AI-based models have shown promising results in diagnosing eye diseases based on multi-sources of data collected from medical IOT systems. However, there are concerns regarding their generalization and robustness, as these methods are prone to overfitting specific datasets. The development of Explainable Artificial Intelligence (XAI) techniques has addressed the black-box problem of machine learning and deep learning models, which can enhance interpretability and trustworthiness and optimize their performance in the real world. Age-related macular degeneration (AMD) is currently the primary cause of vision loss among elderly individuals. In this study, XAI methods were applied to detect AMD using various ophthalmic imaging modalities collected from medical IOT systems, such as colorful fundus photography (CFP), optical coherence tomography (OCT), ultra-wide fundus (UWF) images, and fluorescein angiography fundus (FAF). An optimized deep learning (DL) model and novel AMD identification systems were proposed based on the insights extracted by XAI. The findings of this study demonstrate that XAI not only has the potential to improve the transparency, reliability, and trustworthiness of AI models for ophthalmic applications, but it also has significant advantages for enhancing the robustness performance of these models. XAI could play a crucial role in promoting intelligent ophthalmology and be one of the most important techniques for evaluating and enhancing ophthalmic AI systems.
更多查看译文
关键词
explainable artificial intelligence (XAI), robustness, machine learning and deep learning, age-related macular degeneration, ophthalmology, medical IOT system
AI 理解论文
溯源树
样例
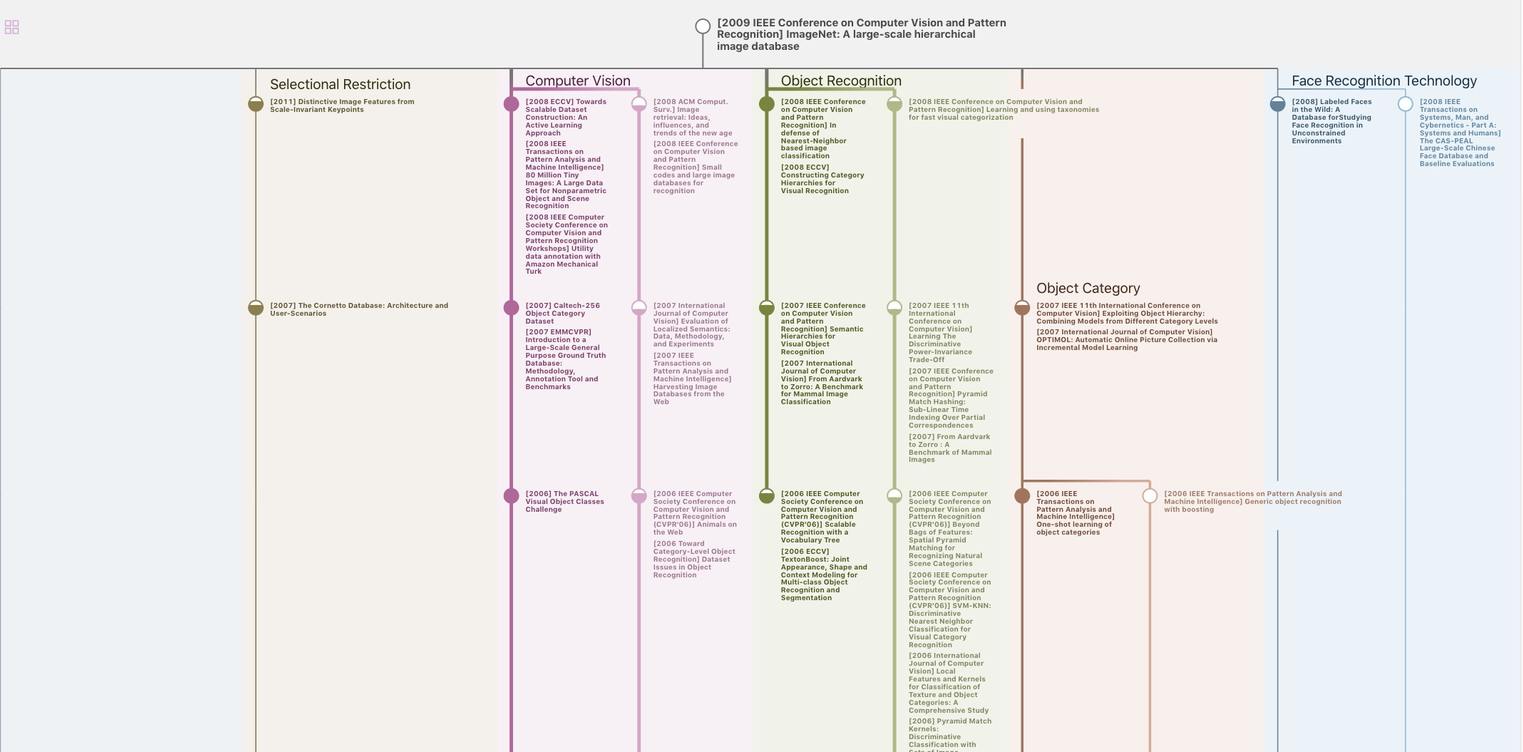
生成溯源树,研究论文发展脉络
Chat Paper
正在生成论文摘要