LEFormer: A Hybrid CNN-Transformer Architecture for Accurate Lake Extraction from Remote Sensing Imagery
ICASSP 2024 - 2024 IEEE International Conference on Acoustics, Speech and Signal Processing (ICASSP)(2023)
摘要
Lake extraction from remote sensing images is challenging due to the complex
lake shapes and inherent data noises. Existing methods suffer from blurred
segmentation boundaries and poor foreground modeling. This paper proposes a
hybrid CNN-Transformer architecture, called LEFormer, for accurate lake
extraction. LEFormer contains three main modules: CNN encoder, Transformer
encoder, and cross-encoder fusion. The CNN encoder effectively recovers local
spatial information and improves fine-scale details. Simultaneously, the
Transformer encoder captures long-range dependencies between sequences of any
length, allowing them to obtain global features and context information. The
cross-encoder fusion module integrates the local and global features to improve
mask prediction. Experimental results show that LEFormer consistently achieves
state-of-the-art performance and efficiency on the Surface Water and the
Qinghai-Tibet Plateau Lake datasets. Specifically, LEFormer achieves 90.86
97.42
while being 20 minor than the previous best lake extraction method. The source
code is available at https://github.com/BastianChen/LEFormer.
更多查看译文
关键词
Lake Extraction,CNN,Transformer,Segmentation
AI 理解论文
溯源树
样例
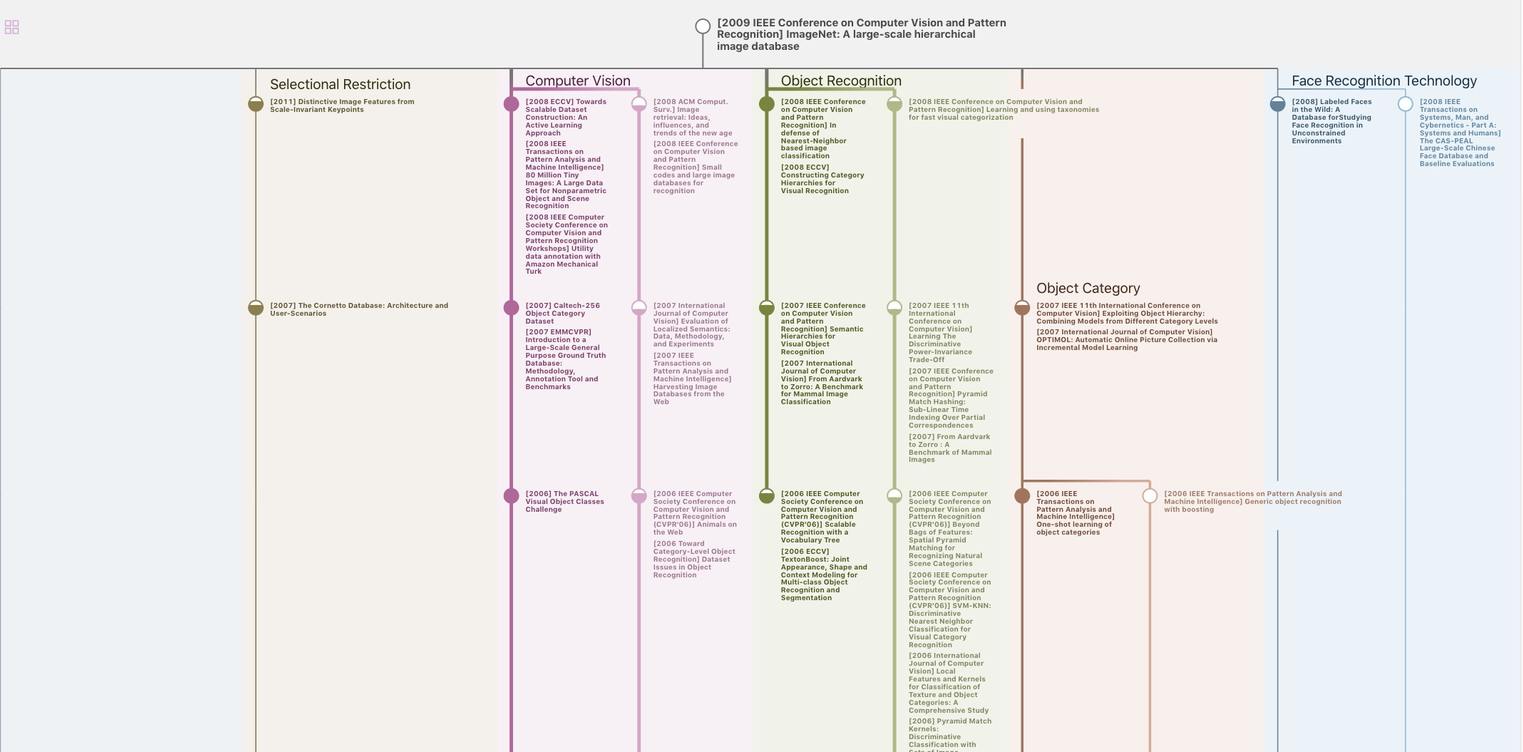
生成溯源树,研究论文发展脉络
Chat Paper
正在生成论文摘要