A Reinforcement Learning-Based Approach to Graph Discovery in D2D-Enabled Federated Learning
GLOBECOM 2023 - 2023 IEEE Global Communications Conference(2023)
摘要
Augmenting federated learning (FL) with direct device-to-device (D2D) communications can help improve convergence speed and reduce model bias through rapid local information exchange. However, data privacy concerns, device trust issues, and unreliable wireless channels each pose challenges to determining an effective yet resource efficient D2D structure. In this paper, we develop a decentralized reinforcement learning (RL) methodology for D2D graph discovery that promotes communication of non-sensitive yet impactful data-points over trusted yet reliable links. Each device functions as an RL agent, training a policy to predict the impact of incoming links. Local (device-level) and global rewards are coupled through message passing within and between device clusters. Numerical experiments confirm the advantages offered by our method in terms of convergence speed and straggler resilience across several datasets and FL schemes.
更多查看译文
关键词
Federated Learning,Information Exchange,Convergence Rate,Reinforcement Learning Agent,Message Passing,Convolutional Neural Network,Learning Task,Global Model,Wireless Networks,Local Policy,Number Of Data Points,Data Exchange,Local Dataset,Formation Of Linkages,Set Of Devices,Network Devices,Policy Update,Incoming Edges,Communication Graph,Graph Optimization,D2D Communication,Fashion-MNIST,Federated Learning Model,Notion Of Trust,Reward Model,Training Policy,Learning Models,Reward Function,Received Signal Strength,Model Aggregation
AI 理解论文
溯源树
样例
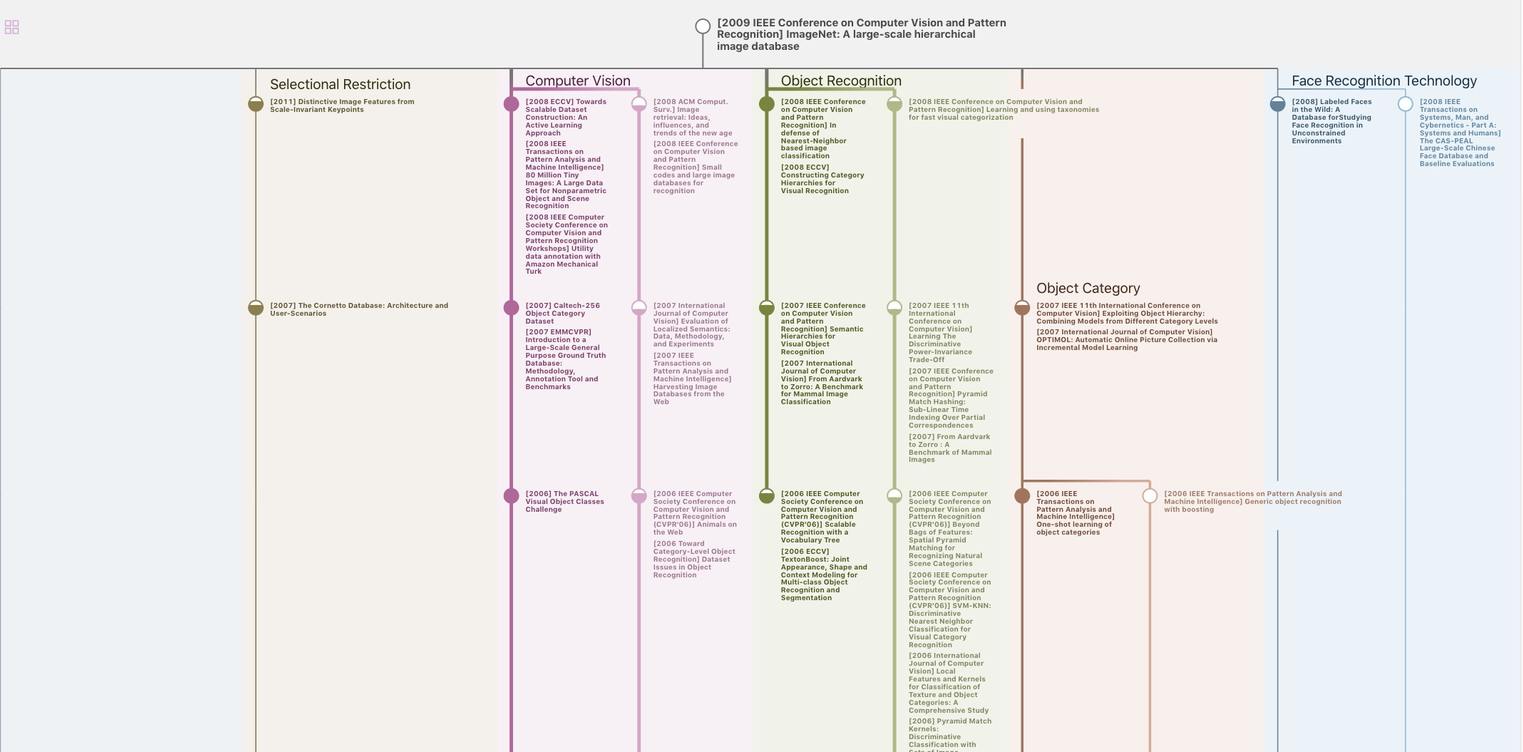
生成溯源树,研究论文发展脉络
Chat Paper
正在生成论文摘要