A Cross-Domain Evaluation of Approaches for Causal Knowledge Extraction
CoRR(2023)
摘要
Causal knowledge extraction is the task of extracting relevant causes and effects from text by detecting the causal relation. Although this task is important for language understanding and knowledge discovery, recent works in this domain have largely focused on binary classification of a text segment as causal or non-causal. In this regard, we perform a thorough analysis of three sequence tagging models for causal knowledge extraction and compare it with a span based approach to causality extraction. Our experiments show that embeddings from pre-trained language models (e.g. BERT) provide a significant performance boost on this task compared to previous state-of-the-art models with complex architectures. We observe that span based models perform better than simple sequence tagging models based on BERT across all 4 data sets from diverse domains with different types of cause-effect phrases.
更多查看译文
关键词
causal knowledge extraction,evaluation,cross-domain
AI 理解论文
溯源树
样例
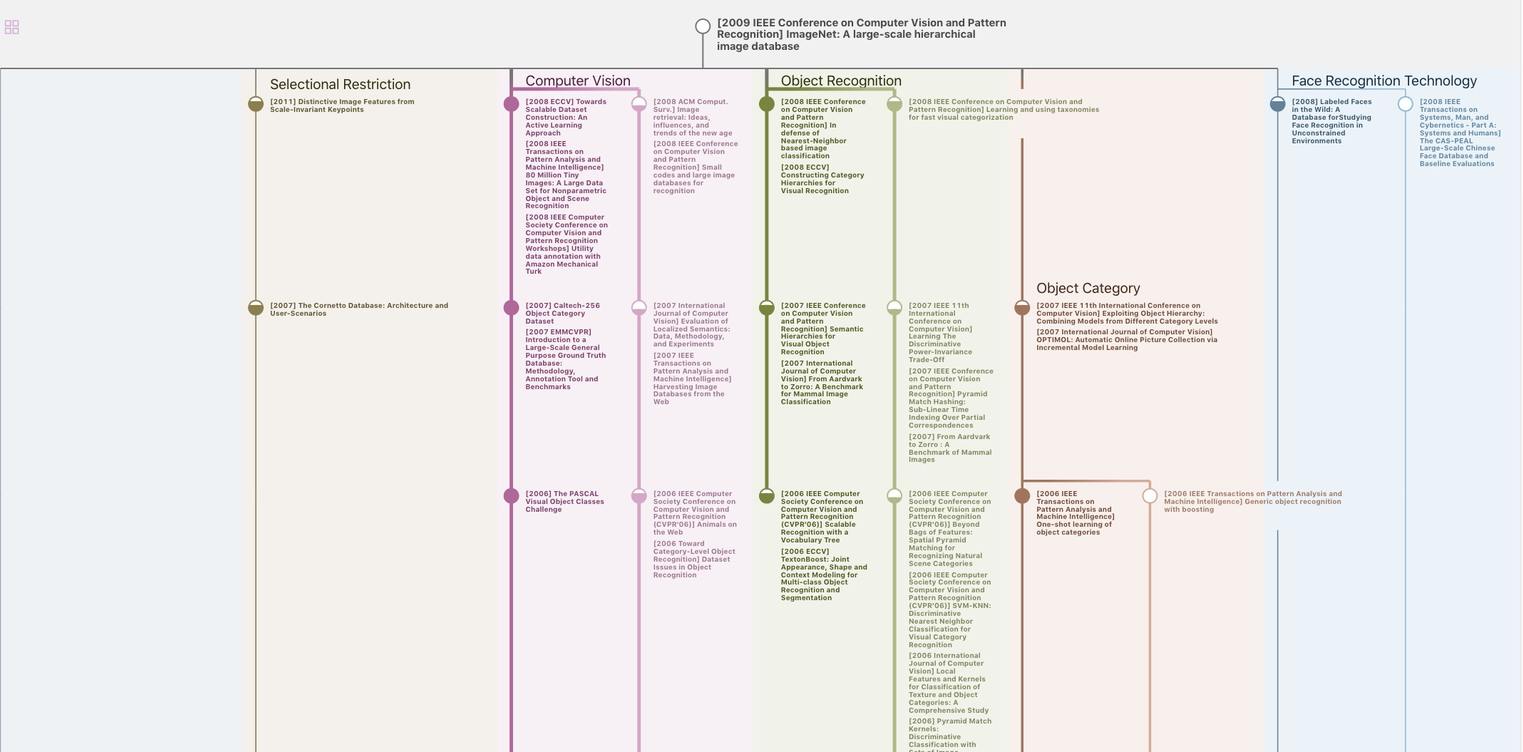
生成溯源树,研究论文发展脉络
Chat Paper
正在生成论文摘要