LISA: LIghtweight single-server Secure Aggregation with a public source of randomness
CoRR(2023)
摘要
Secure Aggregation (SA) is a key component of privacy-friendly federated learning applications, where the server learns the sum of many user-supplied gradients, while individual gradients are kept private. State-of-the-art SA protocols protect individual inputs with zero-sum random shares that are distributed across users, have a per-user overhead that is logarithmic in the number of users, and take more than 5 rounds of interaction. In this paper, we introduce LISA, an SA protocol that leverages a source of public randomness to minimize per-user overhead and the number of rounds. In particular, LISA requires only two rounds and has a communication overhead that is asymptotically equal to that of a non-private protocol -- one where inputs are provided to the server in the clear -- for most of the users. In a nutshell, LISA uses public randomness to select a subset of the users -- a committee -- that aid the server to recover the aggregated input. Users blind their individual contributions with randomness shared with each of the committee members; each committee member provides the server with an aggregate of the randomness shared with each user. Hence, as long as one committee member is honest, the server cannot learn individual inputs but only the sum of threshold-many inputs. We compare LISA with state-of-the-art SA protocols both theoretically and by means of simulations and present results of our experiments. We also integrate LISA in a Federated Learning pipeline and compare its performance with a non-private protocol.
更多查看译文
关键词
randomness,public,single-server
AI 理解论文
溯源树
样例
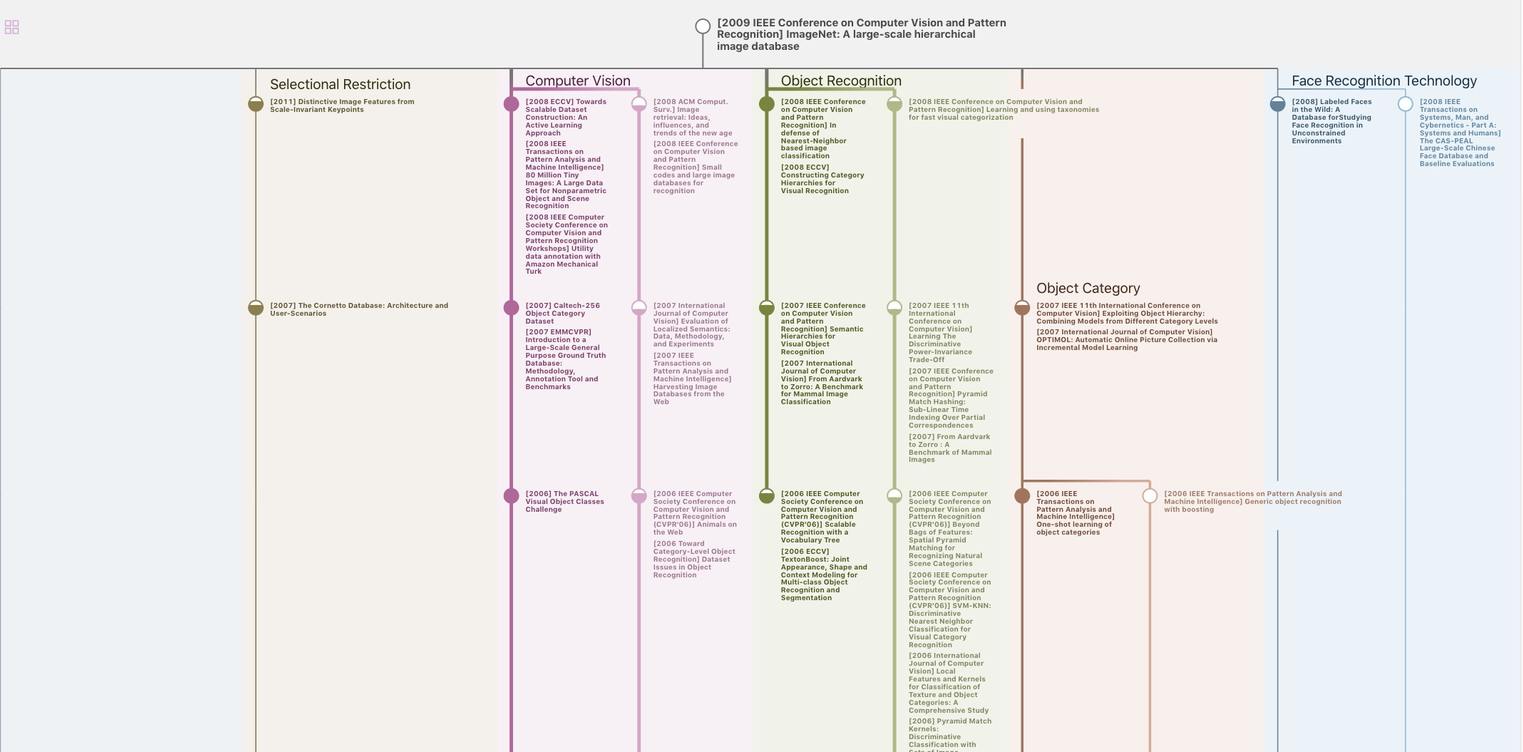
生成溯源树,研究论文发展脉络
Chat Paper
正在生成论文摘要