Knowledge updating in real-world estimation: Connecting hindsight bias and seeding effects.
Journal of experimental psychology. General(2023)
摘要
When people estimate the quantities of objects (e.g., country populations), are then presented with the objects' actual quantities, and subsequently asked to remember their initial estimates, responses are often distorted towards the actual quantities. This -traditionally considered to reflect a cognitive error-has more recently been proposed to result from adaptive knowledge updating. But how to conceptualize such knowledge-updating processes and their potentially beneficial consequences? Here, we provide a framework that conceptualizes knowledge updating in the context of hindsight bias in real-world estimation by connecting it with research on -improvements in people's estimation accuracy after exposure to numerical facts. This integrative perspective highlights a previously neglected facet of knowledge updating, namely, recalibration of metric domain knowledge, which can be expected to lead to transfer learning and thus improve estimation for objects from a domain more generally. We develop an experimental paradigm to investigate the association of hindsight bias with improved estimation accuracy. In Experiment 1, we demonstrate that the classical approach to induce hindsight bias indeed produces transfer learning. In Experiment 2, we provide evidence for the novel prediction that hindsight bias can be triggered via transfer learning; this establishes a direct link from knowledge updating to hindsight bias. Our work integrates two prominent but previously unconnected research programs on the effects of knowledge updating in real-world estimation and supports the notion that hindsight bias is driven by adaptive learning processes. (PsycInfo Database Record (c) 2023 APA, all rights reserved).
更多查看译文
关键词
knowledge,bias,estimation,real-world
AI 理解论文
溯源树
样例
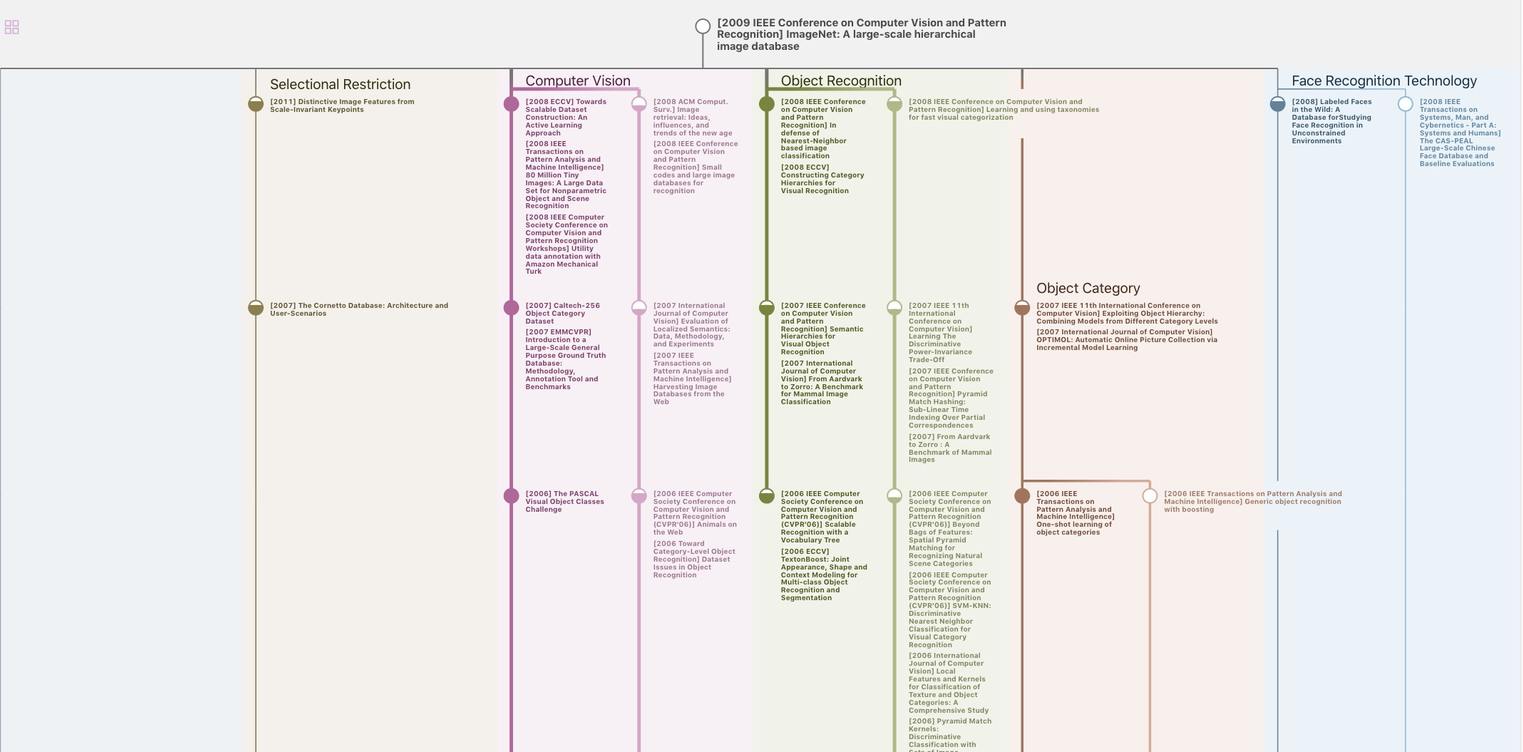
生成溯源树,研究论文发展脉络
Chat Paper
正在生成论文摘要