Time-Aware Data Profiling With Decision Tree Pattern Mining
CBI(2023)
摘要
Data quality issues can lead to significant financial losses, which necessitates the use of data profiling to monitor the statistical properties of datasets and detect harmful deviations early. However, many existing data profiling solutions cannot perform multi-column feature analysis. On the other hand, machine learning algorithms, particularly decision trees, are effective at discovering non-linear and multivariate patterns within tabular data. In this study, we propose a framework that combines the interpretable pattern-mining capabilities of decision trees with time series forecasting to identify significant changes in data. We evaluate our framework on a real-world dataset from a leading telecommunications provider in Germany, which includes a known anomaly resulting from a faulty database entry. Our results indicate that our framework successfully detects data changes and provides interpretable descriptions for each anomaly, highlighting its relevancy for practitioners.
更多查看译文
关键词
Data Drift,Data Profiling,Data Quality
AI 理解论文
溯源树
样例
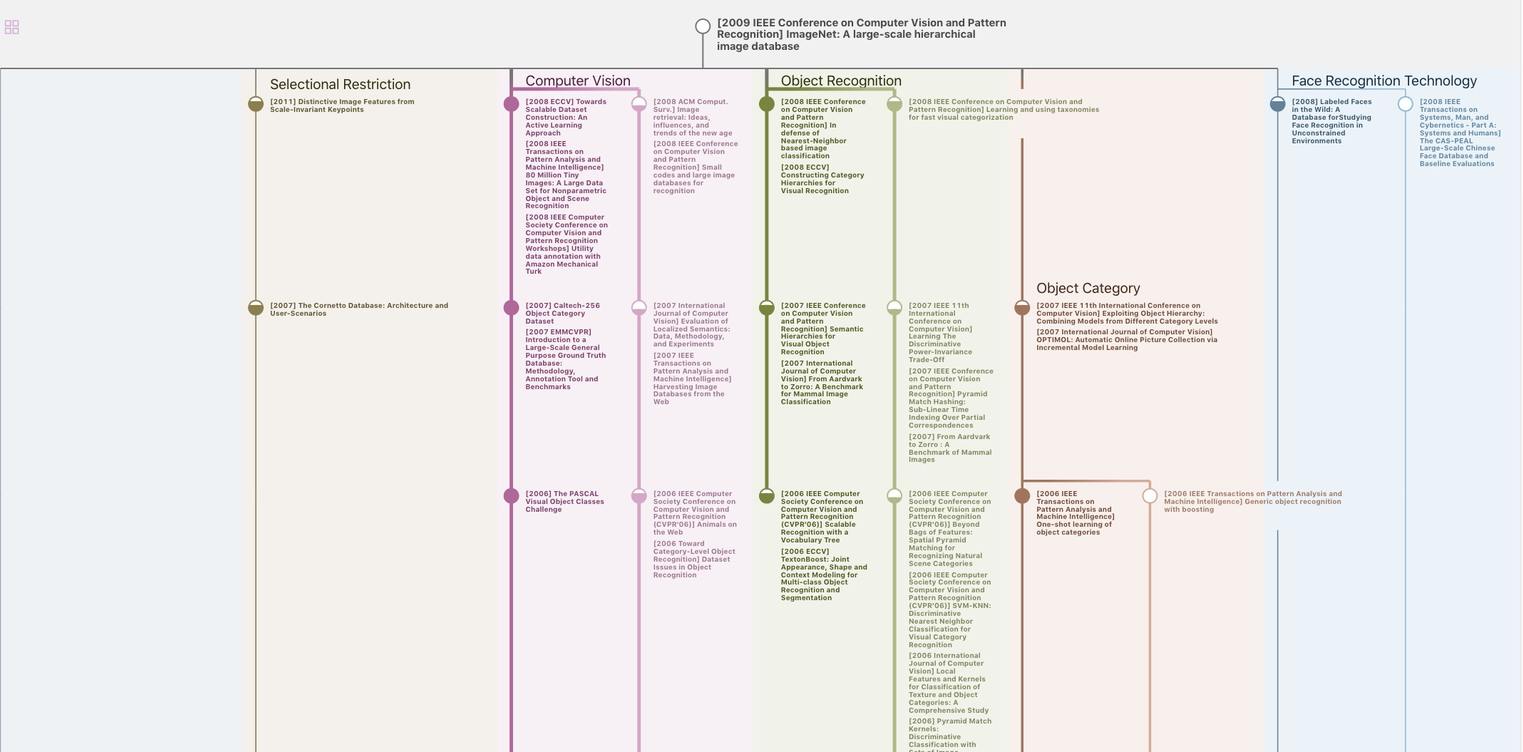
生成溯源树,研究论文发展脉络
Chat Paper
正在生成论文摘要