Interpreting High Order Epistasis Using Sparse Transformers.
CHASE(2023)
摘要
Genome-Wide Association Studies aim to identify relations between Single Nucleotide Polymorphisms (SNPs) and the manifestation of certain diseases, which is an important challenge in biomedicine and personalized healthcare. However, most genetic diseases are explained by the interactions between several SNPs, known as epistasis. Detecting epistasis is a very computationally demanding task, due to the sheer number of SNP combinations to analyze. Recently, deep learning has emerged as a possible solution for genomic prediction, but the black-box nature of neural networks and the lack of explainability is a drawback yet to be solved. In this paper, a new, flexible framework for interpreting neural networks for any-order epistasis detection is presented. Using sparse transformers, a technique not yet employed for epistasis detection, different SNP representations are explored and attention scores are assigned to each SNP to quantify its relevance for phenotype prediction. The results on simulated datasets show that the proposed framework outperforms state-of-the-art methods for explainability, identifying SNP interactions in diverse epistasis scenarios. The proposed frame-work is validated on a real breast cancer dataset, identifying second to fifth order interactions in the top 40% most relevant SNPs.
更多查看译文
关键词
Genome-Wide Association Studies, Epistasis Detection, Machine Learning
AI 理解论文
溯源树
样例
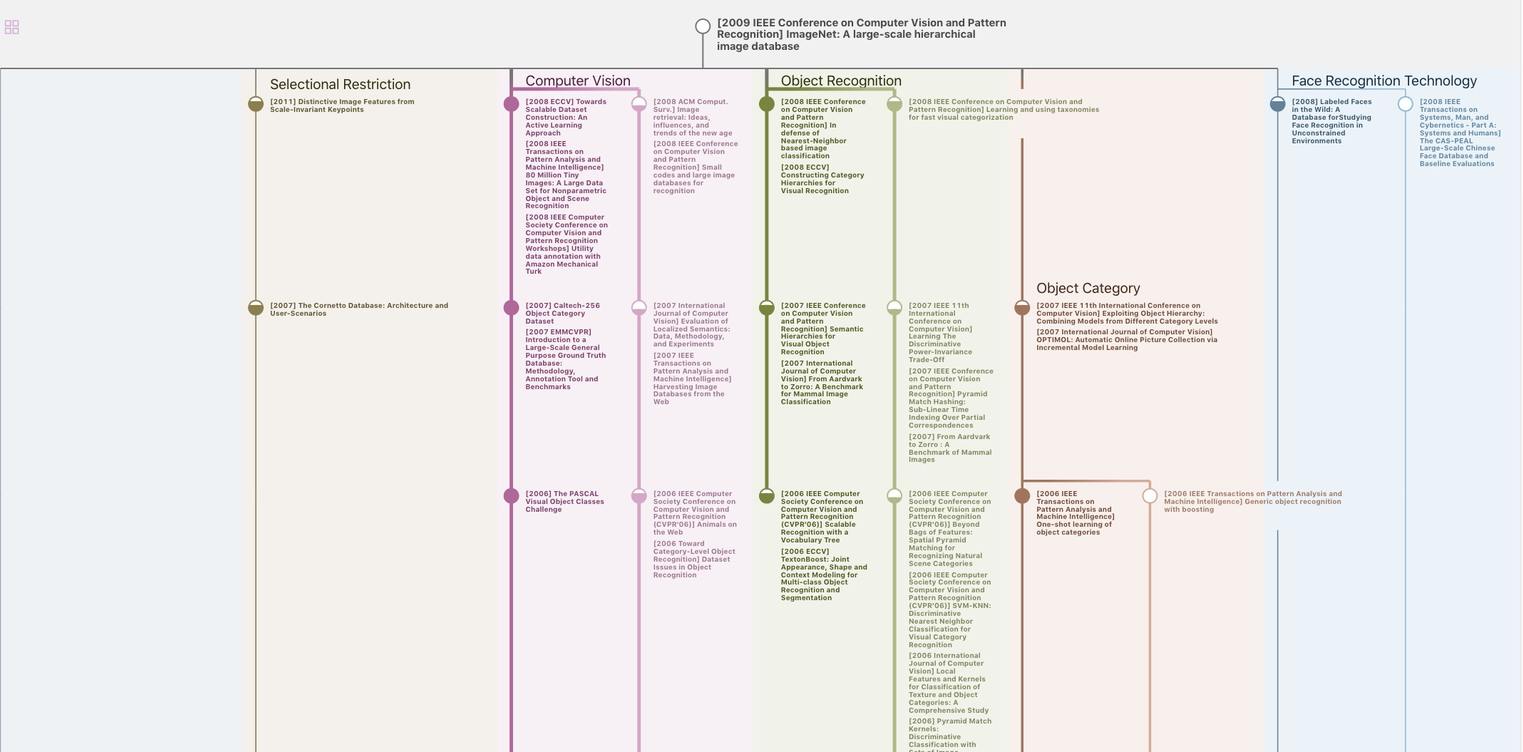
生成溯源树,研究论文发展脉络
Chat Paper
正在生成论文摘要