Towards Generalizing Machine Learning Models to Detect Command and Control Attack Traffic.
CyCon(2023)
摘要
Identifying compromised hosts from network traffic traces has become challenging because benign and malicious traffic is encrypted, and both use the same protocols and ports. Machine learning-based anomaly detection models have been proposed to address this challenge by classifying malicious traffic based on network flow features learned from historical patterns. Previous work has shown that such models successfully identify compromised hosts in the same network environment in which they were trained. However, cyber incident response teams often have to look for intrusions in foreign networks, and we have found that learned models often fail to generalize to different network conditions. In this paper, we analyse the root cause of this problem using five network traces collected from different years and teams of Locked Shields, the world's largest live-fire cyber defence exercise. We then explore techniques to make machine learning models generalize better to unknown network environments and evaluate their accuracy.
更多查看译文
关键词
machine learning, traffic classification, network security, command and control, Locked Shields
AI 理解论文
溯源树
样例
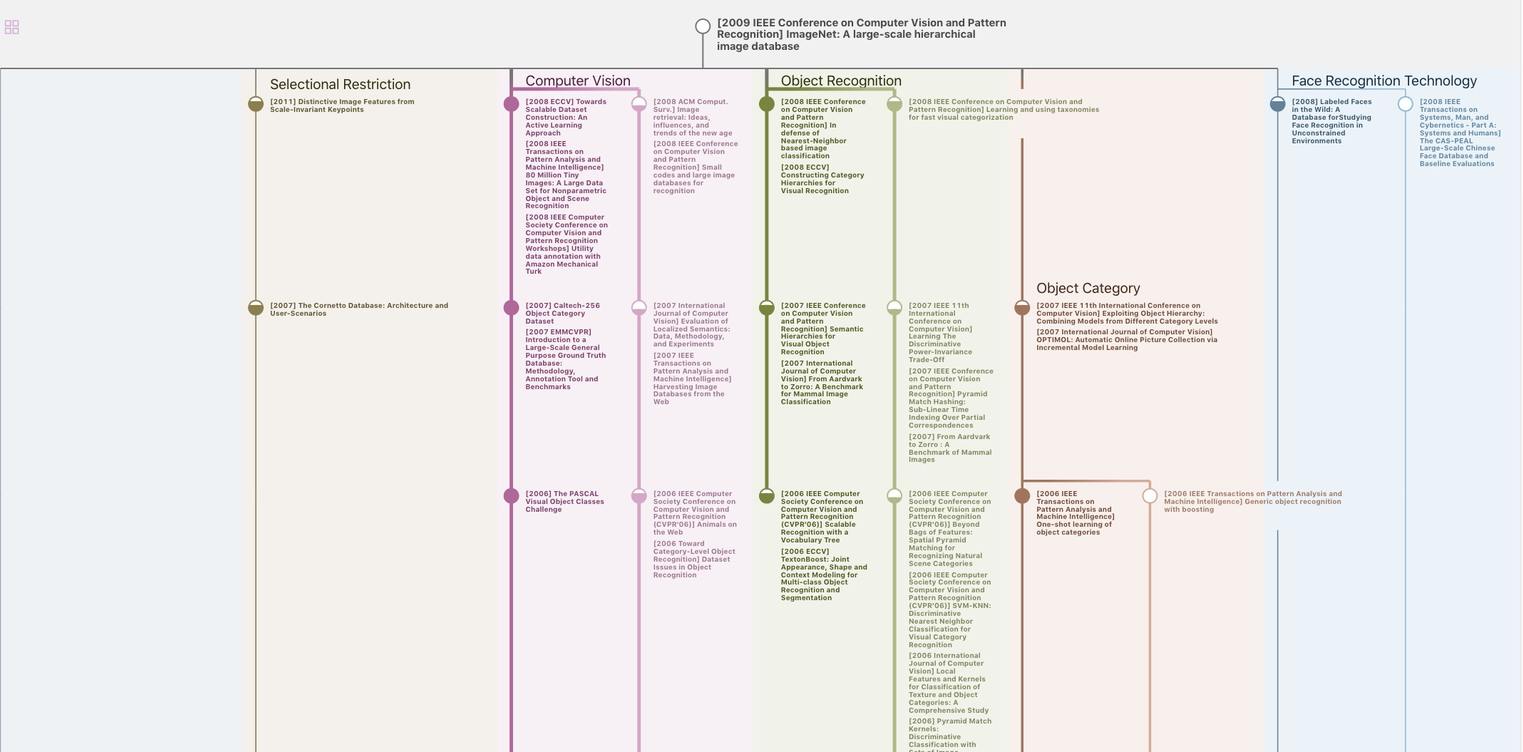
生成溯源树,研究论文发展脉络
Chat Paper
正在生成论文摘要