Effective Continual Learning for Text Classification with Lightweight Snapshots.
AAAI(2023)
摘要
Continual learning is known for suffering from catastrophic forgetting, a phenomenon where previously learned concepts are forgotten upon learning new tasks. A natural remedy is to use trained models for old tasks as 'teachers' to regularize the update of the current model to prevent such forgetting. However, this requires storing all past models, which is very space-consuming for large models, e.g. BERT, thus impractical in real-world applications. To tackle this issue, we propose to construct snapshots of seen tasks whose key knowledge is captured in lightweight adapters. During continual learning, we transfer knowledge from past snapshots to the current model through knowledge distillation, allowing the current model to review previously learned knowledge while learning new tasks. We also design representation recalibration to better handle the class-incremental setting. Experiments over various task sequences show that our approach effectively mitigates catastrophic forgetting and outperforms all baselines.
更多查看译文
关键词
effective continual learning,text classification,lightweight snapshots
AI 理解论文
溯源树
样例
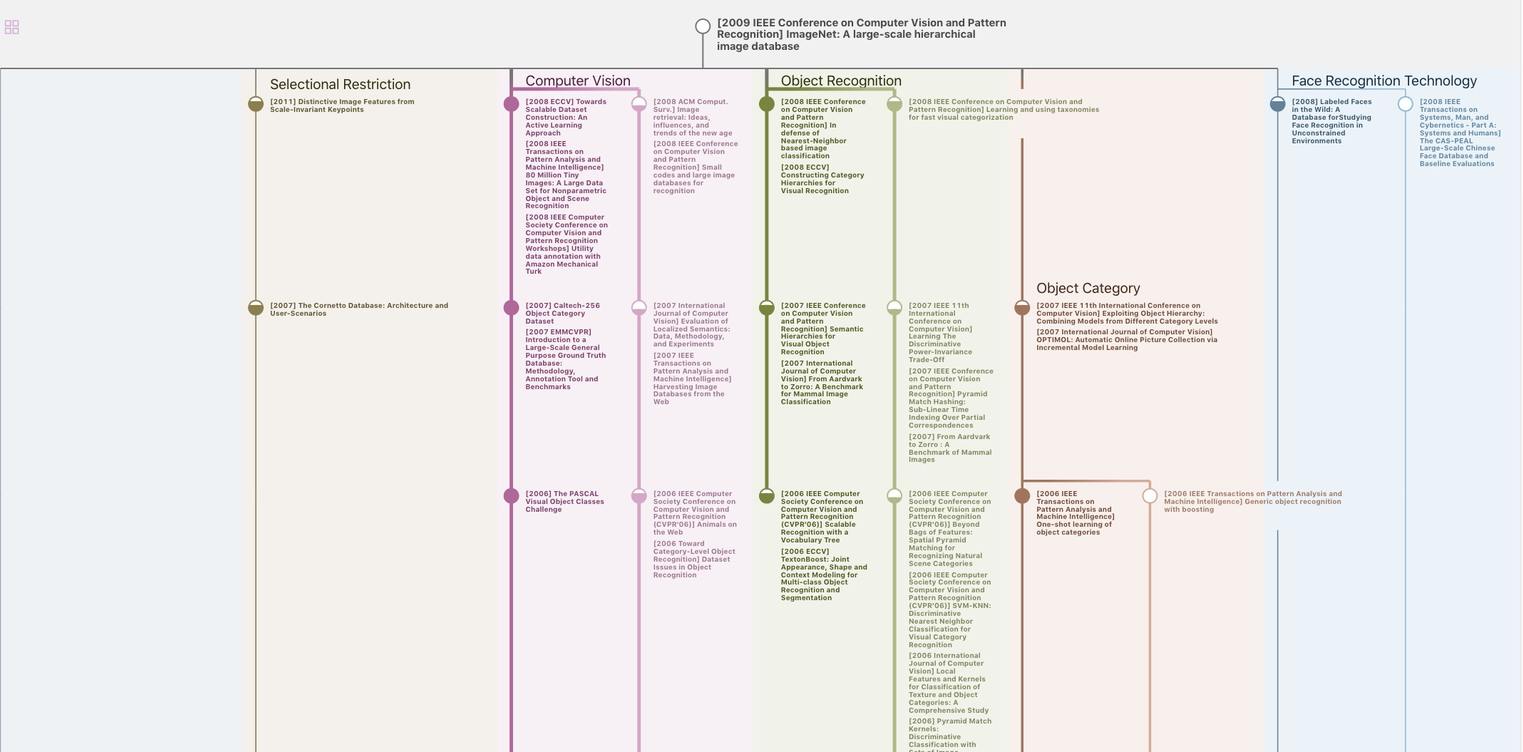
生成溯源树,研究论文发展脉络
Chat Paper
正在生成论文摘要