Improving Dialogue Intent Classification with a Knowledge-Enhanced Multifactor Graph Model (Student Abstract).
AAAI(2023)
摘要
Although current Graph Neural Network (GNN) based models achieved good performances in Dialogue Intent Classification (DIC), they leave the inherent domain-specific knowledge out of consideration, leading to the lack of ability of acquiring fine-grained semantic information. In this paper, we propose a Knowledge-Enhanced Multifactor Graph (KEMG) Model for DIC. We firstly present a knowledge-aware utterance encoder with the help of a domain-specific knowledge graph, fusing token-level and entity-level semantic information, then design a heterogeneous dialogue graph encoder by explicitly modeling several factors that matter to contextual modeling of dialogues. Experiment results show that our proposed method outperforms other GNN-based methods on a dataset collected from a real-world online customer service dialogue system on the e-commerce website, JD.
更多查看译文
关键词
dialogue intent classification,student abstract,knowledge-enhanced
AI 理解论文
溯源树
样例
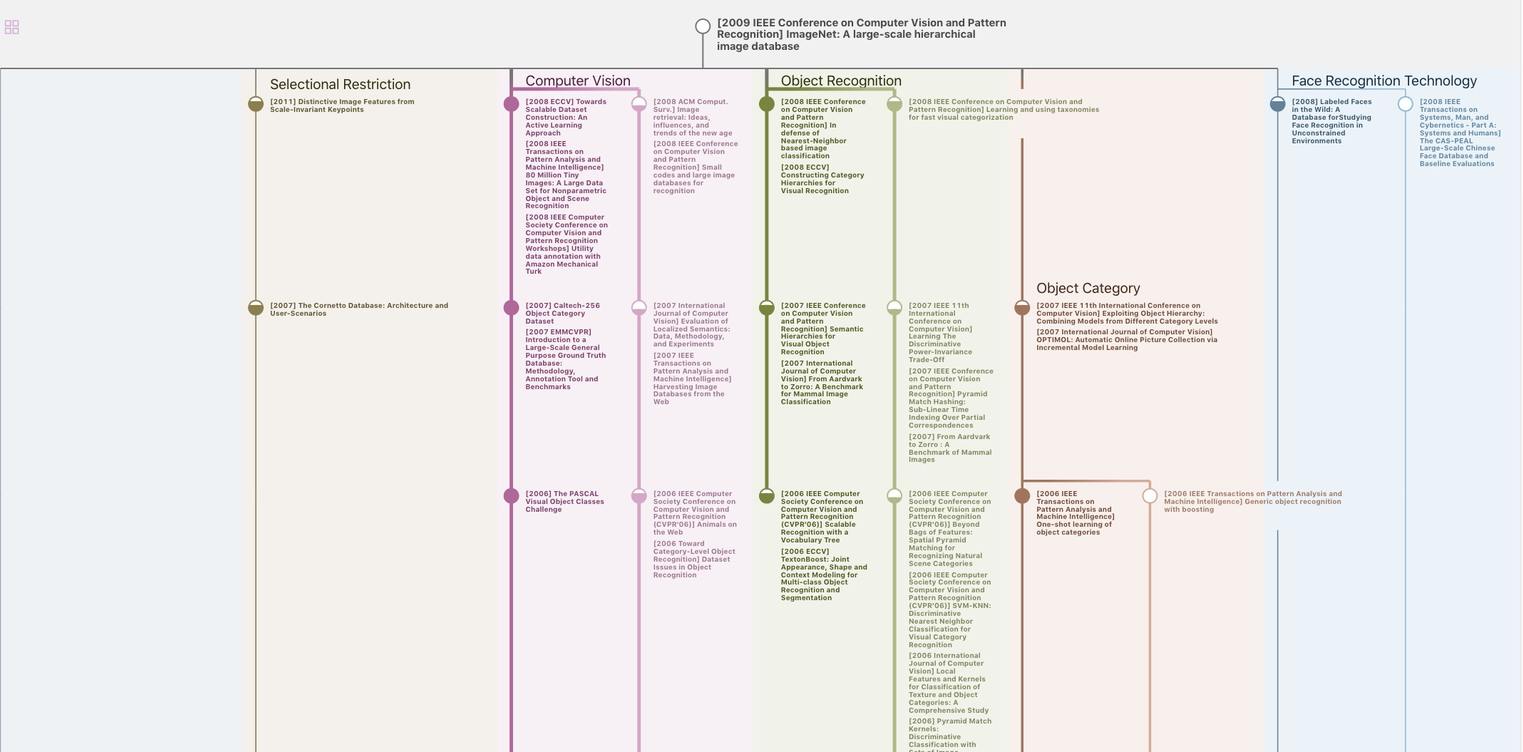
生成溯源树,研究论文发展脉络
Chat Paper
正在生成论文摘要