Preserve Context Information for Extract-Generate Long-Input Summarization Framework.
AAAI(2023)
摘要
The Extract-generate framework has been a classic approach for text summarization. As pretrained language models struggling with long-input summarization for their high memory cost, extract-generate framework regains researchers' interests. However, the cost of its effectiveness in dealing with long-input summarization is the loss of context information. In this paper, we present a context-aware extract-generate framework (CAEG) for long-input text summarization. It focuses on preserving both local and global context information in an extract-generate framework with little cost, and can be applied to most of existing extract-generate summarization models. CAEG generates a set of context-related text spans called context prompts for each text snippet and use them to transfer the context information from the extractor and generator. To find such context prompts, we propose to capture the context information based on the interpretation of the extractor, where the text spans having the highest contribution to the extraction decision are considered as containing the richest context information. We evaluate our approach on both long-document and long-dialogue summarization datasets: arXiv and QMSum. The experiment results show that CAEG achieves the-state-of-art result on QMSum and outperforms other extract-generate based models in arXiv.
更多查看译文
关键词
context information,extract-generate,long-input
AI 理解论文
溯源树
样例
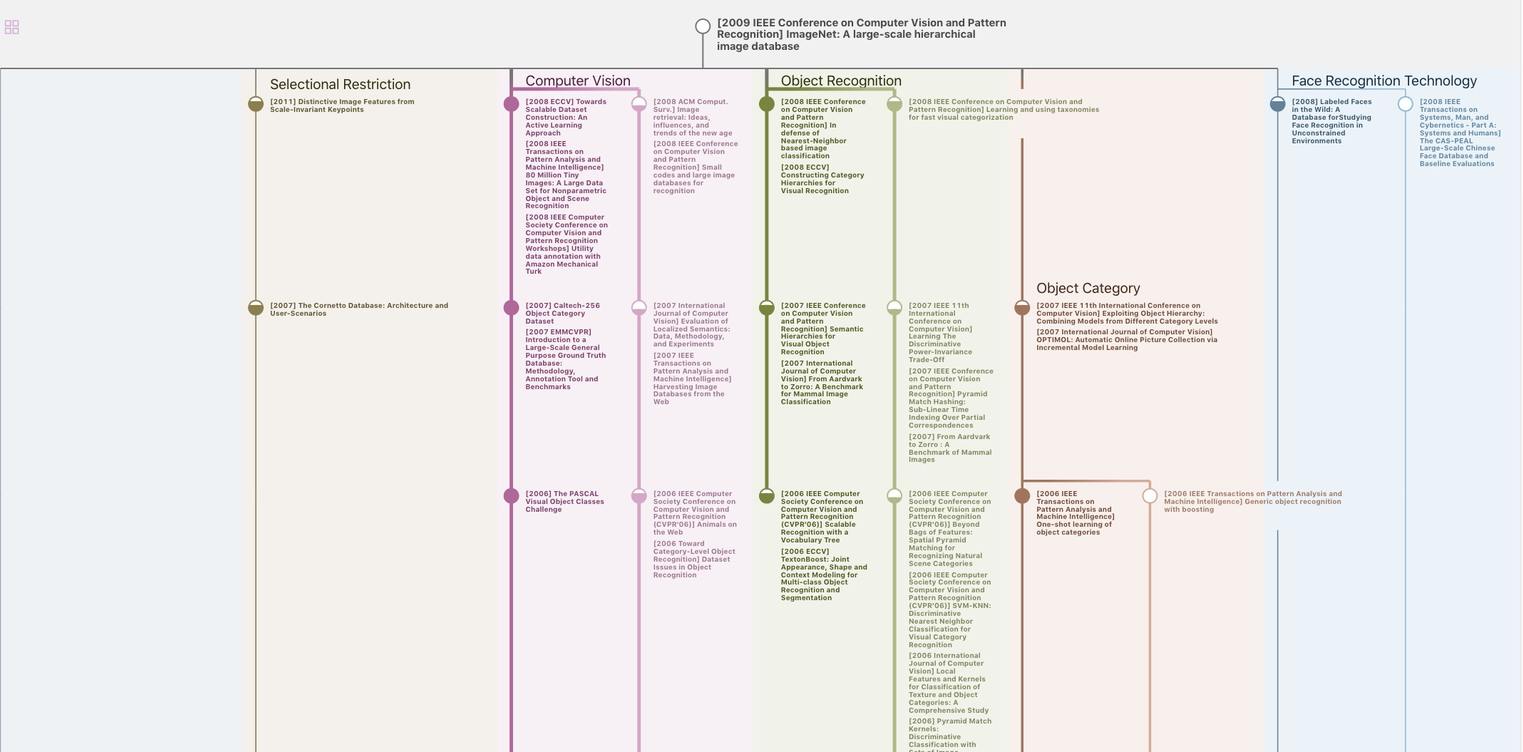
生成溯源树,研究论文发展脉络
Chat Paper
正在生成论文摘要