Learning Dynamic Temporal Relations with Continuous Graph for Multivariate Time Series Forecasting (Student Abstract).
AAAI(2023)
摘要
The recent advance in graph neural networks (GNNs) has inspired a few studies to leverage the dependencies of variables for time series prediction. Despite the promising results, existing GNN-based models cannot capture the global dynamic relations between variables owing to the inherent limitation of their graph learning module. Besides, multi-scale temporal information is usually ignored or simply concatenated in prior methods, resulting in inaccurate predictions. To overcome these limitations, we present CGMF, a Continuous Graph learning method for Multivariate time series Forecasting (CGMF). Our CGMF consists of a continuous graph module incorporating differential equations to capture the longrange intra-and inter-relations of the temporal embedding sequence. We also introduce a controlled differential equation-based fusion mechanism that efficiently exploits multi-scale representations to form continuous evolutional dynamics and learn rich relations and patterns shared across different scales. Comprehensive experiments demonstrate the effectiveness of our method for a variety of datasets.
更多查看译文
关键词
dynamic temporal relations,multivariate time series forecasting,continuous graph
AI 理解论文
溯源树
样例
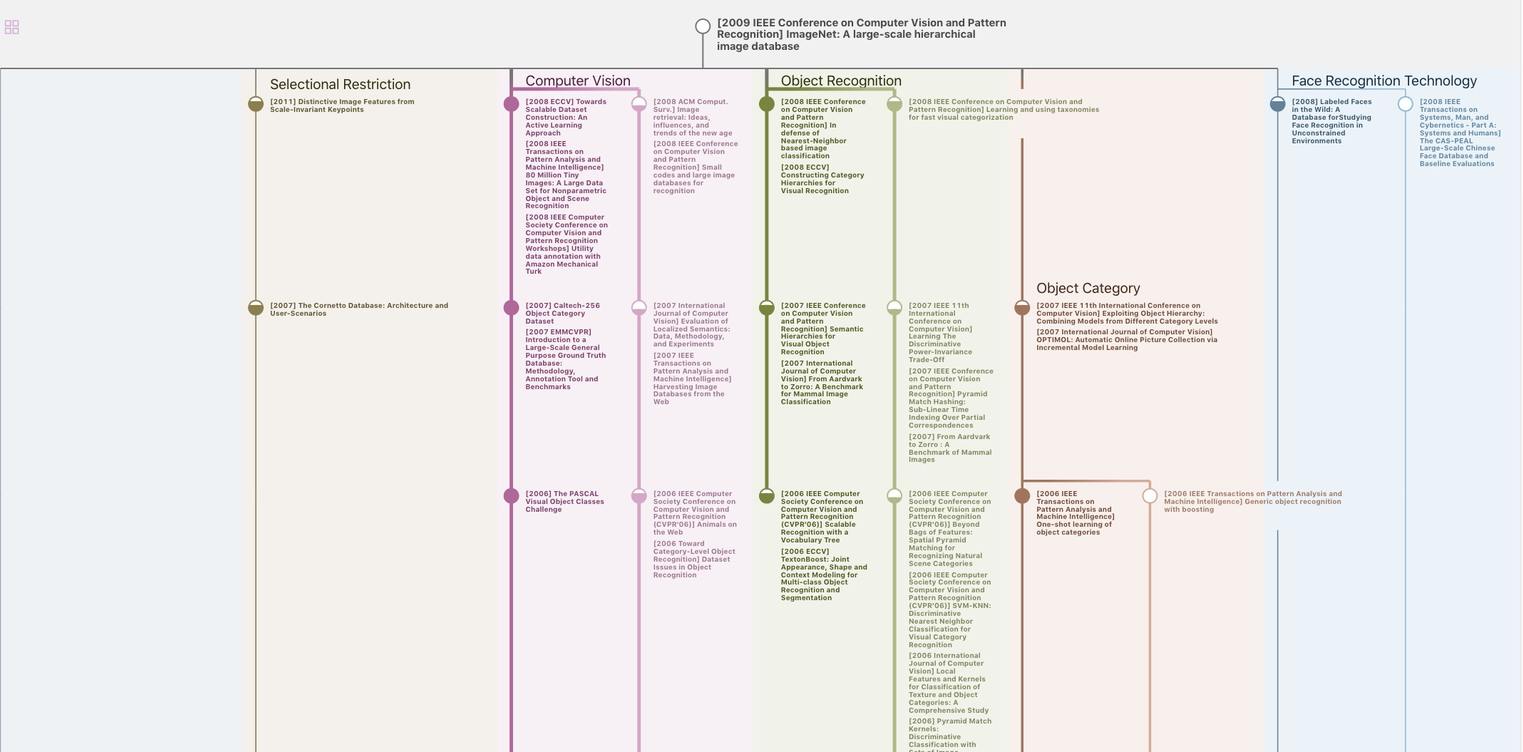
生成溯源树,研究论文发展脉络
Chat Paper
正在生成论文摘要