Neural Policy Safety Verification via Predicate Abstraction: CEGAR.
AAAI(2023)
摘要
Neural networks (NN) are an increasingly important representation of action policies π. Recent work has extended predicate abstraction to prove safety of such π, through policy predicate abstraction (PPA) which over-approximates the state space subgraph induced by π. The advantage of PPA is that reasoning about the NN - calls to SMT solvers - is required only locally, at individual abstract state transitions, in contrast to bounded model checking (BMC) where SMT must reason globally about sequences of NN decisions. Indeed, it has been shown that PPA can outperform a simple BMC implementation. However, the abstractions underlying these results (i.e., the abstraction predicates) were supplied manually. Here we automate this step. We extend counterexample-guided abstraction refinement (CEGAR) to PPA. This involves dealing with a new source of spuriousness in abstract unsafe paths, pertaining not to transition behavior but to the decisions of the neural network π. We introduce two methods tackling this issue based on the states involved, and we show that global SMT calls deciding spuriousness exactly can be avoided. We devise algorithmic enhancements leveraging incremental computation and heuristic search. We show empirically that the resulting verification tool has significant advantages over an encoding into the state-of-the-art model checker nuXmv. In particular, ours is the only approach in our experiments that succeeds in proving policies safe.
更多查看译文
关键词
neural policy safety verification,predicate abstraction
AI 理解论文
溯源树
样例
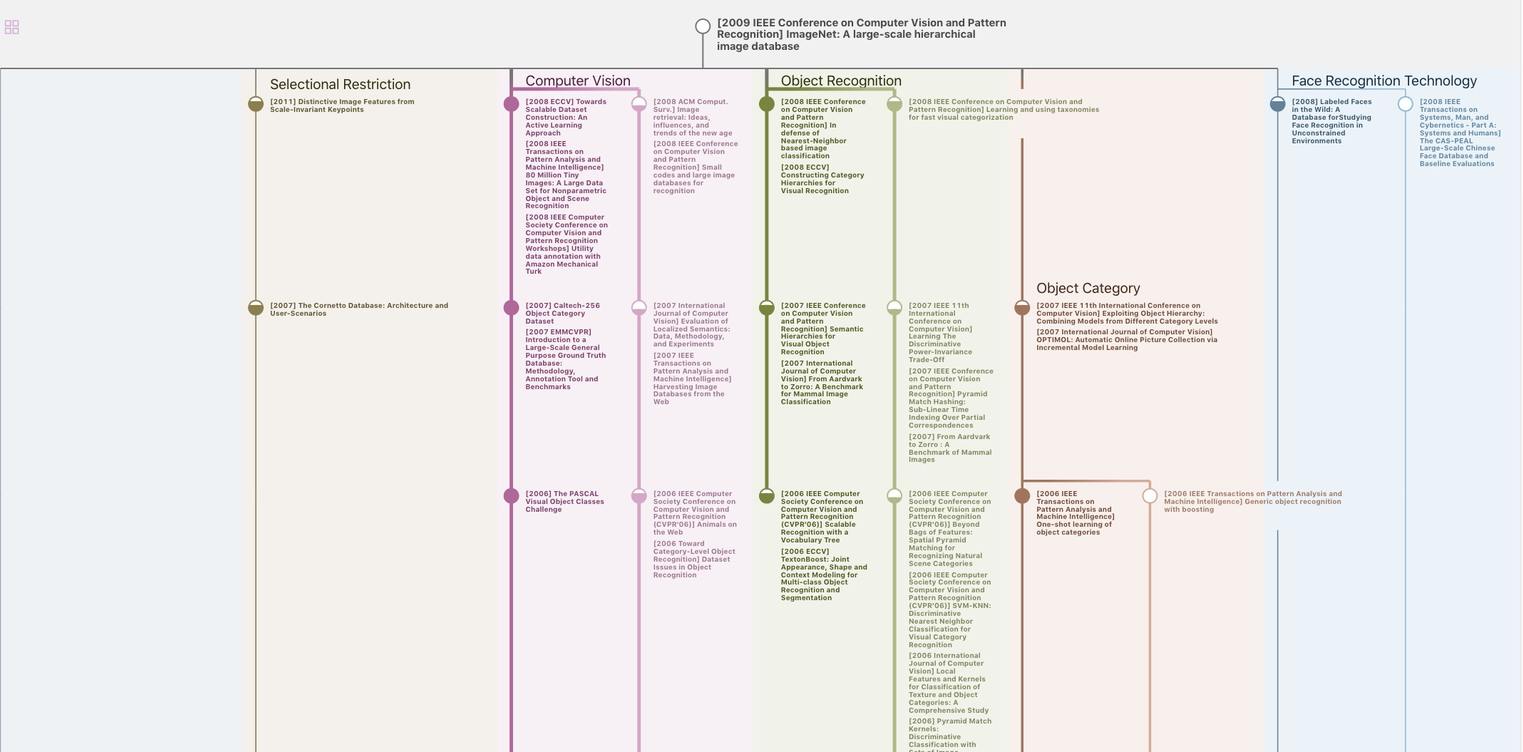
生成溯源树,研究论文发展脉络
Chat Paper
正在生成论文摘要