Incentive Mechanism Design for Responsible Data Governance: A Large-scale Field Experiment.
ACM J. Data Inf. Qual.(2023)
摘要
A crucial building block of responsible artificial intelligence is responsible data governance, including data collection. Its importance is also underlined in the latest EU regulations. The data should be of high quality, foremost correct and representative, and individuals providing the data should have autonomy over what data is collected. In this article, we consider the setting of collecting personally measured fitness data (physical activity measurements), in which some individuals may not have an incentive to measure and report accurate data. This can significantly degrade the quality of the collected data. On the other hand, high-quality collective data of this nature could be used for reliable scientific insights or to build trustworthy artificial intelligence applications. We conduct a framed field experiment (N = 691) to examine the effect of offering fixed and quality-dependent monetary incentives on the quality of the collected data. We use a peer-based incentive-compatible mechanism for the quality-dependent incentives without spot-checking or surveilling individuals. We find that the incentive-compatible mechanism can elicit good-quality data while providing a good user experience and compensating fairly, although, in the specific study context, the data quality does not necessarily differ under the two incentive schemes. We contribute new design insights from the experiment and discuss directions that future field experiments and applications on explainable and transparent data collection may focus on.
更多查看译文
关键词
responsible data governance,large-scale
AI 理解论文
溯源树
样例
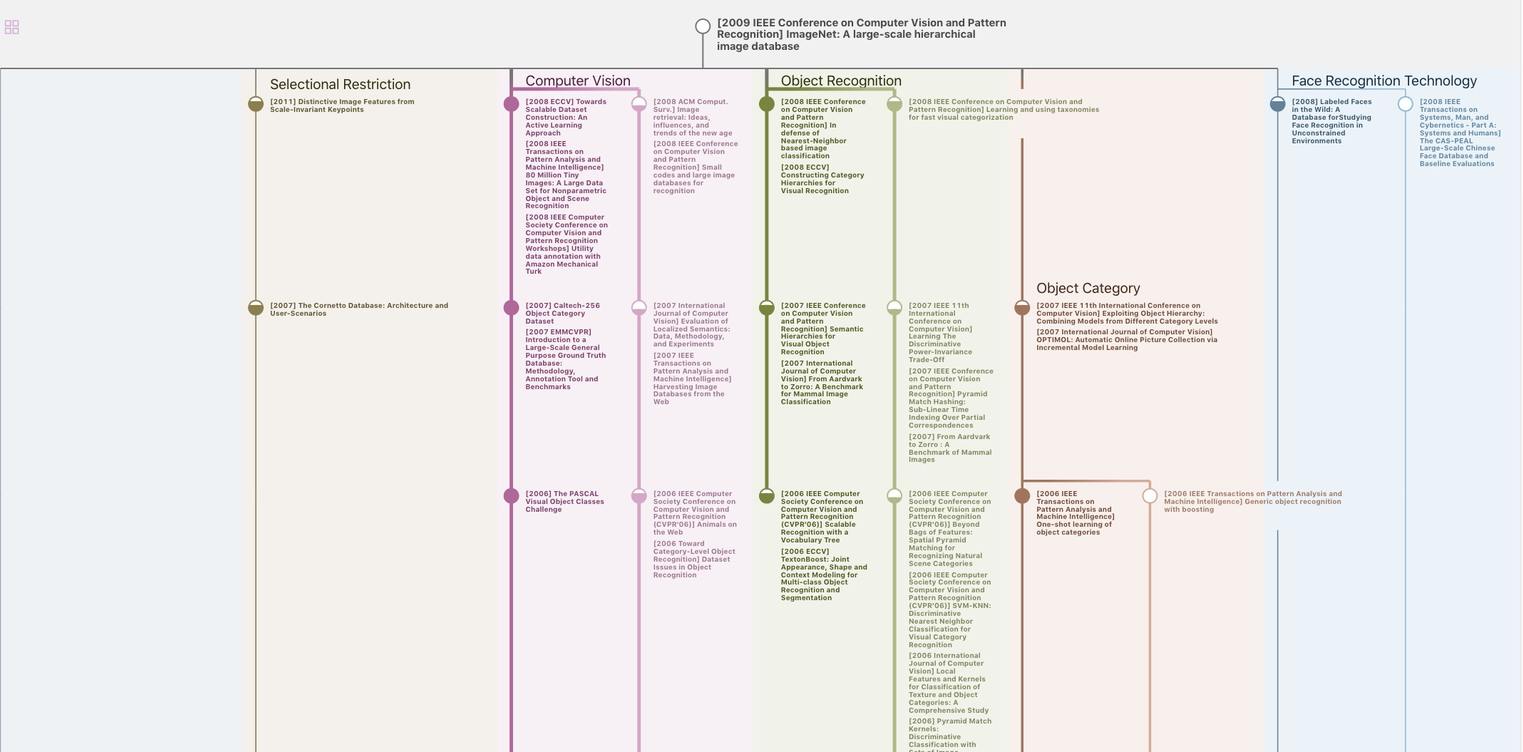
生成溯源树,研究论文发展脉络
Chat Paper
正在生成论文摘要