Causal thinking for decision making on Electronic Health Records: why and how
arXiv (Cornell University)(2023)
摘要
Accurate predictions, as with machine learning, may not suffice to provide optimal healthcare for every patient. Indeed, prediction can be driven by shortcuts in the data, such as racial biases. Causal thinking is needed for data-driven decisions. Here, we give an introduction to the key elements, focusing on routinely-collected data, electronic health records (EHRs) and claims data. Using such data to assess the value of an intervention requires care: temporal dependencies and existing practices easily confound the causal effect. We present a step-by-step framework to help build valid decision making from real-life patient records by emulating a randomized trial before individualizing decisions, eg with machine learning. Our framework highlights the most important pitfalls and considerations in analysing EHRs or claims data to draw causal conclusions. We illustrate the various choices in studying the effect of albumin on sepsis mortality in the Medical Information Mart for Intensive Care database (MIMIC-IV). We study the impact of various choices at every step, from feature extraction to causal-estimator selection. In a tutorial spirit, the code and the data are openly available.
更多查看译文
关键词
electronic health records,causal,decision making,thinking
AI 理解论文
溯源树
样例
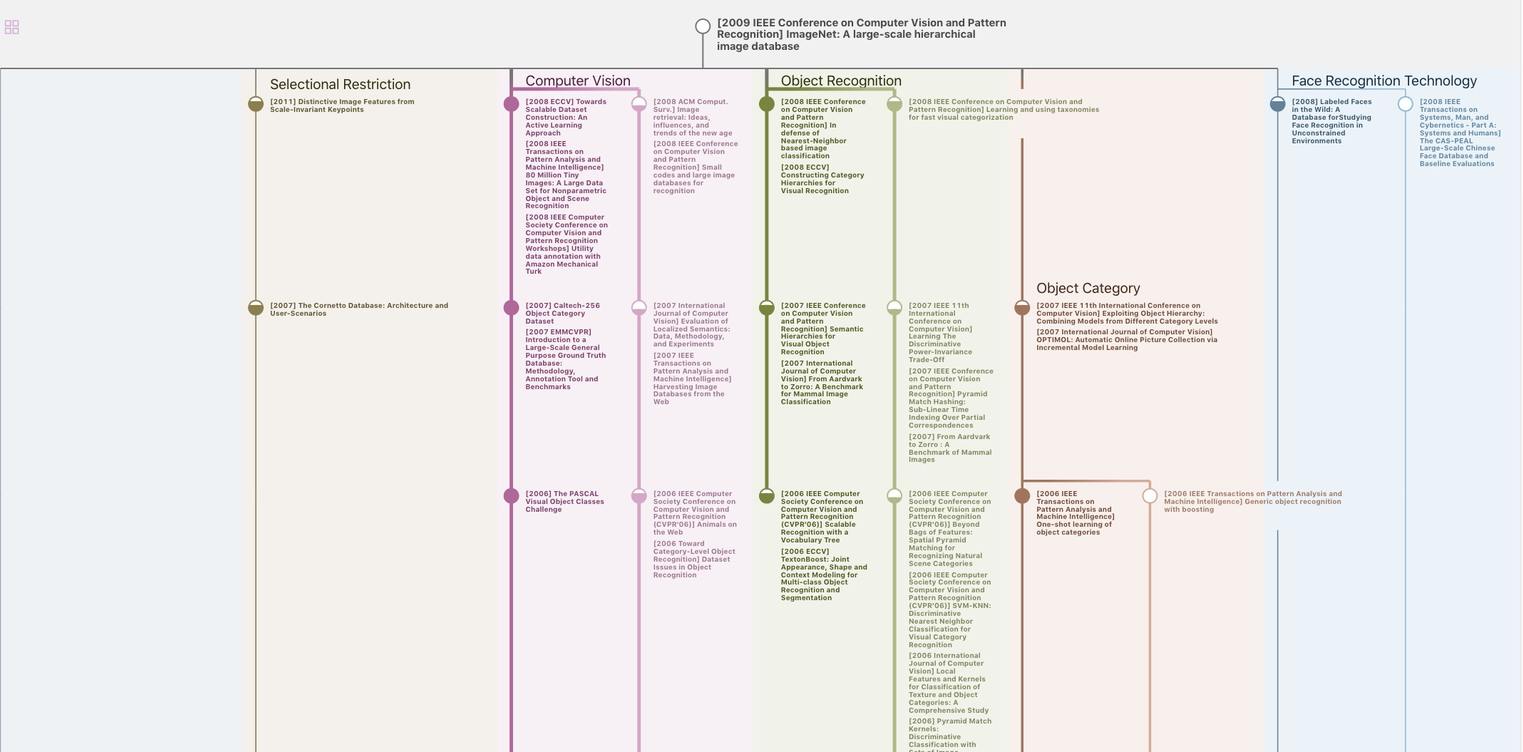
生成溯源树,研究论文发展脉络
Chat Paper
正在生成论文摘要