Classifying seismograms using the FastMap algorithm and support-vector machines
Communications Engineering(2023)
摘要
Neural networks and related deep learning methods are currently at the leading edge of technologies used for classifying complex objects such as seismograms. However they generally demand large amounts of time and data for model training and their learned models can sometimes be difficult to interpret. FastMapSVM is an interpretable machine learning framework for classifying complex objects, combining the complementary strengths of FastMap with support vector machines (SVMs) and extending the applicability of SVMs to domains with complex objects. FastMap is an efficient linear-time algorithm that maps complex objects to points in a Euclidean space while preserving pairwise domain-specific distances between them. Here we invoke FastMapSVM as a lightweight alternative to neural networks for classifying seismograms. We demonstrate that FastMapSVM outperforms other state-of-the-art methods for classifying seismograms when train data or time is limited. We also show that FastMapSVM can provide an insightful visualization of seismogram clustering behaviour and thus earthquake classification boundaries. We expect FastMapSVM to be viable for classification tasks in many other real-world domains.
更多查看译文
关键词
seismograms,fastmap algorithm,support-vector
AI 理解论文
溯源树
样例
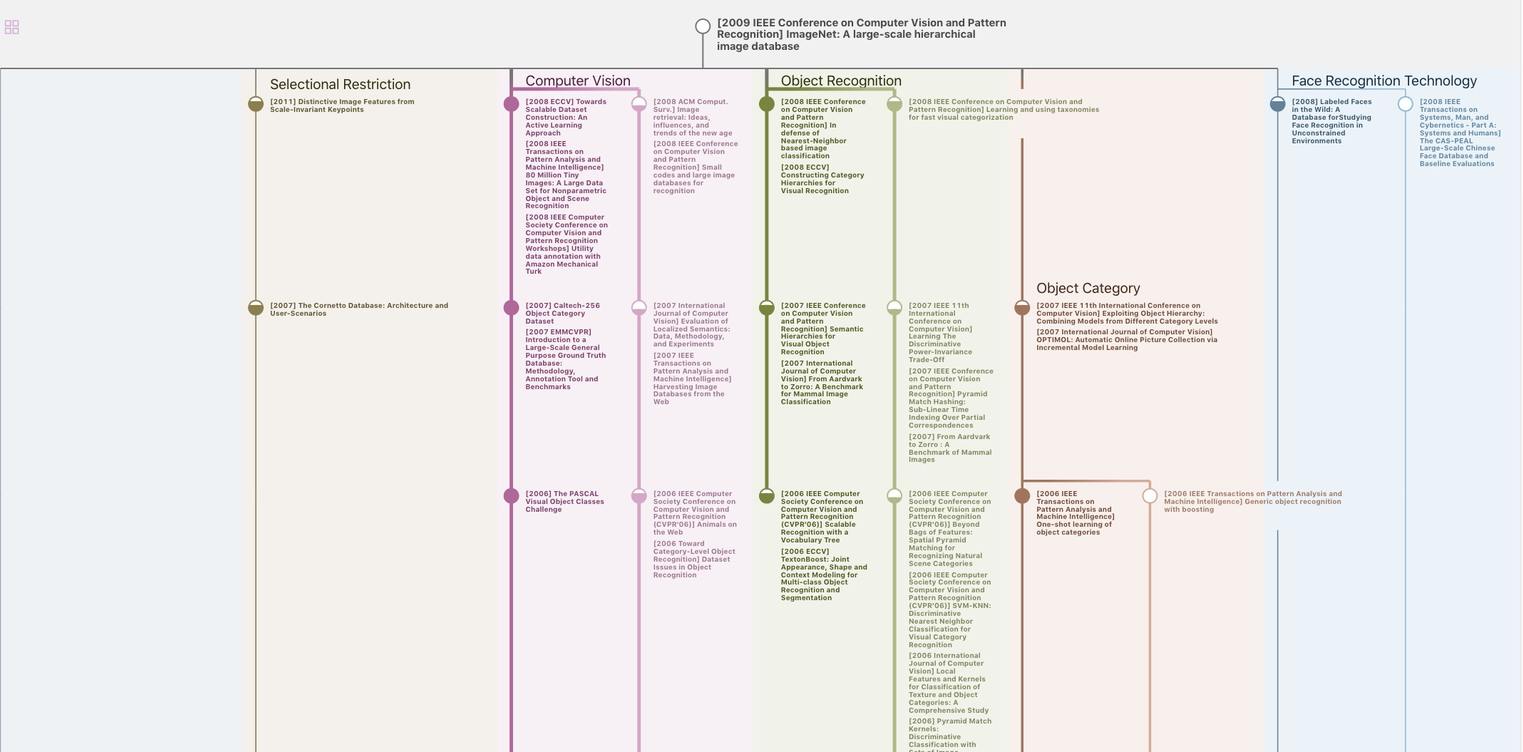
生成溯源树,研究论文发展脉络
Chat Paper
正在生成论文摘要