From IMU Measurement Sequence to Velocity Estimate Sequence: An Effective and Efficient Data-Driven Inertial Odometry Approach
IEEE Sensors Journal(2023)
摘要
We propose a novel deep-learning-based inertial odometry framework to improve both localization accuracy and efficiency. The measured 3-D accelerations and angular velocities are constructed as two independent channel 2-D features. In each channel, the three axes of accelerations or angular velocities constitute the height dimension, whereas the temporal axis represents the width dimension. In the neural network module, we first employ 2-D convolutional operations to convert the input features to 1-D series with fourfold downsampling along the temporal axis. We then apply the convolutional attention module to refine the generated 1-D feature map and long short-term memory (LSTM) layers to generate the hidden state for each refined time index, which is followed by two separate two-layer multilayer perceptrons (MLPs) to regress the velocity and the uncertainty. During backpropagation, we use the negative log-likelihood loss to optimize the velocity and the uncertainty objectives. Benefiting from the velocity sequence objective in a single network forward propagation procedure, we also add the average squared distance between the ground-truth relative position and that of the prediction to minimize the location error. Extensive experiments on both the robust neural inertial navigation (RoNIN) dataset and the real scenario collected data in the CUHK campus show higher localization accuracy with 10 times higher efficiency of the proposed framework than the comparing methods.
更多查看译文
关键词
Inertial measurement unit (IMU),inertial navigation,location awareness,pedestrian dead reckoning,velocity measurement
AI 理解论文
溯源树
样例
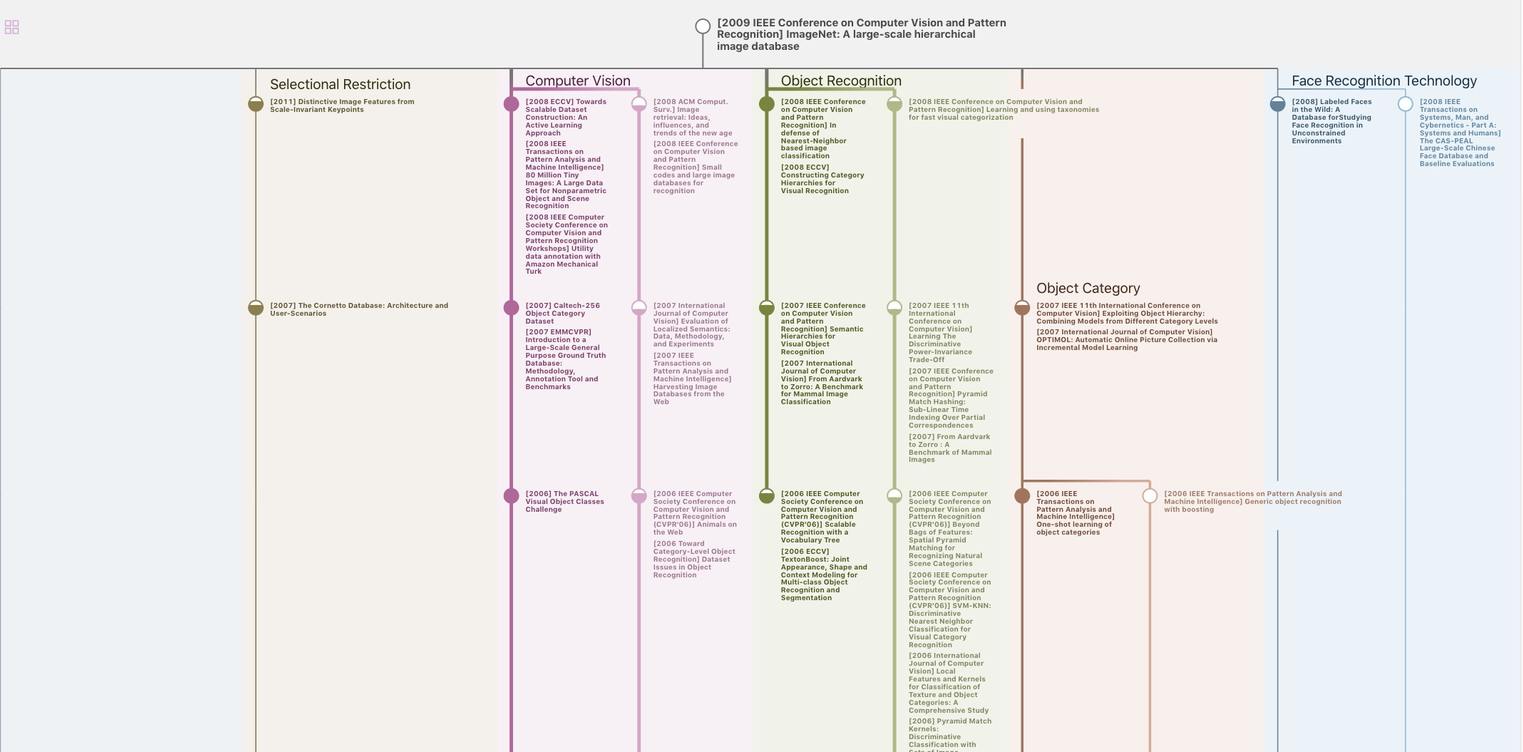
生成溯源树,研究论文发展脉络
Chat Paper
正在生成论文摘要