Electrocardiogram signal compression using adaptive tunable-Q wavelet transform and modified dead-zone quantizer
ISA TRANSACTIONS(2023)
摘要
The electrocardiogram (ECG) signals are commonly used to identify heart complications. These recordings generate large data that needed to be stored or transferred in telemedicine applications, which require more storage space and bandwidth. Therefore, a strong motivation is present to develop efficient compression algorithms for ECG signals. In the above context, this work proposes a novel compression algorithm using adaptive tunable-Q wavelet transform (TQWT) and modified dead-zone quantizer (DZQ). The parameters of TQWT and threshold values of DZQ are selected using the proposed Sparse-grey wolf optimization (Sparse-GWO) algorithm. The Sparse-GWO is proposed in this work to reduce the computation time of the original GWO. Moreover, it is also compared with some popular algorithms such as original GWO, particle swarm optimization (PSO), Hybrid PSOGWO, and SparsePSO. The DZQ has been utilized to perform thresholding and quantization. Then, run-length encoding (RLE) has been used to encode the quantized coefficients. The proposed work has been performed on the MIT-BIH arrhythmia database. Quality assessment performed on reconstructed signals ensure the minimal impact of compression on the morphology of reconstructed ECG signals. The compression performance of proposed algorithm is measured in terms of the following evaluation matrices: percent root-mean-square difference (PRD1), compression ratio (CR), signal-to-noise ratio (SNR), and quality score (QS1). The obtained average values are 3.21%, 20.56, 30.62 dB, and 7.79, respectively.(c) 2023 ISA. Published by Elsevier Ltd. All rights reserved.
更多查看译文
关键词
ECG signal compression,Adaptive tunable-Q wavelet transform,Dead-zone quantizer,Run-length encoding,Sparse-GWO,Optimization algorithms
AI 理解论文
溯源树
样例
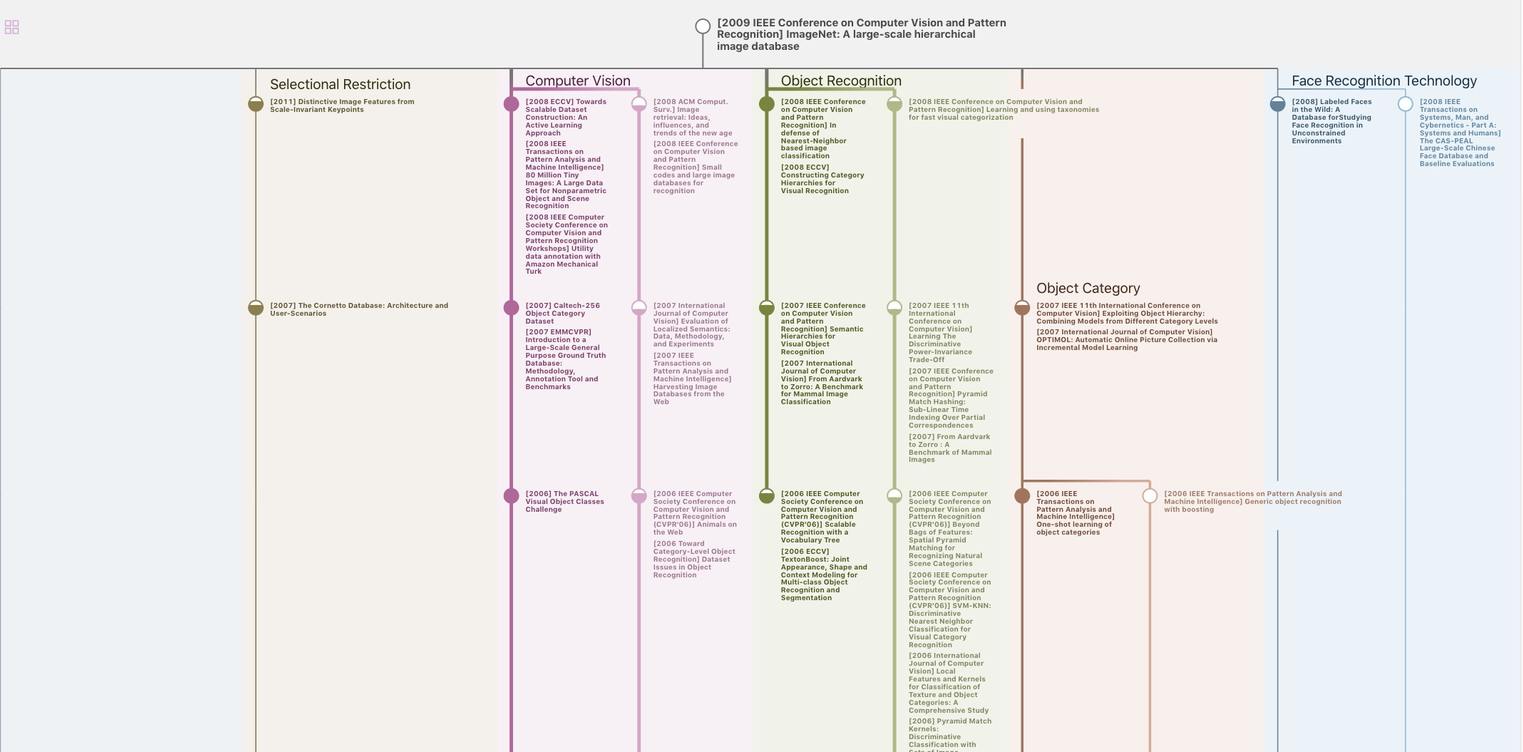
生成溯源树,研究论文发展脉络
Chat Paper
正在生成论文摘要