Painting baryons onto N-body simulations of galaxy clusters with image-to-image deep learning
arXiv (Cornell University)(2023)
摘要
Galaxy cluster mass functions are a function of cosmology, but mass is not a direct observable, and systematic errors abound in all its observable proxies. Mass-free inference can bypass this challenge, but it requires large suites of simulations spanning a range of cosmologies and models for directly observable quantities. In this work, we devise a U-net - an image-to-image machine learning algorithm - to ``paint'' the IllustrisTNG model of baryons onto dark-matter-only simulations of galaxy clusters. Using 761 galaxy clusters with $M_{200c} \gtrsim 10^{14}M_\odot$ from the TNG-300 simulation at $z<1$, we train the algorithm to read in maps of projected dark matter mass and output maps of projected gas density, temperature, and X-ray flux. The models train in under an hour on two GPUs, and then predict baryonic images for $\sim2700$ dark matter maps drawn from the TNG-300 dark-matter-only (DMO) simulation in under two minutes. Despite being trained on individual images, the model reproduces the true scaling relation and scatter for the $M_{DM}-L_X$, as well as the distribution functions of the cluster X-ray luminosity and gas mass. For just one decade in cluster mass, the model reproduces three orders of magnitude in $L_X$. The model is biased slightly high when using dark matter maps from the DMO simulation, which is known to have ultra-dense clumps that in the FP simulation are smoothed out by baryonic feedback. The model performs well on inputs from TNG-300-2, whose mass resolution is 8 times coarser; further degrading the resolution biases the predicted luminosity function high. We conclude that U-net-based baryon painting is a promising technique to build large simulated cluster catalogs which can be used to improve cluster cosmology by combining existing full-physics and large $N$-body simulations.
更多查看译文
关键词
galaxy clusters,baryons,simulations,image-to-image
AI 理解论文
溯源树
样例
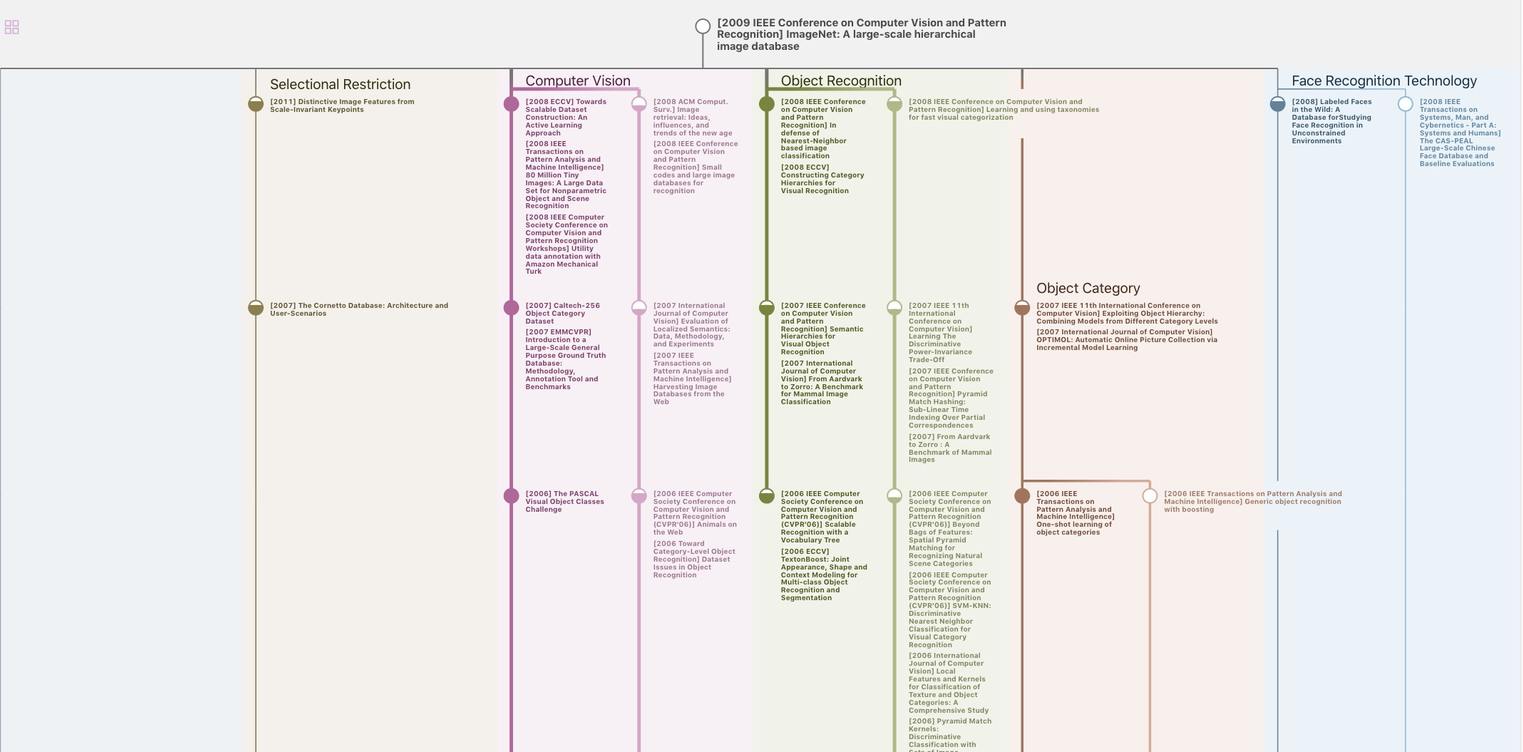
生成溯源树,研究论文发展脉络
Chat Paper
正在生成论文摘要