MTD-GPT: A Multi-Task Decision-Making GPT Model for Autonomous Driving at Unsignalized Intersections
CoRR(2023)
摘要
Autonomous driving technology is poised to transform transportation systems. However, achieving safe and accurate multi-task decision-making in complex scenarios, such as unsignalized intersections, remains a challenge for autonomous vehicles. This paper presents a novel approach to this issue with the development of a Multi-Task Decision-Making Generative Pre-trained Transformer (MTD-GPT) model. Leveraging the inherent strengths of reinforcement learning (RL) and the sophisticated sequence modeling capabilities of the Generative Pre-trained Transformer (GPT), the MTD-GPT model is designed to simultaneously manage multiple driving tasks, such as left turns, straight-ahead driving, and right turns at unsignalized intersections. We initially train a single-task RL expert model, sample expert data in the environment, and subsequently utilize a mixed multi-task dataset for offline GPT training. This approach abstracts the multi-task decision-making problem in autonomous driving as a sequence modeling task. The MTD-GPT model is trained and evaluated across several decision-making tasks, demonstrating performance that is either superior or comparable to that of state-of-the-art single-task decision-making models.
更多查看译文
关键词
mtd-gpt model,autonomous driving
AI 理解论文
溯源树
样例
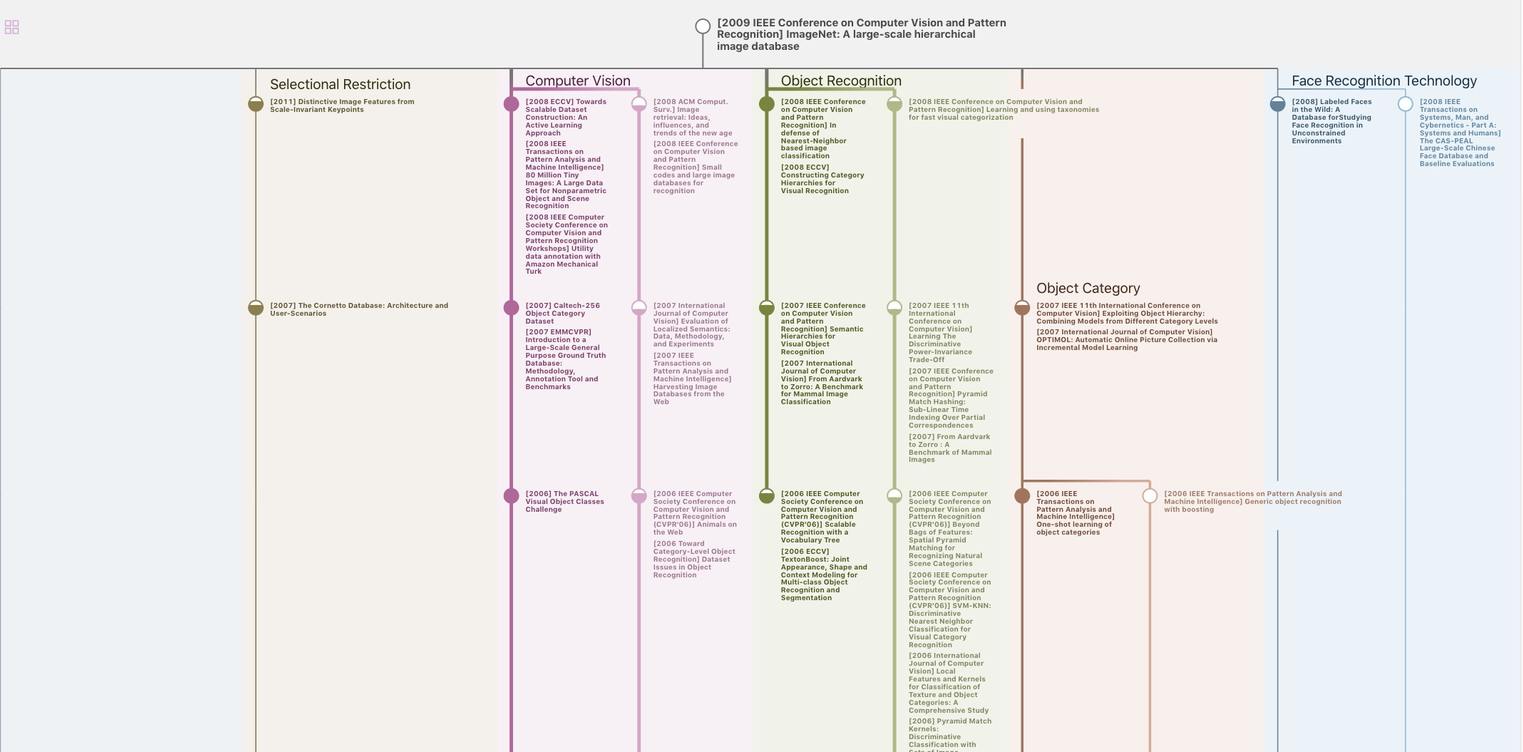
生成溯源树,研究论文发展脉络
Chat Paper
正在生成论文摘要