RGB-D-Fusion: Image Conditioned Depth Diffusion of Humanoid Subjects
IEEE ACCESS(2023)
摘要
We present RGB-D-Fusion, a multi-modal conditional denoising diffusion probabilistic model to generate high resolution depth maps from low-resolution monocular RGB images of humanoid subjects. Accurately representing the human body in 3D is a very active research field given its wide variety of applications. Most 3D reconstruction algorithms rely on depth maps, either coming from low-resolution consumer-level depth sensors, or from monocular depth estimation from standard images. While many modern frameworks use VAEs or GANs for monocular depth estimation, we leverage recent advances in the field of diffusion denoising probabilistic models. We implement a multi-stage conditional diffusion model that first generates a low-resolution depth map conditioned on an image and then upsamples the depth map conditioned on a low-resolution RGB-D image. We further introduce a novel augmentation technique, depth noise augmentation, to increase the robustness of our super-resolution model. Lastly, we show how our method performs on a wide variety of humans with different body types, clothing and poses.
更多查看译文
关键词
Diffusion models,generative deep learning,monocular depth estimation,depth super-resolution,multi-modal,augmented-reality,virtual-reality
AI 理解论文
溯源树
样例
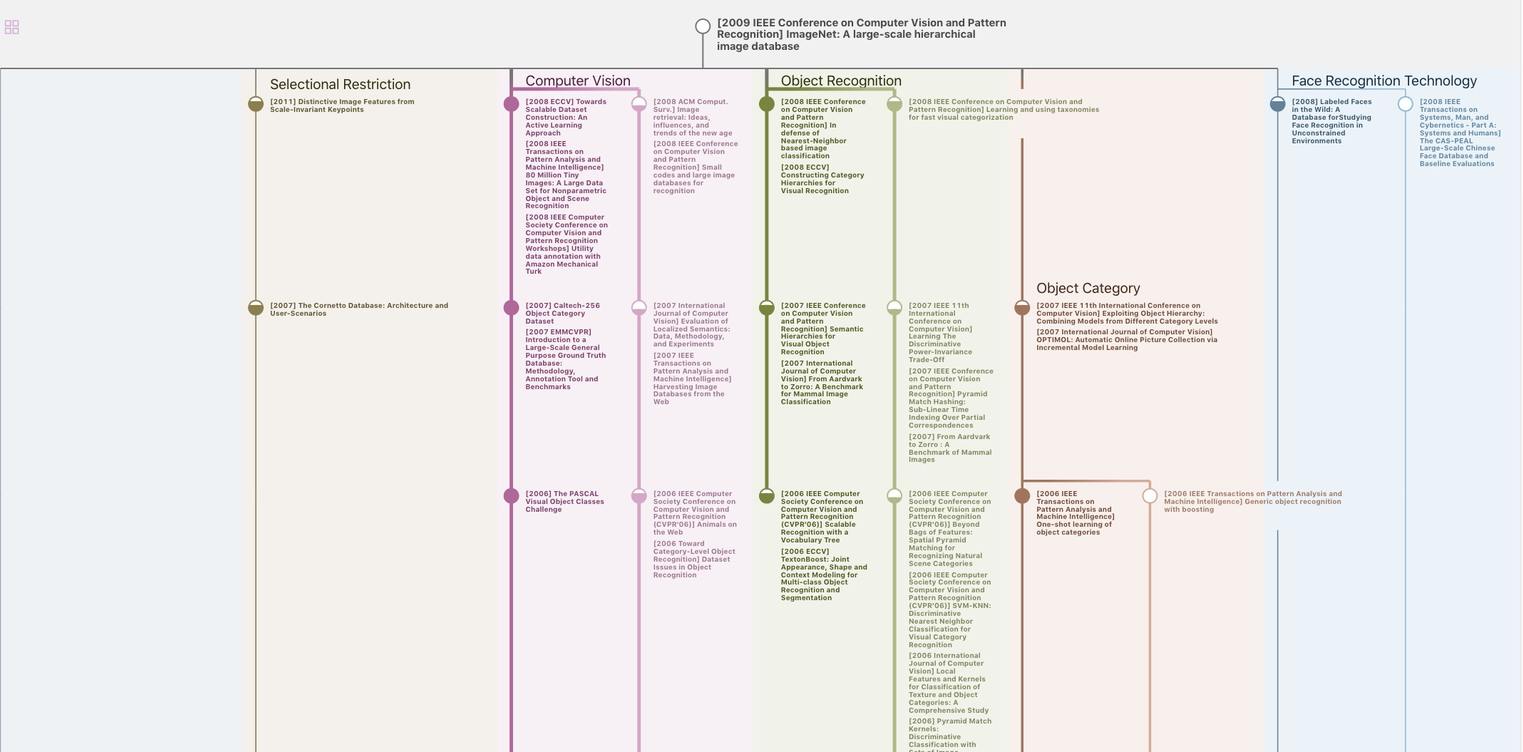
生成溯源树,研究论文发展脉络
Chat Paper
正在生成论文摘要