Improving Realistic Worst-Case Performance of NVCiM DNN Accelerators through Training with Right-Censored Gaussian Noise
2023 IEEE/ACM INTERNATIONAL CONFERENCE ON COMPUTER AIDED DESIGN, ICCAD(2023)
摘要
Compute-in-Memory (CiM), built upon non-volatile memory (NVM) devices, is promising for accelerating deep neural networks (DNNs) owing to its in-situ data processing capability and superior energy efficiency. To battle device variations, noise injection training is commonly used, which perturbs weights with Gaussian noise during training to make the model more robust to weight variations. Despite its prevalence, however, existing successes are mostly empirical, and very little theoretical support is available. Even the most fundamental questions such as why Gaussian but not other types of noises should be used is not answered. In this work, through formally analyzing the effect of injecting Gaussian noise in training to improve the k-th percentile performance (KPP), a realistic worst-case performance metric, for the first time we provide a theoretical justification of the effectiveness of the approach. We further show that surprisingly Gaussian noise is not the best option, contrary to what has been taken for granted in the literature. Instead, a right-censored Gaussian noise significantly improves the KPP of DNNs. We further propose an automated method to determine the optimal hyperparameters for injecting this right-censored Gaussian noise during the training process. Our method achieves up to a 26% improvement in KPP compared to the state-of-the-art methods employed to enhance DNN robustness under the impact of device variations.
更多查看译文
AI 理解论文
溯源树
样例
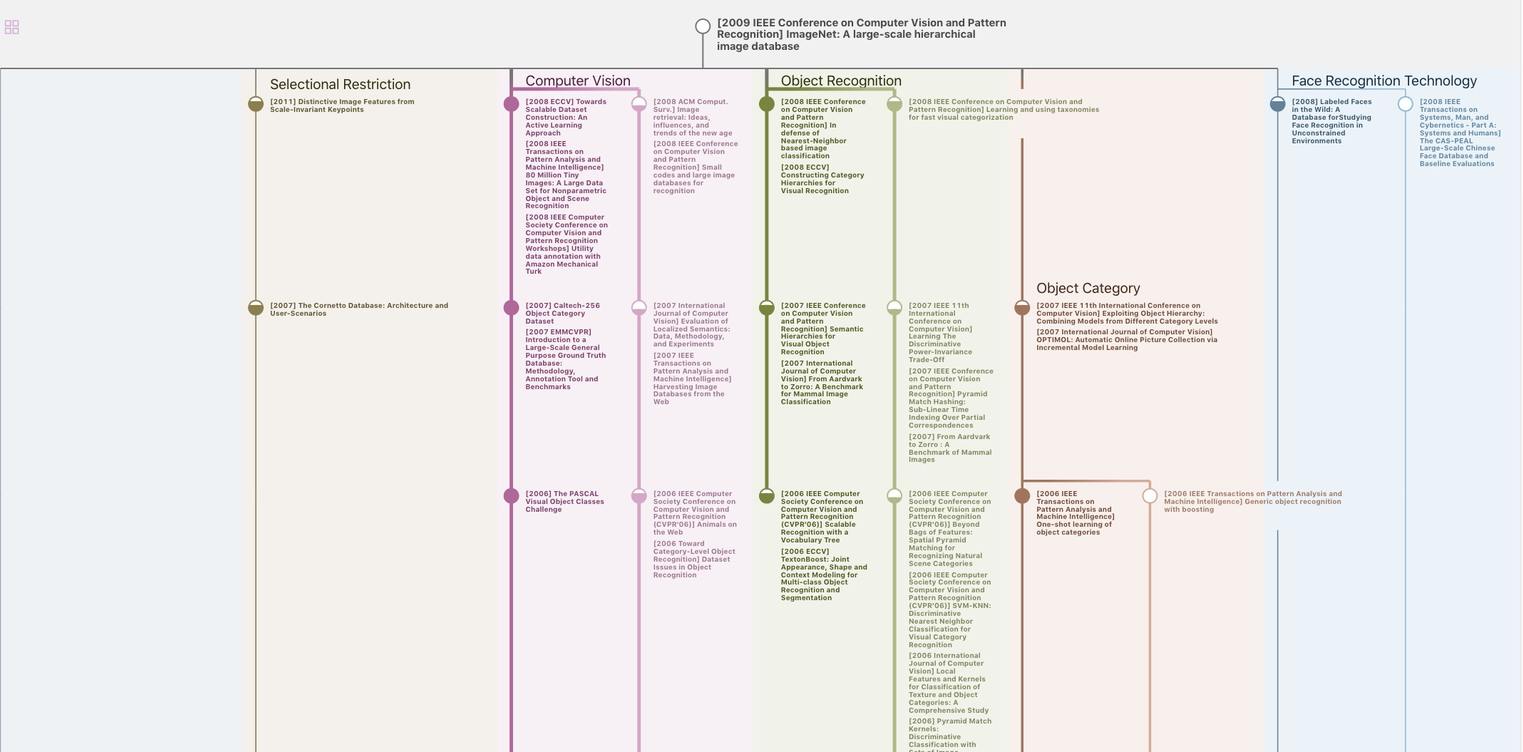
生成溯源树,研究论文发展脉络
Chat Paper
正在生成论文摘要