Automated Feature Document Review via Interpretable Deep Learning.
ICSE Companion(2023)
摘要
A feature in the agile methodology is a function of a product that delivers business value and meets stakeholders' requirements. Developers compile and store the content of features in a structured feature document. Feature documents play a critical role in controlling software development at a macro level. It is therefore important to ensure the quality of feature documents so that defects are not introduced at the outset. Manual review is an effective activity to ensure quality, but it is human-intensive and challenging. In this paper, we propose a feature document review tool to automate the process of manual review (quality classification, and suggestion generation) based on neural networks and interpretable deep learning. Our goal is to reduce human effort in reviewing feature documents and to prompt authors to craft better feature documents. We have evaluated our tool on a real industrial project from ZTE Corporation. The results show that our quality classification model achieved 75.6% precision and 94.4% recall for poor quality feature documents. For the suggestion generation model, about 70% of the poor quality feature documents could be improved to the qualified level in three rounds of revision based on the suggestions. User feedback shows that our tool helps users save an average of 15.9% of their time.
更多查看译文
关键词
Feature Documents, Agile Methodology, Neural Networks, Interpretable Deep Learning
AI 理解论文
溯源树
样例
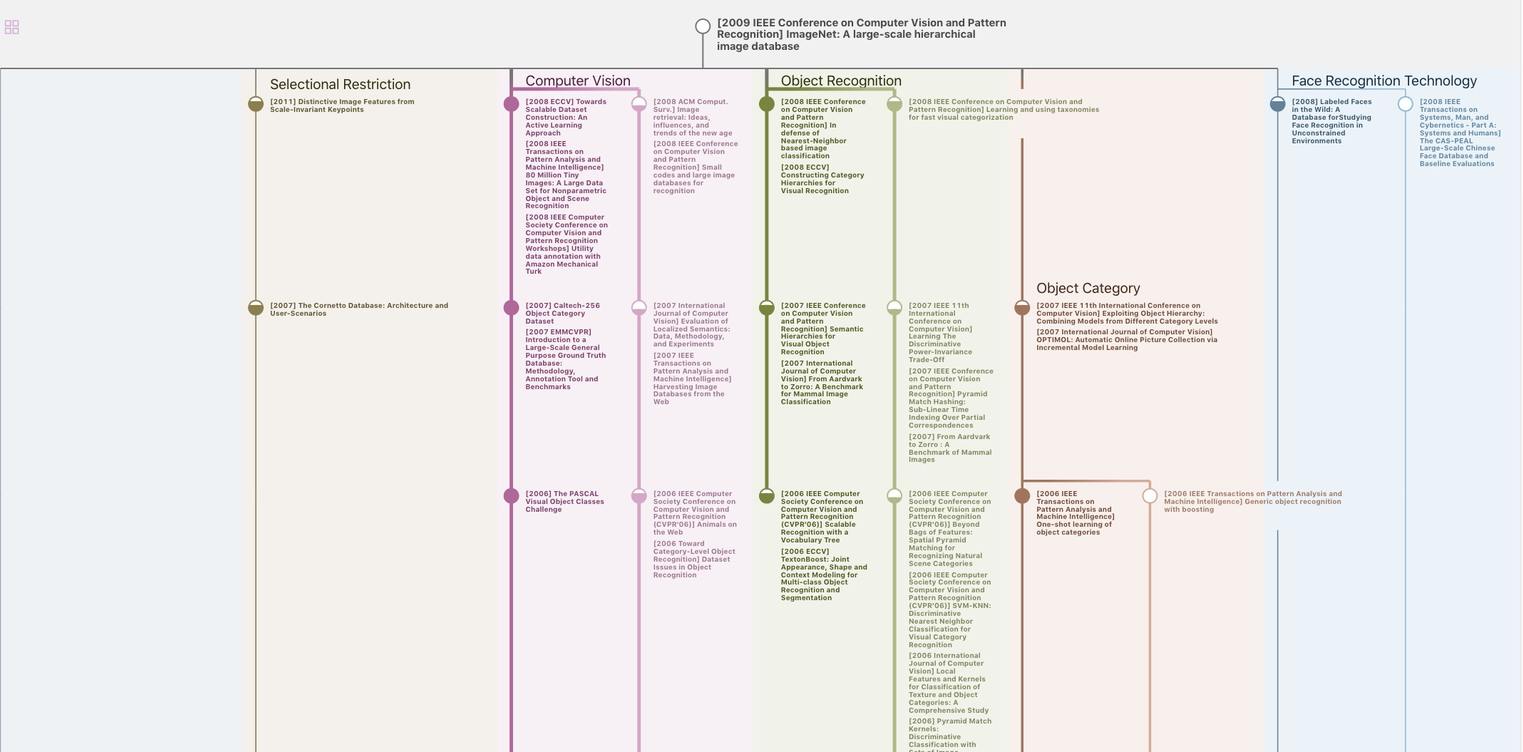
生成溯源树,研究论文发展脉络
Chat Paper
正在生成论文摘要