Correlated Cluster-Based Randomized Experiments: Robust Variance Minimization.
EC(2023)
摘要
Experimentation is prevalent in online marketplaces and social networks to assess the effectiveness of new market intervention. To mitigate the interference among users in an experiment, a common practice is to use a cluster-based experiment, where the designer partitions the market into loosely connected clusters and assigns all users in the same cluster to the same variant (treatment or control). Given the experiment, we assume an unbiased Horvitz-Thompson estimator is used to estimate the total market effect of the treatment. We consider the optimization problem of choosing (correlated) randomized assignments of clusters to treatment and control to minimize the worst-case variance of the estimator under a constraint that the marginal assignment probability is q & ISIN; (0,1) for all clusters. This problem can be formulated as a linear program where both the number of decision variables and constraints are exponential in the number of clusters-and hence is generally computationally intractable. We develop a family of practical experiments that we refer to as independent block randomization (IBR) experiments. Such an experiment partitions clusters into blocks so that each block contains clusters of similar size. It then treats a fraction q of the clusters in each block (chosen uniformly at random) and does so independently across blocks. The optimal cluster partition can be obtained in a tractable way using dynamic programming. We show that these policies are asymptotically optimal when the number of clusters grows large and no cluster size dominates the rest. In the special case where cluster sizes take values in a finite set and the number of clusters of each size is a fixed proportion of the total number of clusters, the loss is only a constant that is independent of the number of clusters. Beyond the asymptotic regime, we show that the IBR experiment has a good approximation for any problem instance when q is not very tiny. We also examine the performance of the IBR experiments on data-driven numerical examples, including examples based on Airbnb and Facebook data.
更多查看译文
关键词
robust variance minimization,randomized experiments,cluster-based
AI 理解论文
溯源树
样例
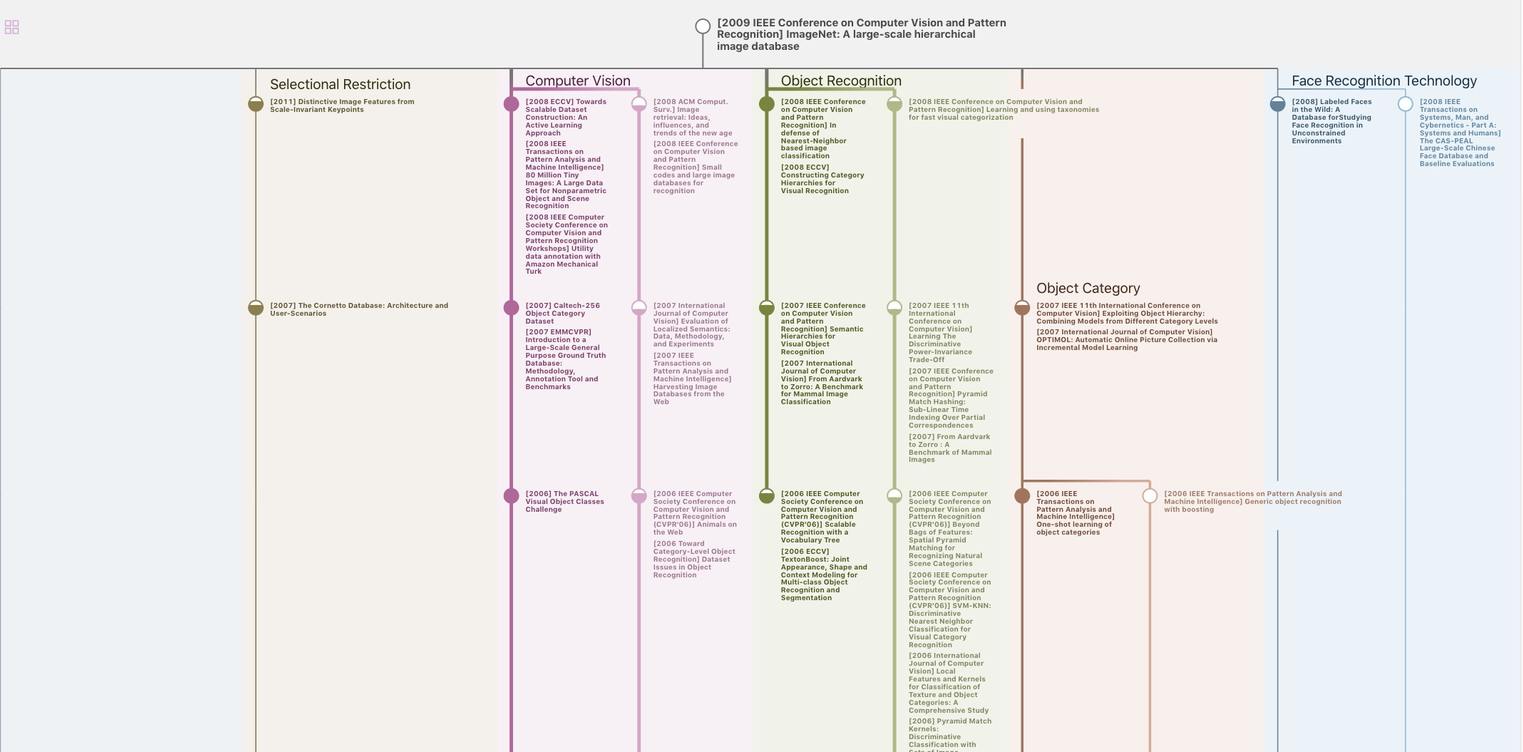
生成溯源树,研究论文发展脉络
Chat Paper
正在生成论文摘要