Semi-supervised Time Series Classification Through Image Representations.
ICCSA (2)(2023)
摘要
Time series data is of crucial importance in different domains, such as financial and medical applications. However, obtaining a large amount of labeled time series data is an expensive and time-consuming task, which becomes the process of building an effective machine learning model a challenge. In these scenarios, algorithms that can deal with reduced amounts of labeled data emerge. One example is Semi-Supervised Learning (SSL), which has the capability of exploring both labeled and unlabeled data for tasks such as classification. In this work, a kNN graph-based transductive SSL approach is used for time series classification. A feature extraction step, based on imaging time series and obtaining features using deep neural networks is performed before the classification step. An extensive evaluation is conducted over four datasets, and a parametric analysis of the nearest neighbors is performed. Also, a statistical analysis over the obtained distances is conducted. Results suggest that our methods are suitable for classification and competitive with supervised baselines in some datasets.
更多查看译文
关键词
classification,representations,semi-supervised
AI 理解论文
溯源树
样例
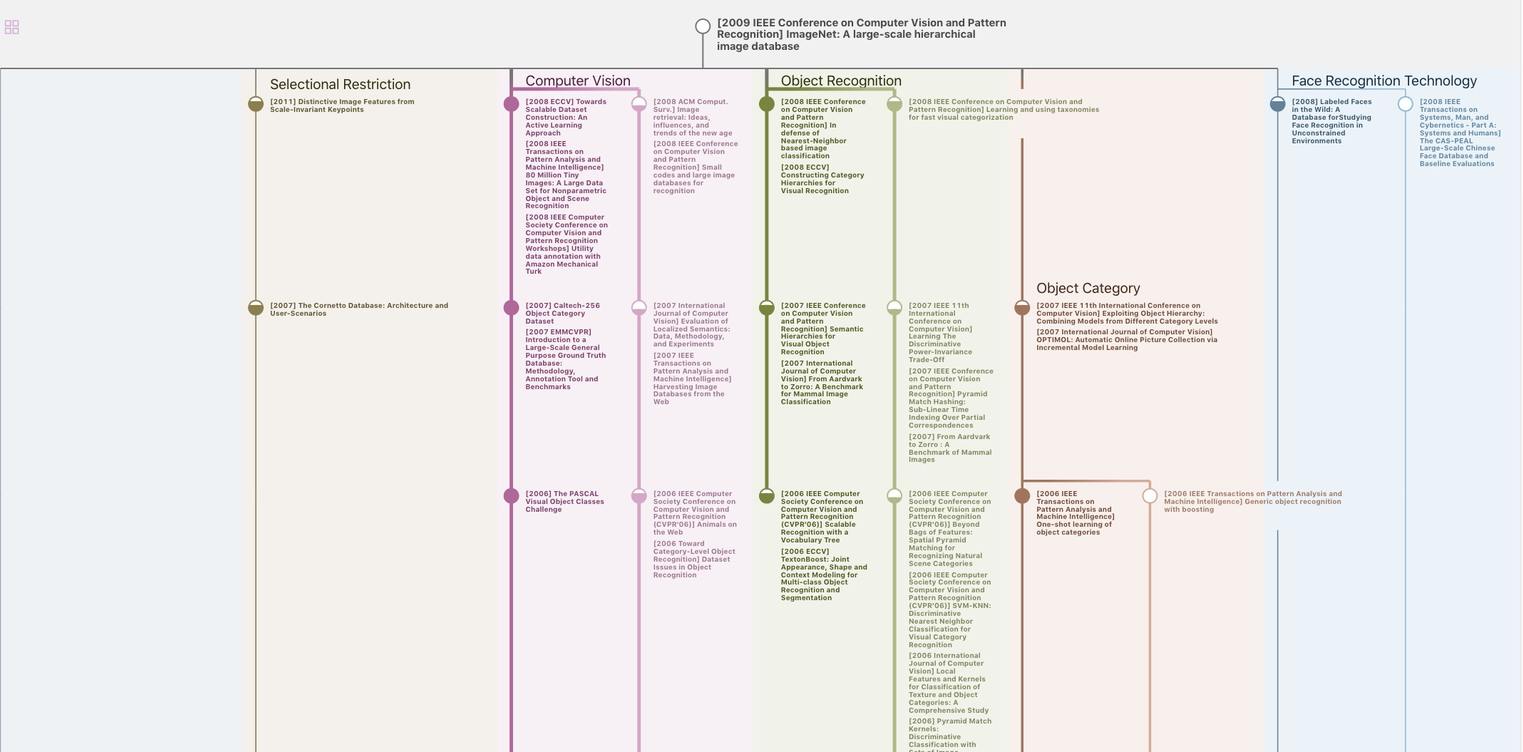
生成溯源树,研究论文发展脉络
Chat Paper
正在生成论文摘要