Dynamic Machine Learning Algorithm Selection For Network Slicing in Beyond 5G Networks.
NetSoft(2023)
摘要
The advanced 5G and 6G mobile network generations offer new capabilities that enable the creation of multiple virtual network instances with distinct and stringent requirements. However, the coexistence of multiple network functions on top of a shared substrate network poses a resource allocation challenge known as the Virtual Network Embedding (VNE) problem. In recent years, this NP-hard problem has received increasing attention in the literature due to the growing need to optimize resources at the edge of the network, where computational and storage capabilities are limited. In this demo paper, we propose a solution to this problem, utilizing the Algorithm Selection (AS) paradigm. This selects the most optimal Deep Reinforcement Learning (DRL) algorithm from a portfolio of agents, in an offline manner, based on past performance. To evaluate our solution, we developed a simulation platform using the OMNeT++ framework, with an orchestration module containerized using Docker. The proposed solution shows good performance and outperforms standalone algorithms.
更多查看译文
关键词
Network Slicing,Virtual Network Embedding,Deep Reinforcement Learning,Algorithm Selection,Containerized Networks
AI 理解论文
溯源树
样例
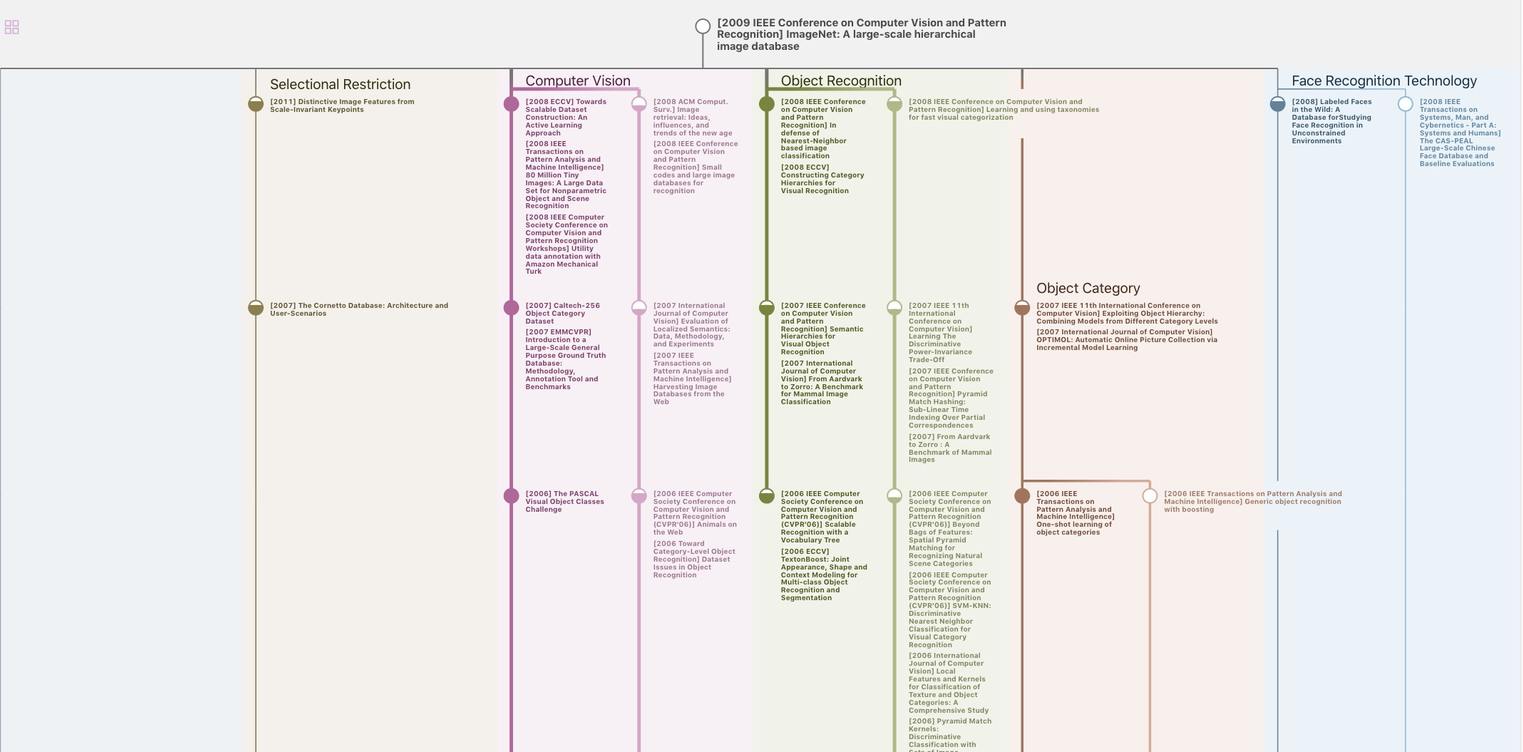
生成溯源树,研究论文发展脉络
Chat Paper
正在生成论文摘要