Graph Contrastive Learning with Intrinsic Augmentations.
BIC-TA(2022)
摘要
Graph contrastive learning has become an important approach for learning unsupervised representations of graphs, with the key idea of maximizing the consistency of representations in both augmented views through data augmentation. Existing graph contrastive learning models concentrate on topology enhancement of the graph structure by simply removing/adding edges between nodes randomly, which may not only destroy the important structure of the graph but also generate meaningless graphs, leading to a significant degradation of the contrastive learning performance. In addition, current research is too focused on minimizing losses, while ignoring the intrinsic factors that can affect the quality of node representations, which is detrimental to the training of models and the generation of high-quality node representations. To address these issues, we propose intrinsic augmented graph contrastive learning, named IAG, which consists of two components: 1) In the topology augmentation part, we propose a novel topology augmentation strategy based on potential connections in the feature space, which complements the traditional topology augmentation by allowing different graphs to obtain augmentation strategies more suitable for their own characteristics to advance the traditional graph contrastive learning. 2) We explored the effect of temperature coefficient in the loss function on the quality of the final representation and proposed dynamic temperature with penalty terms, which helps to generate high-quality node representations. Finally, we conducted extensive node classification experiments on 8 real-world datasets. The experimental results show that our proposed method is highly competitive with the existing state-of-the-art baselines and even surpasses some supervised methods.
更多查看译文
关键词
graph contrastive learning
AI 理解论文
溯源树
样例
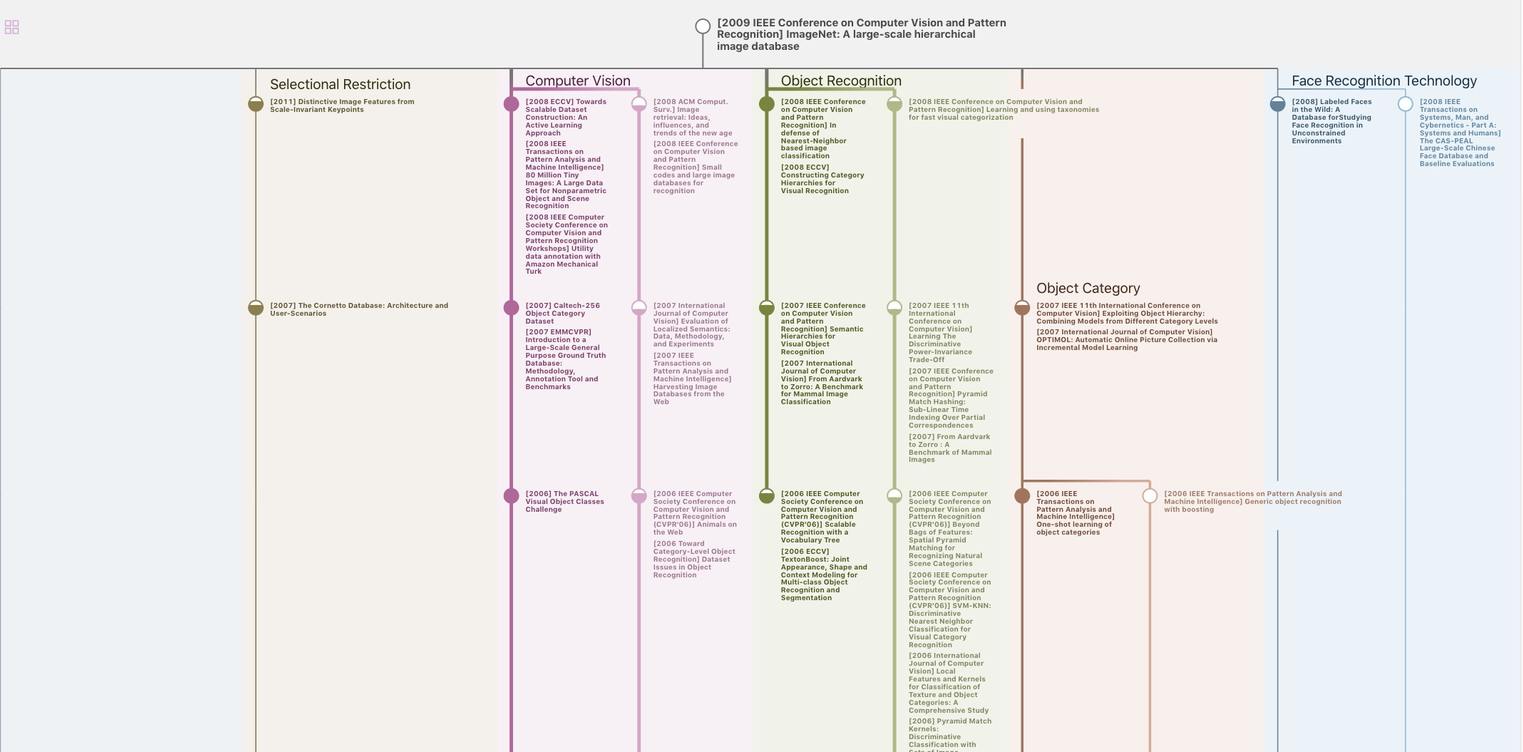
生成溯源树,研究论文发展脉络
Chat Paper
正在生成论文摘要