Effect of incorporating metadata to the generation of synthetic time series in a healthcare context
2023 IEEE 36TH INTERNATIONAL SYMPOSIUM ON COMPUTER-BASED MEDICAL SYSTEMS, CBMS(2023)
摘要
Syntheticdata is becoming the way forward to manage legal and regulatory aspects of biomedical research involving personal and clinical data. As no matches are expected between artificial instances and real samples and/or subjects, external researchers performing secondary analyses could benefit significantly by having unlimited access to uncompromised information. In this context, one of the main objectives of the 112020 VITALISE project is to develop a platform for providing synthetic data generated from real data collected in Living Labs to those external researchers. in addition, while some time series specific synthetic data generation models exist, only a few of them consider metadata (e.g., patient demographics) as part of the time series generation process itself. Therefore, the objective of this research is to perform a comparative assessment of two synthetic data generation models that use and process the metadata of subjects differently: The Wasserstein CAN with Gradient Penalty (WGAN-GP) and the DOppelGANger (DGAN). To achieve this goal making sure the analyses were data-independent, we selected two healthcare -related longitudinal datasets: (1) Treadmill Maximal Effort Test (TMET) measurements from the University of Malaga; and (2) a hypotension subset derived from the MIMIC -Ill v1.4 database. After synthetic data was generated, we assessed three pivotal aspects: resemblance to the original data, utility, and level of privacy. As a main conclusion, the importance of using metadata as context variables and the methodology to take them into account was proved to be significant and valuable, the DEAN model offering better results overall.A more extensive time series specific evaluation is left as the main avenuefor future research.
更多查看译文
关键词
time series,synthetic data,shareable data,health data.
AI 理解论文
溯源树
样例
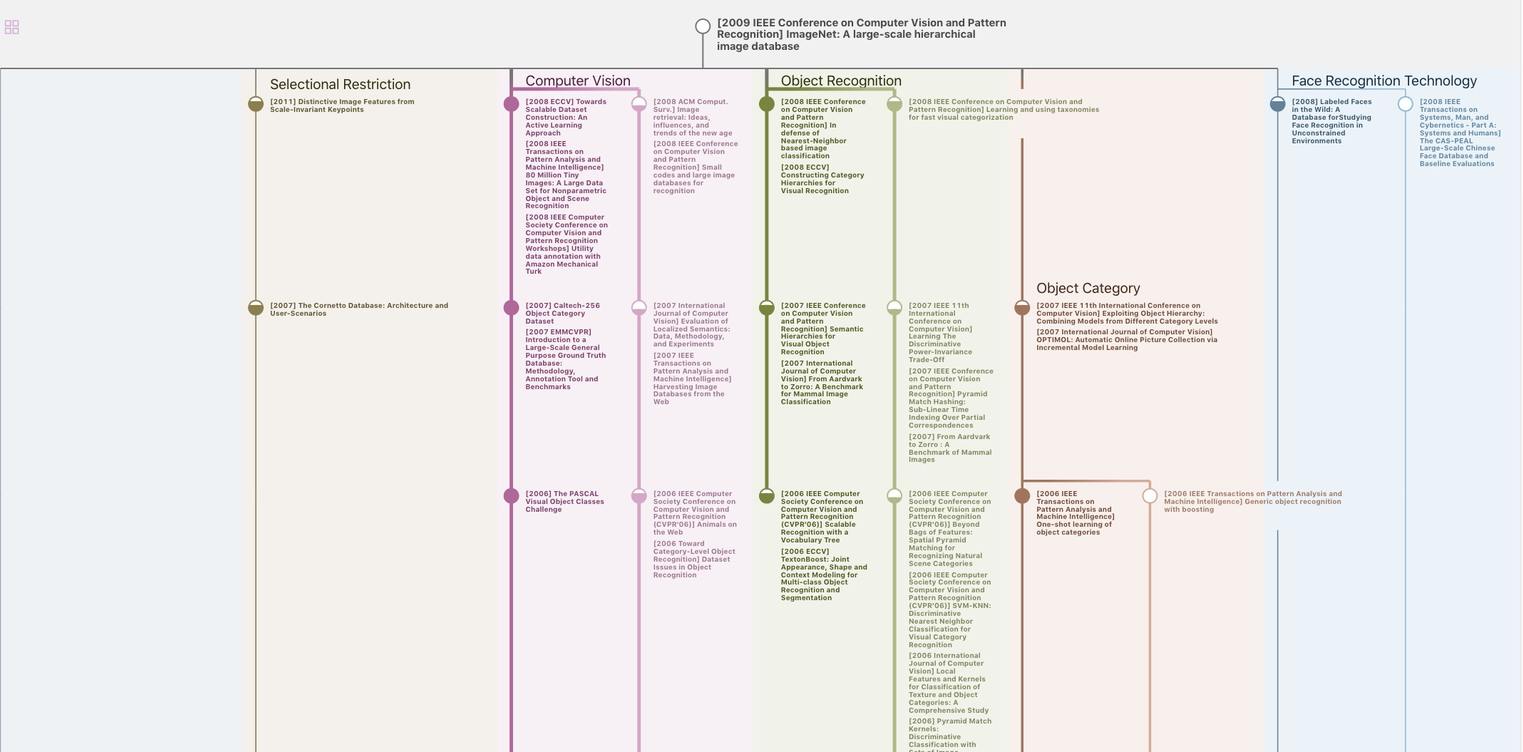
生成溯源树,研究论文发展脉络
Chat Paper
正在生成论文摘要