A Feature-Driven Fixed-Ratio Lossy Compression Framework for Real-World Scientific Datasets.
ICDE(2023)
摘要
Today’s scientific applications and advanced instruments are producing extremely large volumes of data everyday, so that error-controlled lossy compression has become a critical technique to the scientific data storage and management. Existing lossy scientific data compressors, however, are designed mainly based on error-control driven mechanism, which cannot be efficiently applied in the fixed-ratio use-case, where a desired compression ratio needs to be reached because of the restricted data processing/management resources such as limited memory/storage capacity and network bandwidth. To address this gap, we propose a low-cost compressor-agnostic feature-driven fixed-ratio lossy compression framework (FXRZ). The key contributions are three-fold. (1) We perform an in-depth analysis of the correlation between diverse data features and compression ratios based on a wide range of application datasets, which is a fundamental work for our framework. (2) We propose a series of optimization strategies that can enable the framework to reach a fairly high accuracy in identifying the expected error configuration with very low computational cost. (3) We comprehensively evaluate our framework using 4 state-of-the-art error-controlled lossy compressors on 10 different snapshots and simulation configuration-based real-world scientific datasets from 4 different applications across different domains. Our experiment shows that FXRZ outperforms the state-of-the-art related work by 108×. The experiments with 4,096 cores on a supercomputer show a performance gain of 1.18∼8.71× than the related work in overall parallel data dumping.
更多查看译文
关键词
Lossy Compression,Scientific Data Management,Machine Learning,Data Features
AI 理解论文
溯源树
样例
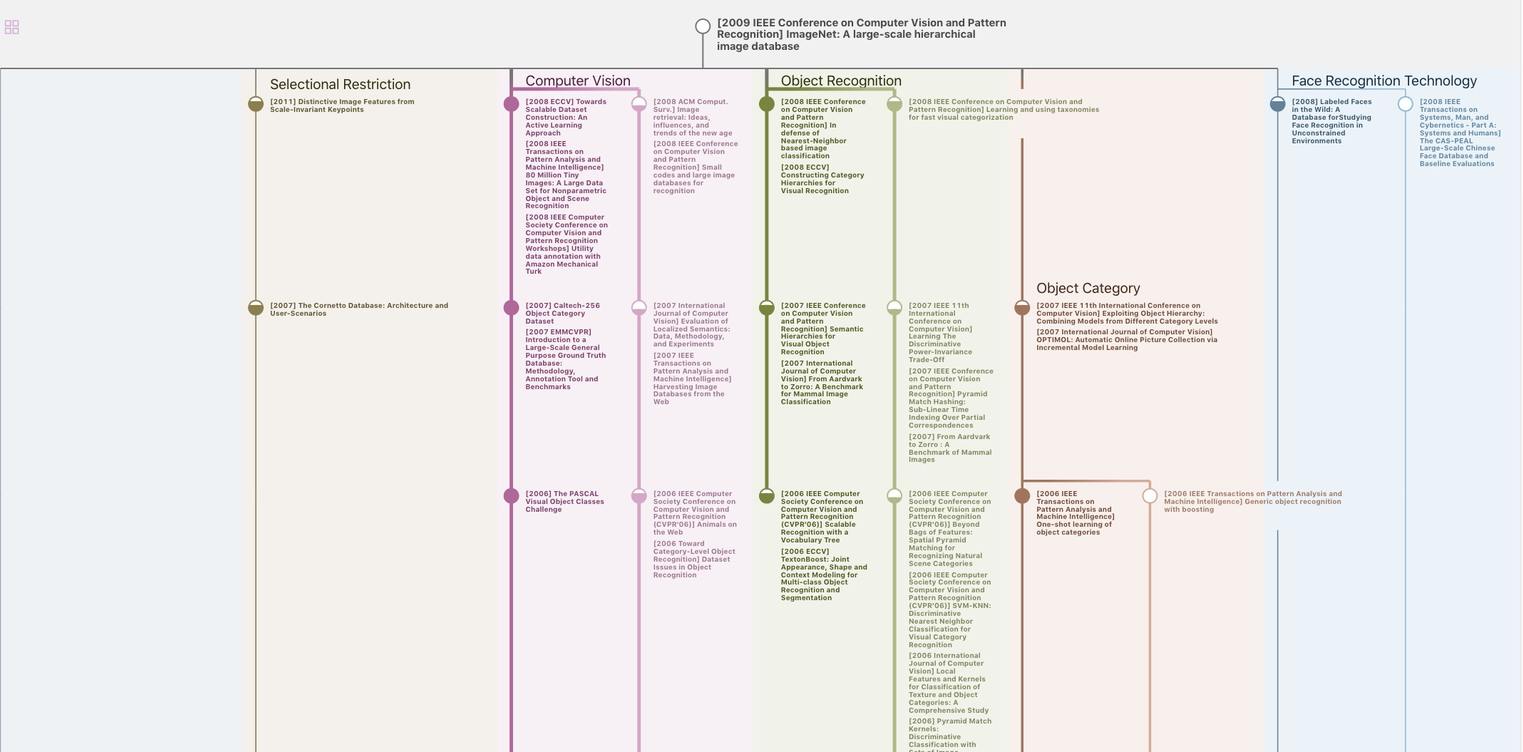
生成溯源树,研究论文发展脉络
Chat Paper
正在生成论文摘要