Efficiently Learning Spatial Indices.
ICDE(2023)
摘要
Learned indices can leverage the high prediction accuracy and efficiency of modern deep learning techniques. They are capable of delivering better query performance than traditional indices over one-dimensional data. Recent studies demonstrate that we can also achieve query-efficient learned in-dices for spatial data by partitioning and subsequently transforming spatial data to one-dimensional values, after which existing techniques can be applied. While enabling efficient querying, building and rebuilding learned spatial indices efficiently remains largely unaddressed. As the model training needed to learn a spatial index is costly, efficient building and rebuilding of learned spatial indices on large data sets is challenging if performed by means of model training and retraining.To advance the practicality of learned spatial indices, we propose a system named ELSI that enables the efficient building and rebuilding of a class of learned spatial indices that follow two simple design principles. The core idea is to reduce the model (re-)building times by engineering reduced training sets that preserve key data distribution patterns. ELSI encompasses a suite of methods for constructing small and distribution-preserving training sets from input data sets. Further, given an input data set, ELSI can adaptively select a method that produces a learned index with high query efficiency. Experiments on real data sets of 100+ million points show that ELSI can reduce the build times of four different learned spatial indices consistently (by up to two orders of magnitude) without jeopardizing query efficiency.
更多查看译文
关键词
learned spatial indices,index building
AI 理解论文
溯源树
样例
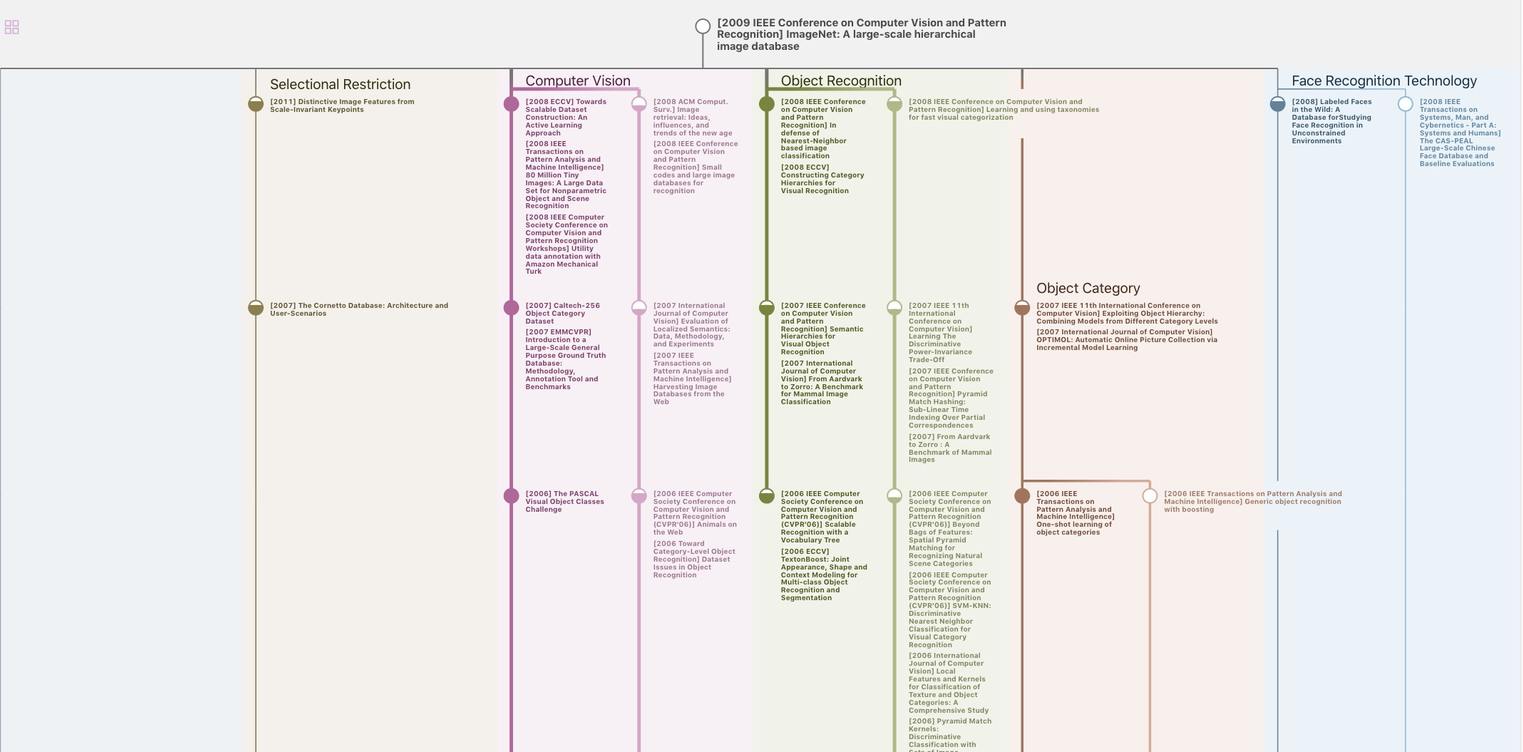
生成溯源树,研究论文发展脉络
Chat Paper
正在生成论文摘要