Noise amplifiation of momentum-based optimization algorithms
2023 AMERICAN CONTROL CONFERENCE, ACC(2023)
摘要
We study momentum-based first-order optimization algorithms in which the iterations utilize information from the two previous steps and are subject to additive white noise. For strongly convex quadratic problems, we utilize Jury stability criterion to provide a novel geometric characterization of linear convergence and exploit this insight to derive alternative proofs of standard convergence results and identify fundamental performance tradeoffs. We use the steady-state variance of the error in the optimization variable to quantify noise amplification and establish analytical lower bounds on the product between the settling time and the smallest/largest achievable noise amplification that scale quadratically with the condition number. This extends the prior work [1], where only the special cases of Polyak's heavy-ball and Nesterov's accelerated algorithms were studied. We also use this geometric characterization to introduce a parameterized family of algorithms that strikes a balance between noise amplification and settling time while preserving order-wise Pareto optimality.
更多查看译文
关键词
First-order methods,convergence rate,convex optimization,heavy-ball method,noise amplification,Nesterov's accelerated algorithm,performance tradeoffs,settling time
AI 理解论文
溯源树
样例
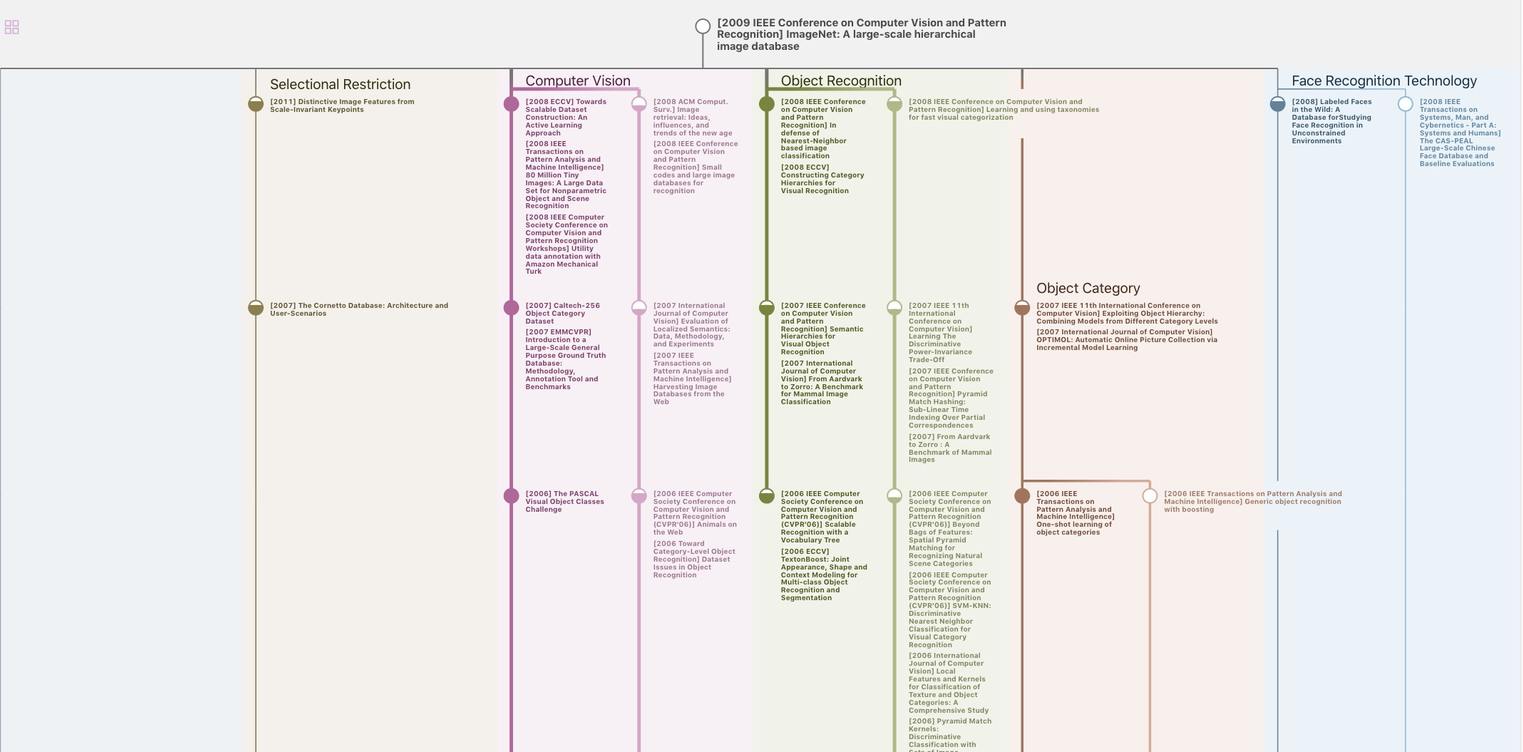
生成溯源树,研究论文发展脉络
Chat Paper
正在生成论文摘要