Challenges and constraints when applying few shot learning to a real-world scenario: In-the-wild camera-trap species classification.
Imaging and Multimedia Analytics at the Edge(2023)
摘要
Few shot learning (FSL) describes the challenge of learning to classify when there are only few labeled examples. The goal is to be able to adapt to a new classification task using a minimum amount of new data. However, when applying FSL to real-world problems, a number of constraints and challenges are encountered: model selection, training data selection, hyper-parameter and cost function, and final decision making. In this paper, we consider a realistic problem that fits perfectly with the narratives of FSL: our goal is to classify animal species that appear in our in-the-wild camera traps located in Senegal, when these species have yet to appear in popular animal datasets. Using the philosophy of FSL, we first train a FSL network to learn to separate animal species, using popular, large animal datasets, and then evaluate the network with our data, for which there are few labeled images. Using this framework, we conduct a comparison between FSL models, parameter selection, training strategy, etc. We also discuss a weakness of the current FSL framework – lack of the option “does not belong to any of the classes”. This is a common problem in real-world systems due to false positives when detecting bounding boxes that contain, in our case, animals. We then propose an additional constraint to overcome this weakness.
更多查看译文
关键词
few shot,species,classification,real-world,in-the-wild,camera-trap
AI 理解论文
溯源树
样例
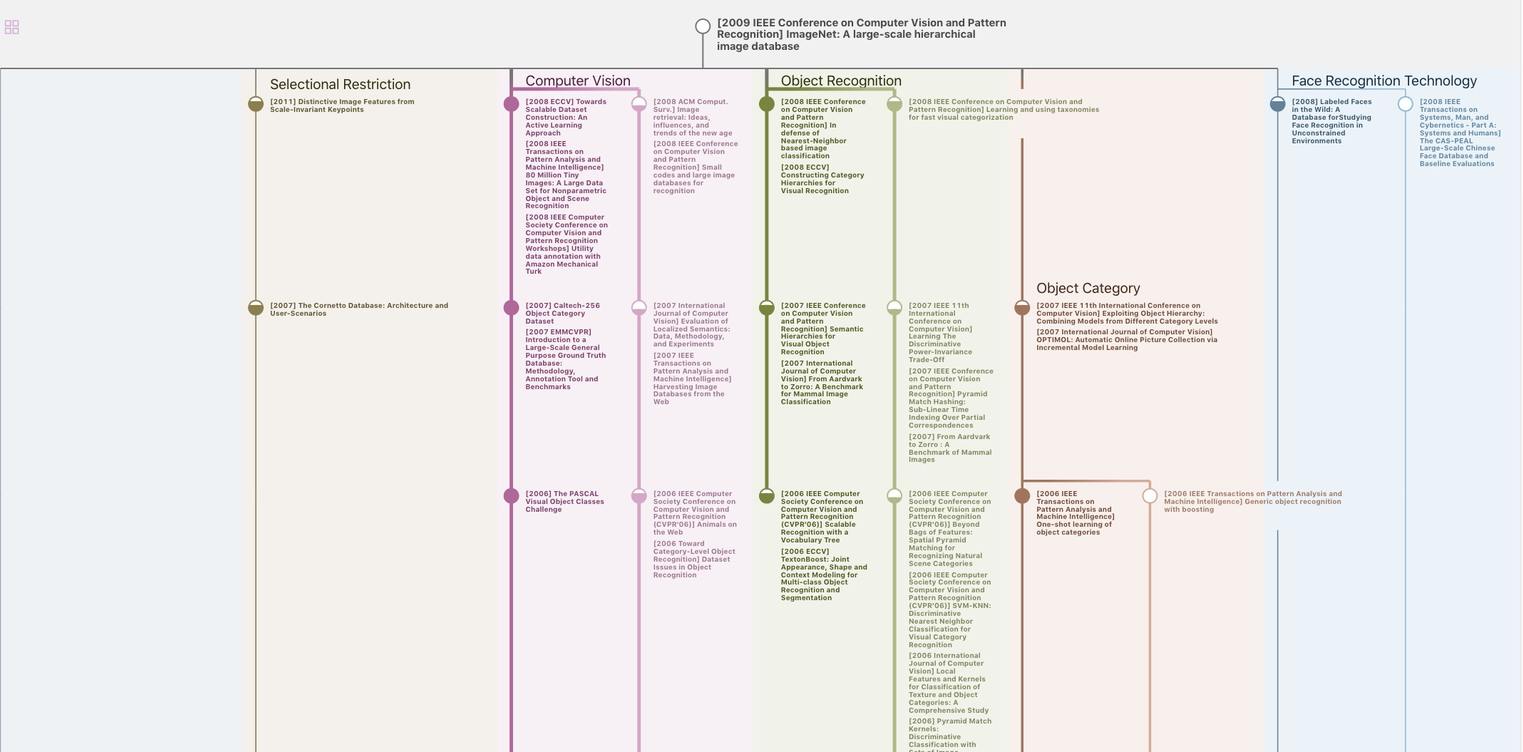
生成溯源树,研究论文发展脉络
Chat Paper
正在生成论文摘要