SmartMoE: Efficiently Training Sparsely-Activated Models through Combining Offline and Online Parallelization.
USENIX Annual Technical Conference(2023)
摘要
Deep neural networks are growing large for stronger model ability, consuming enormous computation resources to train them. Sparsely activated models have been increasingly proposed and deployed to reduce training costs while enlarging model size. Unfortunately, previous auto-parallelization approaches designed for dense neural networks can hardly be applied to these sparse models, as sparse models are datasensitive and barely considered by prior works. To address these challenges, we propose SMARTMOE to perform distributed training for sparsely activated models automatically. We find optimization opportunities in an enlarged space of hybrid parallelism, considering the workload of data-sensitive models. The space is decomposed into static pools offline, and choices to pick within a pool online. To construct an optimal pool ahead of training, we introduce a data-sensitive predicting method for performance modeling. Dynamic runtime selection of optimal parallel strategy is enabled by our efficient searching algorithm. We evaluate SMARTMOE on three platforms with up to 64 GPUs. It achieves up to 1.88x speedup in end-to-end training over the state-of-the-art MoE model training system FasterMoE.
更多查看译文
AI 理解论文
溯源树
样例
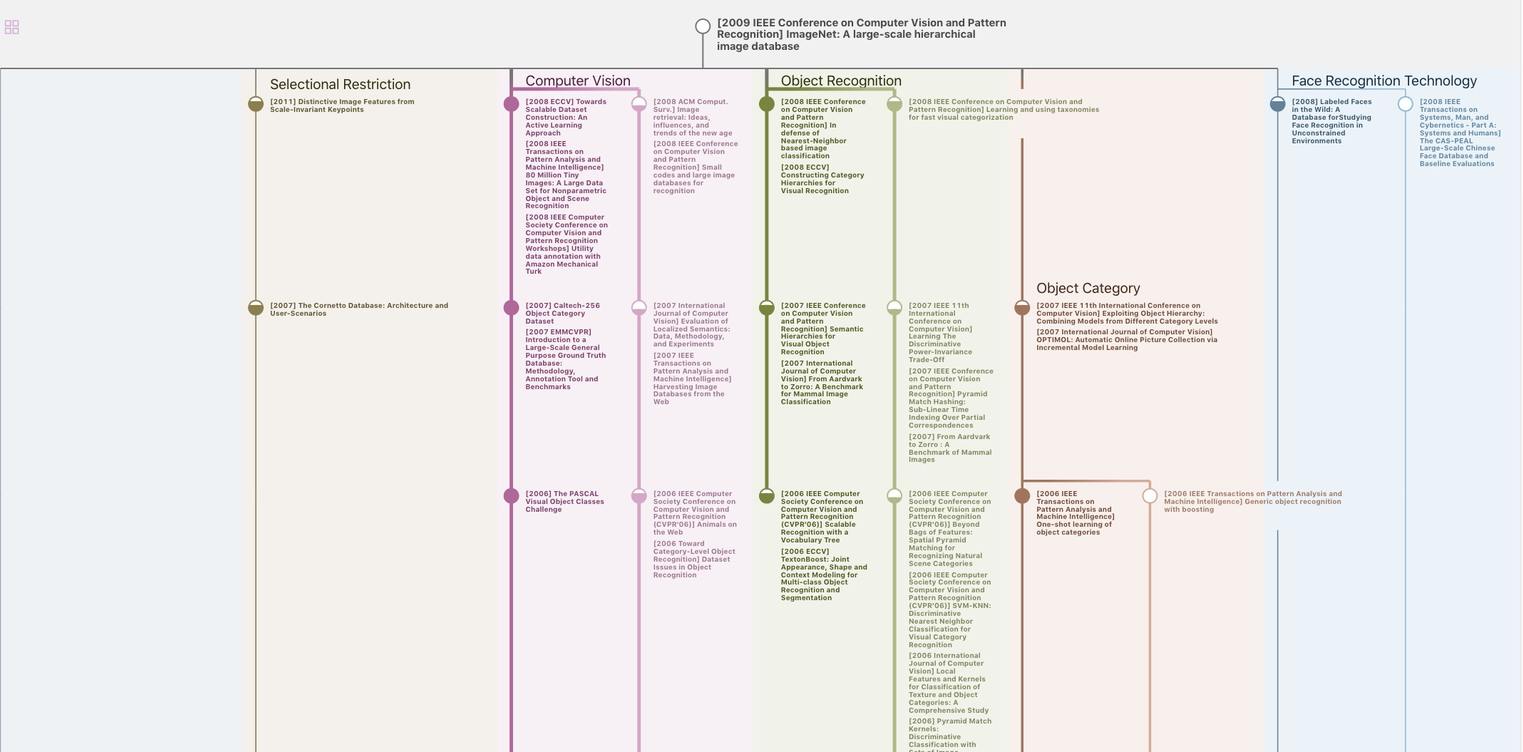
生成溯源树,研究论文发展脉络
Chat Paper
正在生成论文摘要