Data-driven heuristic symbolic models and application to limit-cycle detection
2023 AMERICAN CONTROL CONFERENCE, ACC(2023)
摘要
Symbolic control allows to provide formal guarantees for generic optimal control problems on nonlinear systems. It relies on the construction of a finite abstraction of the system which requires the discretization of the state space. Therefore, these methods suffer from the curse of dimensionality and a critical step is the choice of the state space partition. In this paper, we propose a data-driven heuristic abstraction approach relying on a probabilistic interpretation of the discretization error. Our approach can be used to automatically compare different partitions of the state space and to infer complex properties about the original system. As a proof of concept, we use our approach for the detection of limit cycles.
更多查看译文
AI 理解论文
溯源树
样例
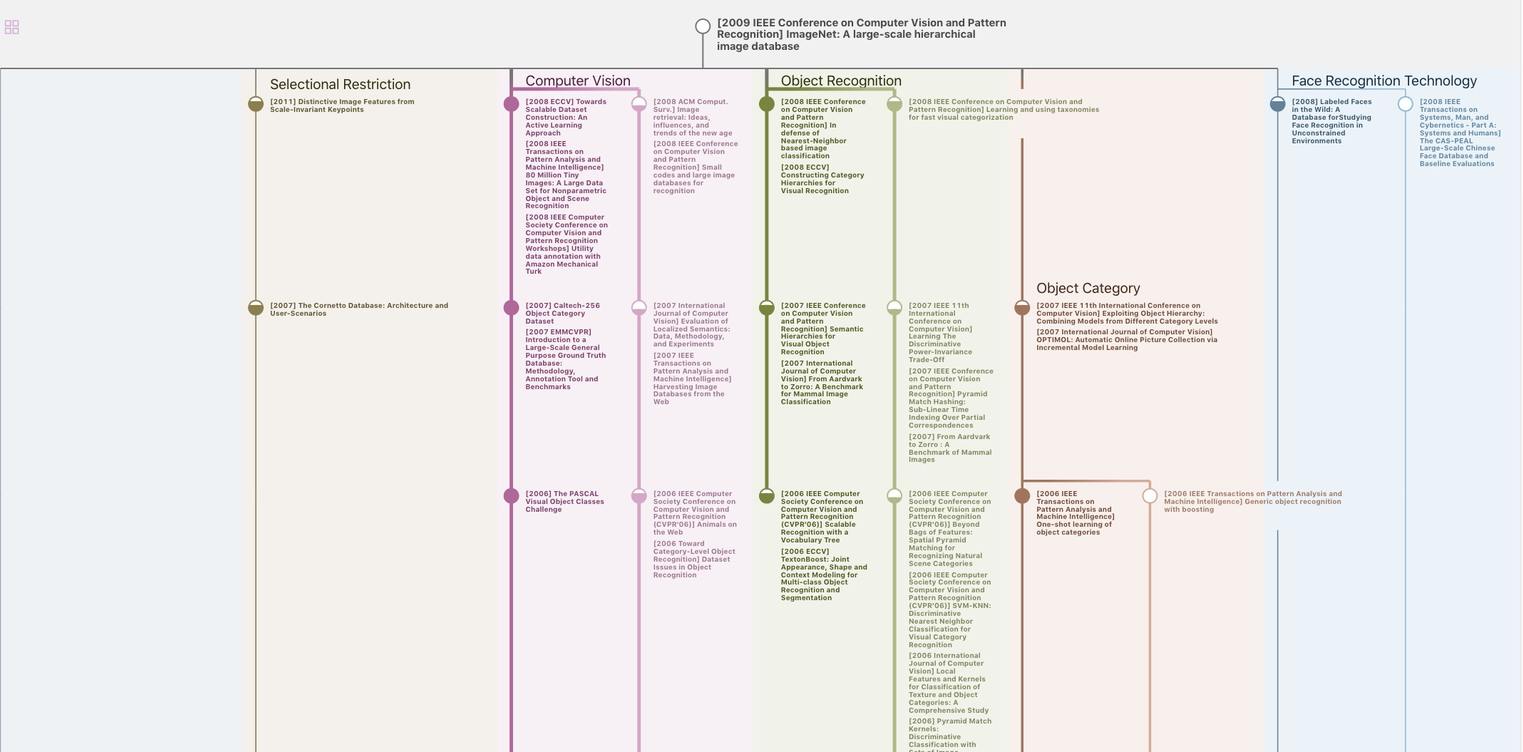
生成溯源树,研究论文发展脉络
Chat Paper
正在生成论文摘要