Continual Observation under User-level Differential Privacy.
SP(2023)
摘要
In the foundational work of Dwork et al. [15] on continual observation under differential privacy (DP), two privacy models have been proposed: event-level DP and user-level DP. The latter provides a much stronger notion of privacy, as it allows a user to contribute an arbitrary number of items. Under event-level DP, their mechanisms match the optimal utility bounds in the static setting up to polylogarithmic factors for all union-preserving functions. Unfortunately, in contrast to this strong result for event-level DP, their user-level DP mechanisms have weak utility guarantees and many restrictions on the data. In this paper, we take an instance-specific approach, designing continual observation mechanisms for a number of fundamental functions under user-level DP. Our mechanisms do not need any a priori restrictions on the data, while providing utility guarantees that degrade gracefully as the hardness of the data increases. For the count and sum function, our mechanisms are down-neighborhood optimal, matching the static setting up to polylogarithmic factors. For other functions, they do not match the static case, but we prove that this is inevitable, which is the first separation result for continual observation under differential privacy.
更多查看译文
关键词
Differential privacy,Continual observation,User-level differential privacy
AI 理解论文
溯源树
样例
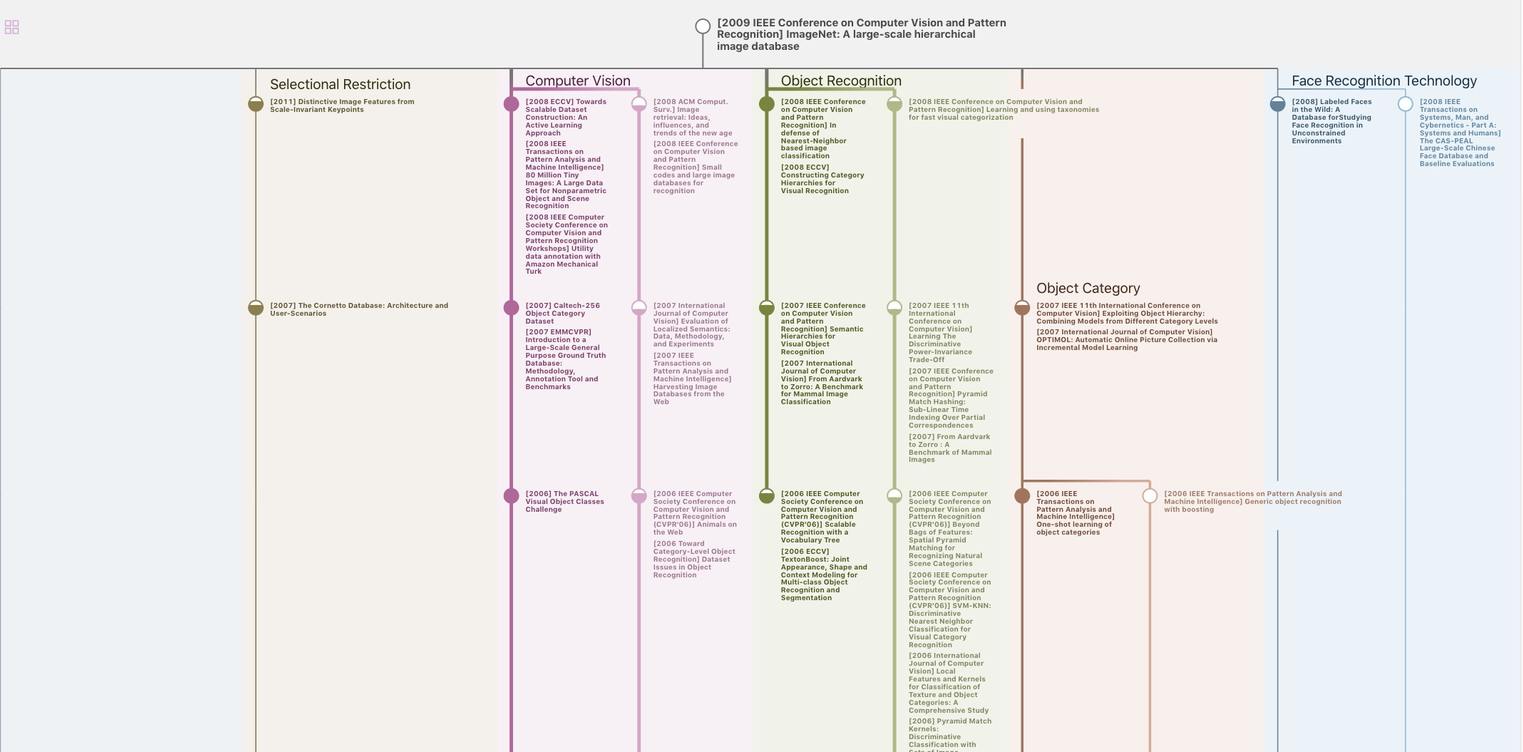
生成溯源树,研究论文发展脉络
Chat Paper
正在生成论文摘要